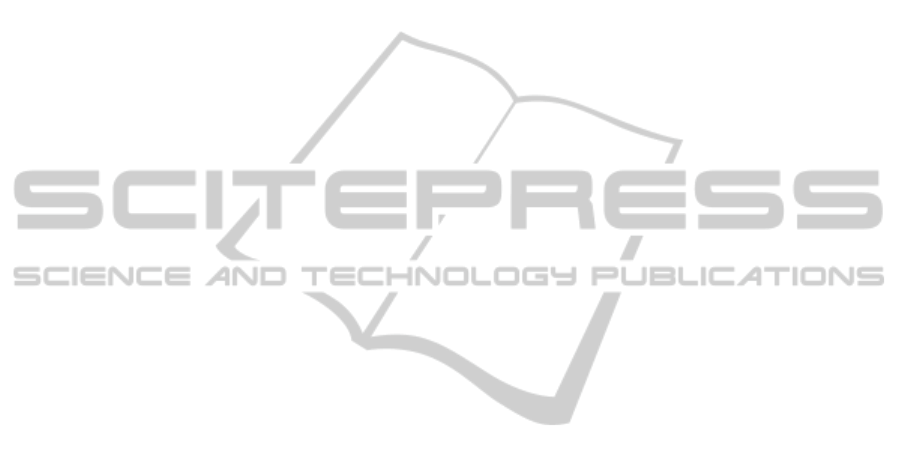
5 CONCLUSIONS
The extractions of the finger pulse waves using the
largest Lyapunov exponents and the sample entropy
values have shown to be a better combination of the
biosignal features than the coupling of the largest Lya-
punov exponents and autonomic nerve balance val-
ues. The improvement suggests the usefulness of
chaos and nonlinear dynamical analysis of the pho-
toplethysmography waveforms for depression detec-
tion, which can be useful for mental health care.
ACKNOWLEDGEMENTS
This work was supported by the FY 2012 University
of Aizu Competitive Research Funds for Revitaliza-
tion Category.
REFERENCES
Allen J., Photoplethysmography and its application in clin-
ical physiological measurement, Physiol. Meas., 28
(2007) R1-R39.
Abarbanel H.D.I., Analysis of Observed Chaotic Data. New
York: Springer-Verlag, 1996.
Asada HH, et al., Mobile monitoring with wearable pho-
toplethysmographic biosensors, IEEE EMB Magazine
22 (2003) 28-40.
Chen Y.T., I.C. Hung, M.W. Huang, C.J. Hou, K.S. Cheng,
Physiological signal analysis for patients with depres-
sion, BMEI 2011, pp. 805-808, 2011.
Cohn J.F., et al., Detecting depression from facial actions
and vocal prosody, 3rd Int. Conf. ACII, pp. 1-7, 2009.
Dingwell J.B., Lyapunov exponents. Wiley Encyclopedia of
Biomedical Engineering, John Wiley & Sons, pp. 1-
12, 2006.
Higa M., Autonomic nervous balance computation appara-
tus and method therefor, US Patent US 2011/0313303
A1, 2011.
Hu YH, W. Wang, T. Suzuki, M. Oyama-Higa, Character-
istic extraction of mental disease patients by nonlin-
ear analysis of plethysmograms, CMLS-11, AIP Conf.
Proc 1371, pp. 92-101, 2011.
Kemp A. H., et al., Depression, comorbid anxiety disor-
ders, and heart rate variability in physically healthy,
non-medicated patients: implications for cardiovascu-
lar risk, PLoS ONE, 7 (2012) e30777.
Low L. S., N. C. Maddage, M. Lech, L. B. Sheeber, N. B.
Allen, Detection of clinical depression in adolescents’
speech during family interactions, IEEE Trans Biomed
Engineering, 58 (2011) 574-586.
Marcus M., H. Westra, M. Vermani, M. A. Katzman, Pa-
tient predictors of detection of depression and anxiety
disorders in primary care, J. Participat Med., 3 (2011)
e15.
Pincus S. M., Approximate entropy as a measure of system
complexity. Proc. Natl. Acad. Sci. U.S.A., 88 (1991)
2297-2301.
Richman J. S., J. R. Moorman, Physiological time-series
analysis using approximate entropy and sample en-
tropy. Amer. J. Physiol. Heart Circ. Physiol., 278
(2000) H2039-H2049.
Rosenstein M. T., et al., A practical method for calculat-
ing largest Lyapunov exponents from small data sets,
Physica D: Nonlinear Phenomena, 65 (1993) 117-134.
Russoniello C. V., et al., A measurement of electrocardio-
graphy and photoplethesmography in obese children,
Appl Psychophysiol Biofeedback, 35 (2010) 257-259.
Soennesyn H., et al., White matter hyperintensitives and the
course of depressive symptoms in elderly people with
mild dementia, Dement Geriatr Cogn Disord Extra, 2
(2012) 97-111.
Sprott J. L., Chaos and Time-Series Analysis. Oxford, New
York, 2003.
Takens F., Detecting strange attractors in turbulence. In:
D. Rand and L.S. Young, eds., Dynamical Systems
and Turbulence, Lecture Notes in Mathematics, 898
(1981) 366-381.
Waza K., A. V. Grahama, S. J. Zyzanskia, K. Inoue, Com-
parison of symptoms in Japanese and American de-
pressed primary care patients, Family Practice, 16
(1999) 528-533.
Williams G. P., Chaos Theory Tamed. Joseph Henry Press,
Washington D.C., 1997.
Wilson L., Autonomic nervous system health, Arizona Net-
working News, http://drlwilson.com/Articles/
NERVOUS%20SYSTEM.htm (2005).
ChaosandNonlinearTime-seriesAnalysisofFingerPulseWavesforDepressionDetection
301