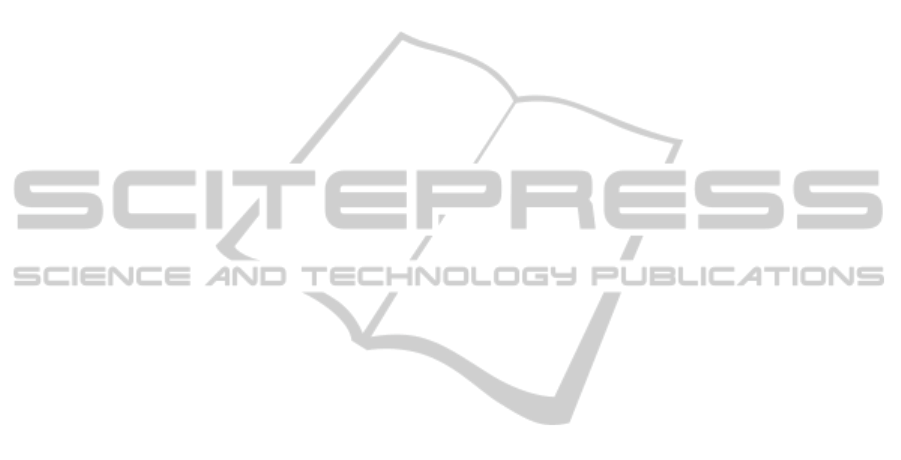
5 RESULTS AND CONCLUSIONS
In the image presented in Fig.2(a), the aim is to
smooth the noise present in the images while preserv-
ing both the white and the grey matters. We used
our detector with µ = 5, λ = 1.5 and ∆θ = 5
◦
for re-
gions classification. The threshold for the edge/region
classifier s
th
is equal to 0.002. Parameters used in
anisotropic edge detector in order to compute (θ
1
,θ
2
)
are µ = 5, λ = 1 and ∆θ = 2
◦
. The results of our
anisotropic diffusion are presented in the Fig. 2(l).
Note that the limit between the grey and the white
matter is perfectly visible, Fig. 2(t) illustrates the
sharpness of this edge.
We compare our result with several approaches
as well as the well known median, Nagao (Nagao
and Matsuyama, 1979), Kuwahara (Kuwahara et al.,
1976) and bilateral filters (Tomasi and Manduchi,
1998). For these different methods, the noise is not
completely removed and grey matter edges are not
preserved. Tensorial approaches bring either a fiber
effect to the image (Weickert, 1999) or grey matter is
blurred (Tschumperl
´
e, 2006), as for the approach of
(Alvarez et al., 1992).
In order to show the efficiency of our method for
noise removal and edge of grey/white matter contours
enhancement, we show the image surface of our re-
sults. The 3D elevation of our result allows to see
that grey/white matter edges are well preserved and
sharped. Figs. 2 (m), (n), (o) and (p) show the
isophotes (curves of the image surface of constant in-
tensity) according to 15 levels. It is visible that our
approach preserves also small objects which could be
a tumor or a default inside the brain.
We have proposed in this paper a method for re-
moving noise preserving white/grey matters edges in
MRI images by pixel classification using a rotating
smoothing filter followed by a PDE. Our classifica-
tion method seems very promising as we have been
able to classify correctly white/grey matters edges.
Anisotropic diffusion in two directions provided by
an edge detector using half smoothing kernels keeps
edges and corners of different objects. Comparing our
results with existing algorithms allows us to validate
our method. Next on our agenda is to develop an in-
ternet platform where users could experiment restora-
tion with their own images and apply a segmentation
on the result.
REFERENCES
Alvarez, L., Lions, P.-L., and Morel, J.-M. (1992). Image
selective smoothing and edge detection by nonlinear
diffusion, ii. SIAM J. of Num. Anal., 29(3):845–866.
Aubert, G. and Kornprobst, P. (2006). Mathematical prob-
lems in image processing: partial differential equa-
tions and the calculus of variations (second edition),
volume 147. Springer-Verlag.
Canny, F. (1986). A computational approach to edge detec-
tion. IEEE TPAMI, 8(6):679–698.
Catt
´
e, F., Dibos, F., and Koepfler, G. (1995). A morpholog-
ical scheme for mean curvature motion and applica-
tions to anisotropic diffusion and motion of level sets.
SIAM J. Numer. Anal., 32:1895–1909.
Deriche, R. (1992). Recursively implementing the gaussian
and its derivatives. In ICIP, pages 263–267.
Freeman, W. T. and Adelson, E. H. (1991). The design and
use of steerable filters. IEEE TPAMI, 13:891–906.
Jacob, M. and Unser, M. (2004). Design of steerable filters
for feature detection using canny-like criteria. IEEE
TPAMI, 26(8):1007–1019.
Klauschen, F., Goldman, A., Barra, V., Meyer-Lindenberg,
A., and Lundervold, A. (2009). Evaluation of auto-
mated brain mr image segmentation and volumetry
methods. Human brain mapping, 30(4):1310–1327.
Kuwahara, M., Hachimura, K., Eiho, S., and Kinoshita, M.
(1976). Processing of ri-angiocardiographic images.
Digital Processing of Biomedical Images, pages 187–
203.
Magnier, B., Montesinos, P., and Diep, D. (2011a). Fast
Anisotropic Edge Detection Using Gamma Correction
in Color Images. In IEEE 7th ISPA, pages 212–217.
Magnier, B., Montesinos, P., and Diep, D. (2011b). Texture
Removal in Color Images by Anisotropic Diffusion.
In VISAPP, pages 40–50.
Magnier, B., Montesinos, P., and Diep, D. (2012). A new
region-based pde for perceptual image restoration. In
VISAPP, pages 56–65.
Montesinos, P. and Magnier, B. (2010). A New Perceptual
Edge Detector in Color Images. In ACIVS, volume 2,
pages 209–220.
Nagao, M. and Matsuyama, T. (1979). Edge preserving
smoothing. CGIP, 9:394–407.
Perona, P. (1992). Steerable-scalable kernels for edge detec-
tion and junction analysis. IMAVIS, 10(10):663–672.
Perona, P. and Malik, J. (1990). Scale-space and edge
detection using anisotropic diffusion. IEEE TPAMI,
12:629–639.
Poynton, C. (1996). A technical introduction to digital
video. John Wiley & Sons, Inc. New York, NY, USA.
Simoncelli, E. and Farid, H. (1996). Steerable wedge filters
for local orientation analysis. IEEE TIP, 5(9):1377–
1382.
Tomasi, C. and Manduchi, R. (1998). Bilateral filtering for
gray and color images. In International Conference
on Computer Vision, pages 839–846. IEEE.
Tschumperl
´
e, D. (2006). Fast anisotropic smoothing
of multi-valued images using curvature-preserving
PDE’s. IJCV, 68(1):65–82.
Weickert, J. (1999). Coherence-enhancing diffusion filter-
ing. IJCV, 31(2):111–127.
AToolforBrainMagneticResonanceImageSegmentation
79