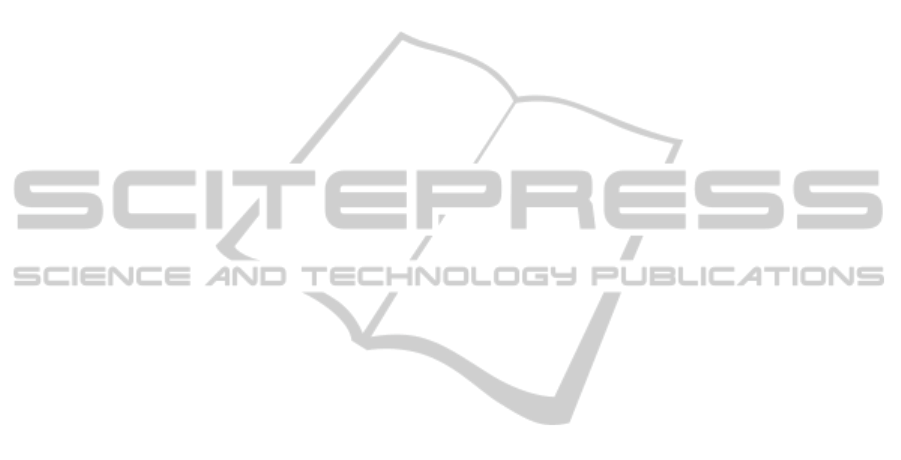
and habits emergence), agents are able to explore new
organisation and to discover pattern of actions in rela-
tion with time constraints and energy price for saving
purpose. In addition to this evaluation, our simulator
is provided with a participatory-simulation user inter-
face (introduced in (Haradji et al., 2012) ) that allow
to give control of one or several agents to users. Stu-
dents in our lab “played their own role” in our test
scenarios, which allowed us to validate the believabil-
ity of the model. In most situations, students could
not distinguish between artificial agents and human-
controlled ones.
We are currently extending the SMACH model to
study the activity of groups of families in different en-
vironments, over long period of time (one year) and
taking into account external temperature and build-
ing’s thermodynamical properties. This lead us to re-
consider the action rhythm model and to use multi-
level agent systems for individuals, families and ac-
tivities. Our long-term goal is to allow energy com-
panies to be able to investigate incentive to reduce or
to have a better prediction of consumption peaks us-
ing simulation of human activity.
REFERENCES
Duy Ha, L., Ploix, S., Zama
¨
ı, E., and Jacomino, M. (2006).
A home automation system to improve household en-
ergy control. In Proceedings of The 12th IFAC Sym-
posium INCOM2006.
European Environment Agency (2008). Energy and envi-
ronment report. Technical Report 6.
FIPA consortium (2003). FIPA Communicative Act Library
Specification and FIPA ACL Message Structure Spec-
ification. Technical report.
Freire, R. Z., Oliveira, G. H., and Mendes, N. (2008). Pre-
dictive controllers for thermal comfort optimization
and energy savings. Energy and Buildings, 40(7):1353
– 1365.
Gil-Quijano, J. and Sabouret, N. (2010). Prediction of hu-
mans’ activity for learning the behaviors of electrical
appliances in an intelligent ambiant environment. In
Proc. of the IEEE/WIC/ACM IAT’10 int. conf., pages
283–286.
Haradji, Y., Poizat, G., and Semp
´
e, F. (2012). Human Activ-
ity and Social Simulation, pages 416–425. CRC Press.
Kashif, A., Ploix, S., Dugdale, J., and Le, X. H. B. (2012).
Simulating the dynamics of occupant behaviour for
power management in residential buildings. Energy
and Buildings, To appear.
Mahdavi, A. and Pr
¨
oglh
¨
of, C. (2009). Toward empirically-
based models of people’s presence and actions in
buildings. In Proceedings of Building Simulation 09,
pages 537–544.
Massoud, A. S. and Wollenberg, B. (2005). Toward a smart
grid: power delivery for the 21st century. Power and
Energy Magazine, IEEE, 3(5):34–41.
Newsham, G. R. and Bowker, B. G. (2010). The effect of
utility time-varying pricing and load control strategies
on residential summer peak electricity use: A review.
Energy Policy, 38(7):3289 – 3296.
North, M., Howe, T., Collier, N., and Vos, J. (2007). A
declarative model assembly infrastructure for verifica-
tion and validation. In Advancing Social Simulation:
The First World Congress, pages 129–140. Springer
Japan.
Poquet, G. and Dujin, A. (2008). Pour les m
´
enages, la
recherche du confort prime encore sur les
´
economies
d’
´
energie. Consommation & Modes de Vie (CRE-
DOC), 210.
Rogers, A., Maleki, S., Ghosh, S., and Nicholas R, J.
(2011). Adaptive home heating control through gaus-
sian process prediction and mathematical program-
ming. In ATES’10, pages 71–78.
Searle, J. (1969). Speech Acts. Cambridge University Press.
Semp
´
e, F. and Gil-Quijano, J. (2010). Incremental and sit-
uated modeling for multi-agent based simulations. In
Proc. of the IEEE RIVF’10 int. conf., pages 1–6.
Sierhuis, M., Clancey, W. J., van Hoof, R., and de Hoog, R.
(2000). Modeling and simulating human activity. In-
telligent Agents for Computer Supported Co-operative
Work Workshop.
Taillandier, P., Vo, D.-A., Amouroux, E., and Drogoul, A.
(2010). Gama: A simulation platform that integrates
geographical information data, agent-based modeling
and multi-scale control. In PRIMA’10, pages 242–
258.
Wid
´
en, J. and W
¨
ackelgard, E. (2010). A high-resolution
stochastic model of domestic activity patterns and
electricity demand. Applied Energy, 87(6):1880–
1892.
Wilensky, U. and Evanston, I. (1999). Netlogo. center
for connected learning and computer based modeling.
Technical report, Northwestern University.
Yamaguchi, Y., Fujimoto, T., and Shimoda, Y. (2011). Oc-
cupant Behavior Model For Households To Estimate
High-temporal Resolution Residential Electricity De-
mand Profile. In Proceedings of BS2011, pages 1548–
1555, Sydney, Australia.
ICAART2013-InternationalConferenceonAgentsandArtificialIntelligence
80