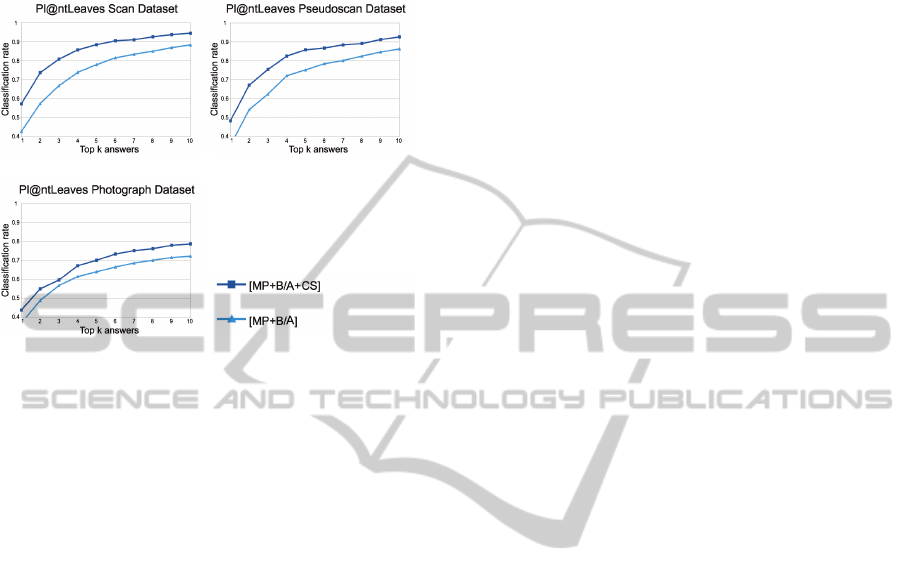
Database (Scan, Pseudoscan and Photograph) and
measured the presence of the true species among the
top k answers (k going from 1 to 10) returned by the
classification algorithm.
(a) (b)
(c)
Figure 6: Classification rates on the Pl@ntLeaves Database
on scan (a) pseudoscan (b) and photograph (c) images
Figure 6 presents the results obtained with
the global, base and apex shape descriptors only
[MP+B/A] and with the addition of the margin
descriptors [MP+B/A+CS], underlining the interest
of considering the contour in a very specific way,
with an average recognition score of 53%, and a
presence of the correct species in the 5 first answers
of 85%. The great improvement induced by the use
of curvature-based descriptors is here clearly visible.
The enhancement is less important on photographs
given the hardness of the task of retrieving the
exact leaf contour, which obviously deteriorates the
relevance of the contour description.
These scores may be compared to the results of
the 2011 ImageCLEF Plant Identification task (Go¨eau
et al., 2011a) concerning a very similar database, and
where the best participants reached an average score
of 45%.
6 CONCLUSIONS
The method presented in this article constitutes an
interesting step towards an explicative system of leaf
classification for an independent mobile application.
Being able to place high-level semantic concepts over
the values extracted from an image gives the user a
feedback that may prove of great usefulness for both
interactive and educational purposes.
A first implementation of the recognition process
on mobile devices is engaged with very satisfactory
results, but not yet with the nearly 150 native
European tree species we aim at recognizing. With
the number of potential classes increasing, it will
become a necessity to reduce the scope of the search,
by including geographical information linked to the
GPS system present in every smartphone. Knowing
in advance which species are likely to be found in
the geographical area where the user stands may be
a decisive step towards a truly reliable identification.
However, what we have now is a good base
structure for a new tree identification application,
designed to be functional in a natural environment,
and destined to anyone with interest in plants
but without the otherwise compulsory botanical
background.
REFERENCES
Belhumeur, P., Chen, D., Feiner, S., Jacobs, D., Kress,
W., Ling, H., Lopez, I., Ramamoorthi, R., Sheorey,
S., White, S., and Zhang, L. (2008). Searching the
world’s herbaria: A system for visual identication of
plant species. In ECCV.
Caballero, C. and Aranda, M. C. (2010). Plant
species identification using leaf image retrieval. In
Proceedings of the ACM International Conference on
Image and Video Retrieval, CIVR 2010, pages 327–
334.
Cerutti, G., Tougne, L., Mille, J., Vacavant, A., and Coquin,
D. (2011). Guiding active contours for tree leaf
segmentation and identification. In CLEF Working
Notes.
Coste, H. (1906). Flore descriptive et illustr´ee de la France
de la Corse et des contr´ees limitrophes.
Du, J. X., Wang, X. F., and Zhang, G.-J. (2007). Leaf shape
based plant species recognition. Applied Mathematics
and Computation, 185:883–893.
Go¨eau, H., Bonnet, P., Joly, A., Boujemaa, N., Barthelemy,
D., Molino, J.-F., Birnbaum, P., Mouysset, E., and
Picard, M. (2011a). The clef 2011 plant images
classification task. In CLEF Working Notes.
Go¨eau, H., Joly, A., Yahiaoui, I., Bonnet, P., and Mouysset,
E. (2011b). Participation of inria & pl@ntnet to
imageclef 2011 plant images classification task. In
CLEF Working Notes.
Im, C., Nishida, H., and Kunii, T. L. (1998). A hierarchical
method of recognizing plant species by leaf shapes. In
MVA, pages 158–161.
Mokhtarian, F. and Abbasi, S. (2004). Matching
shapes with self-intersections: Application to leaf
classification. IEEE IP, 13(5):653–661.
Mokhtarian, F. and Mackworth, A. (1992). A theory of
multiscale, curvature-based shape representation for
planar curves. IEEE PAMI, 14:789–805.
Pei, S. C. and Lin, C. N. (1992). The detection of
dominant points on digital curves by scale-space
filtering. Pattern Recognition, 25(11):1307–1314.
Curvature-Scale-basedContourUnderstandingforLeafMarginShapeRecognitionandSpeciesIdentification
283