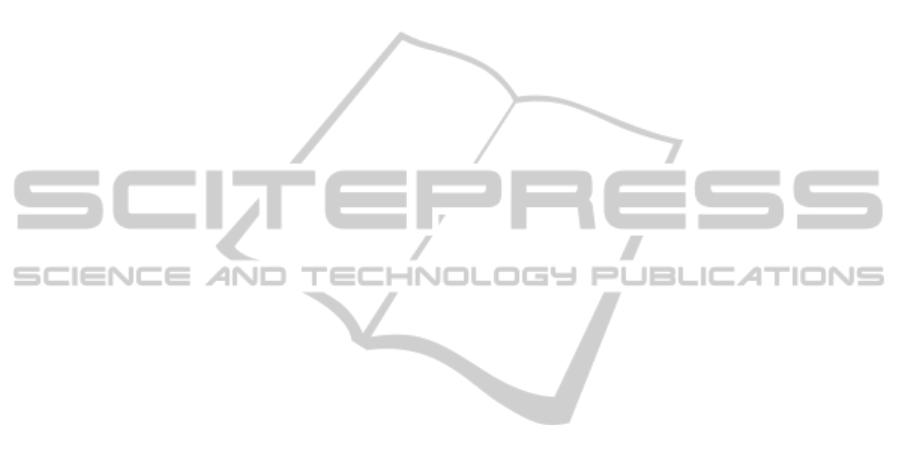
portant to focus the number of pulses in each one of
the clusters. Cluster 1 presents only 458 pulses, a re-
duced value in comparison with pulses in cluster 2
and 3, 1550 and 2463, respectively. However, this
fact occurs due to the population characteristics, only
young subjects between 18 and 30 years. Clustering
methodologies can be further improved by perform-
ing a progressive attribute removal for a subsequent
visual correction.
The developed methodology has proven its usage
in screening studies due to its descriptive and predic-
tive power. The successful application of data mining
techniques can help to predict under-diagnosed pa-
tients, and identify and classify at-risk people in terms
of health with the goal of reducing healthcare cost.
ACKNOWLEDGEMENTS
Authors acknowledge Fundação para a Ciência e
Tecnologia for funding SFRH/BD/61356/2009 and
PTDC/SAU-BEB/100650/2008, project developed
under the initiative of QREN, funding by UE/FEDER,
through COMPETE-Programa Operacional Factores
de Competitividade. The authors also thanks to Coim-
bra University Hospital Centre (C.H.U.C.) and Dr.
Rui Providência, SCDSOS-Sudden Cardiac Death
Screening of Risk factors, for the support in clinical
trials.
REFERENCES
Almeida, V. G., Pereira, H. C., Pereira, T., Figueiras, E.,
Borges, E., Cardoso, J. M. R., and Correia, C. (2011a).
Piezoelectric probe for pressure waveform estimation
in flexible tubes and its application to the cardiovas-
cular system. Sensors and Actuators A: Physical,
169(1):217–226.
Almeida, V. G., Pereira, T., Borges, E., Pereira, H. C., Car-
doso, J. M. R., and Correia, C. (2011b). A real time
cardiac monitoring system-arterial pressure waveform
capture and analysis. Proceedings of the PECCS 2011.
Algarve, Portugal.
Almeida, V. G., Santos, P., Figueiras, E., Borges, E.,
Pereira, T., Pereira, H. C., Cardoso, J. M. R., and
Correia, C. (2011c). Hemodynamic features extrac-
tion from a new arterial pressure waveform probe.
Proceedings of the BIOSTEC (BIOSIGNALS 2011).
Rome, Italy.
Avolio, A. P., Butlin, M., and Walsh, A. (2010). Ar-
terial blood pressure measurement and pulse wave
analysis–their role in enhancing cardiovascular as-
sessment. Physiol Meas, 31(1):R1–47. Avolio, Al-
berto P Butlin, Mark Walsh, Andrew England Physiol
Meas. 2010 Jan;31(1):R1-47. Epub 2009 Nov 26.
Bortel, L. M. V., Duprez, D., Starmans-Kool, M. J., Safar,
M. E., Giannattasio, C., Cockcroft, J., Kaiser, D. R.,
and Thuillez, C. (2002). Clinical applications of arte-
rial stiffness, task force iii: Recommendations for user
procedures. AJH, 15:445–452.
Breiman, L. (2001). Random forests. Machine Learning,
45:5–32.
Clemente, F., Arpaia, P., and Cimmino, P. (2010).
A piezo-film-based measurement system for
global haemodynamic assessment. Physiol Meas,
31(5):697–714. Clemente, Fabrizio Arpaia, Pasquale
Cimmino, Pasquale England Physiol Meas. 2010
May;31(5):697-714. Epub 2010 Apr 16.
Gorunescu, F., Gorunescu, M., Saftoiu, A., Vilmann, P., and
Belciug, S. (2011). Competitive/collaborative neural
computing system for medical diagnosis in pancreatic
cancer detection. Expert Systems, 28(1):33–48.
Han, J. and Kamber, M. (2006). Data Mining:Concept and
Techniques. Elsevier, San Francisco.
Haykin, S. (1998). Neural Networks - A Comprehensive
Foundation. Pearson education, India.
Jovic, A. and Bogunovic, N. (2011). Electrocardio-
gram analysis using a combination of statistical, ge-
ometric, and nonlinear heart rate variability features.
Artif Intell Med, 51(3):175–86. Jovic, Alan Bo-
gunovic, Nikola Netherlands Artif Intell Med. 2011
Mar;51(3):175-86. Epub 2010 Oct 25.
Kotsiantis, S. B. (2007). Supervised machine learning:
A review of classification techniques. Informatica,
31:249–268.
Laurent, S., e. a. (2006). Expert consensus document on ar-
terial stiffness:methodological issues and clinical ap-
plications. European Heart Journal, 27:2588–2605.
Mendis, S. e. a. (2011). Global Atlas on Cardiovascular
Disease Prevention and Control. World Health Orga-
nization, Geneva.
Quinlan, J. R. (1993). C4.5: Programs for Machine Learn-
ing. Morgan Kaufmann Publishers, San Mateo, Cali-
fornia.
Shah, A. S., Dolan, L. M., Gao, Z., Kimball, T. R., and
Urbina, E. M. (2011). Clustering of risk factors: a
simple method of detecting cardiovascular disease in
youth. Pediatrics, 127(2):e312–8.
Tsipouras, M. G., ThemisP.Exarchos, Fotiadis, D. I., Kot-
sia, A. P., Vakalis, K. V., Naka, K. K., and Michalis,
L. K. (2008). Automated diagnosis of coronary
artery disease based on data mining and fuzzy mod-
eling. IEEE Transactions On Information Technology
In Biomedicine.
WEKA (2012). Weka 3: Data mining software in java.
Yang, J., Singh, H., Hines, E. L., Schlaghecken, F., Iliescu,
D. D., Leeson, M. S., and Stocks, N. G. (2012). Chan-
nel selection and classification of electroencephalo-
gram signals: An artificial neural network and ge-
netic algorithm-based approach. Artif Intell Med,
55(2):117–26. Yang, Jianhua Singh, Harsimrat Hines,
Evor L Schlaghecken, Friederike Iliescu, Daciana D
Leeson, Mark S Stocks, Nigel G Netherlands Artif In-
tell Med. 2012 Jun;55(2):117-26. Epub 2012 Apr 12.
DataMiningbasedMethodologiesforCardiacRiskPatternsIdentification
133