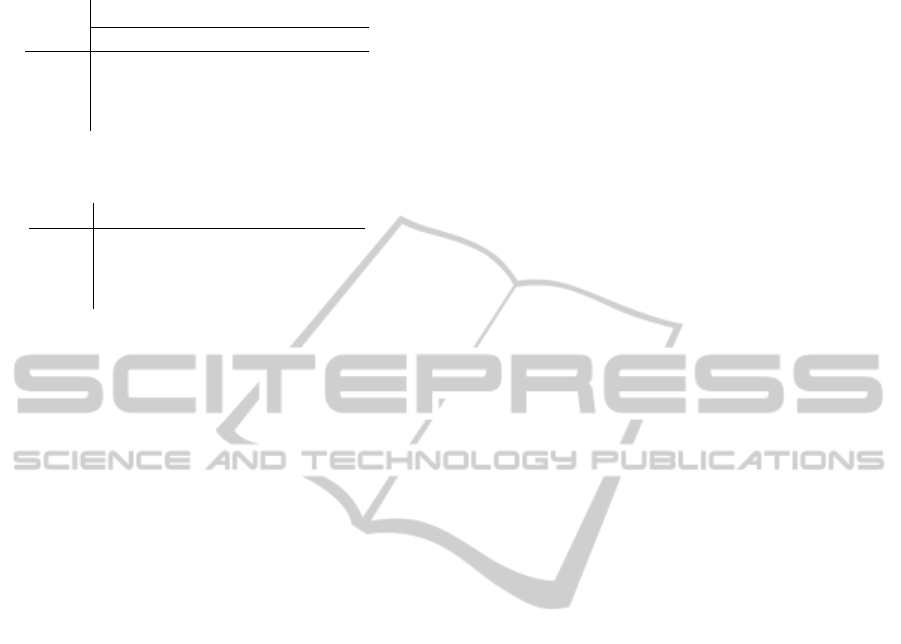
Table 2: Relative (%) Errors for varying capacities.
∆T
Capacity, Q
10 20 40 60 80
1.0 6.67 3.15 1.68 0.91 0.55
0.5 1.83 0.64 0.48 0.51 0.51
0.25 1.58 1.17 1.46 1.46 1.46
0.125 1.65 0.87 0.58 0.58 0.58
Table 3: Computation Times for the ‘joint’ method.
∆T Comput. Times Relative Comput. Times
1.0 45.5s 46.7%
0.5 56.8s 17.5%
0.25 71.6s 11.5%
0.125 102.4s 7.7%
as ∆T → 0. To justify this further the reader is re-
ferred to figure 2 where it shows that the price rate
functions used in the two methods would converge
as ∆T → 0. Moreover, the maximum relative error
decreases with capacity. This observation has more
to do with the nature of the carpark rather than the
choice of the method, as relatively big carparks might
require little or even no optimization at all, which im-
plies that the role of any optimal policy is reduced and
thus both methods would be even closer to each other.
Lastly, table 3 presents the computation times needed
for the ‘joint’ method to calculate the full set of opti-
mal revenues (the entire matrix for all capacities and
times) along with a relative speed comparison against
the MC approach. Our results show that the reduction
in computation time is significant; in particular, for
∆T = 1, the ‘joint’ algorithm takes only 46% of the
time it would take the MC approach to calculate the
optimal solution. When ∆T = 0.125 the statistics are
even more impressive as it only needs less than 8% of
the original time. This monotonic increase in speed
is explained by the fact that the time the PDE takes
to calculate the optimal policy is independent of the
size of ∆T, while in the MC the computation times
increase linearly.
4 CONCLUSIONS
In this paper, we have worked with the continuous-
time PDE model and MC model proposed in (Pa-
payiannis et al., 2012). Since the PDE could only
solve for the rate at which cash is generated through
an infinitesimal time we reformulated it in such a way
that it solves for the cash that is generated within a
time period of finite size ∆T and matched that against
the MC. The results validated our expectations as the
optimal values and optimal rejection policies where
remarkably close to one another. Moreover, the opti-
mal surfaces from the reformulated PDE where much
smoother and the computation times have improved
significantly. However, as the PDE could still not
solve the ‘exact’ problem we have proposed a ‘joint’
method that calculates the optimal policy from the
PDE and it then employs this to make optimal deci-
sions by using the MC. This method performed ex-
ceptionally well as it produced revenues around 1%
of the actual ones at most cases. For carparks that op-
erate with slots that can be booked for a minimum of
3 hours (∆T = 0.125) the improvement in computa-
tional speed was more than 92% (it only took 7.7% of
the original time) which it renders it ideal for being
used in a web reservation engine; for even smaller ∆T
the speed improvement is expected to be greater.
REFERENCES
Arnott, R. and Rowse, J. (1999). Modeling parking. Journal
of Urban Economics, 45(1):97 – 124.
Bitran, G. and Caldentey, R. (2003). An overview of pricing
models for revenue management. Manufacturing and
Service Operations Management, 5(3):203 – 229.
Gallego, G. and van Ryzin, G. (1994). Optimal dynamic
pricing of inventories with stochastic demand over fi-
nite horizons. Management Science, 40(8):pp. 999–
1020.
Onieva, L., Mu˜nuzuri, J., Guadix, J., and Cortes, P. (2011).
An overview of revenue management in service indus-
tries: an application to car parks. The Service Indus-
tries Journal, 31(1):pp.91–105.
Papayiannis, A., Johnson, P., Yumashev, D., Howell, S.,
Proudlove, N., and Duck, P. (2012). Continuous-
time revenue management in carparks. In Proceed-
ings of the 1st International Conference on Opera-
tions Research and Enterprise Systems, pages 73–82.
ICORES, SciTePress Digital Library.
Talluri, K. and van Ryzin, G. (2004). The Theory and Prac-
tice of Revenue Management. Kluwer Academic Pub-
lishers, Boston/Dordrecht/London.
Teodorovi´c, D. and Luˇci´c, P. (2006). Intelligent parking
systems. European Journal of Operational Research,
175(3):1666 – 1681.
Teodorovi´c, D. and Vukadinovi´c, K. (1998). Traffic con-
trol and Trasport Planning: A Fuzzy Sets and Neural
Netweorks Approach. Kluwer Academic Publishers,
Boston.
Young, W., Thompson, R. G., and Taylor, M. A. (1991). A
review of urban car parking models. Transport Re-
views, 11(1):63–84.
Zhao, Y., Triantis, K., Teodorovic, D., and Edara, P. (2010).
A travel demand management strategy: The down-
town space reservation system. European Journal of
Operational Research, 205(3):584 – 594.
Continuous-timeRevenueManagementinCarparks-PartTwo:RefiningthePDE
279