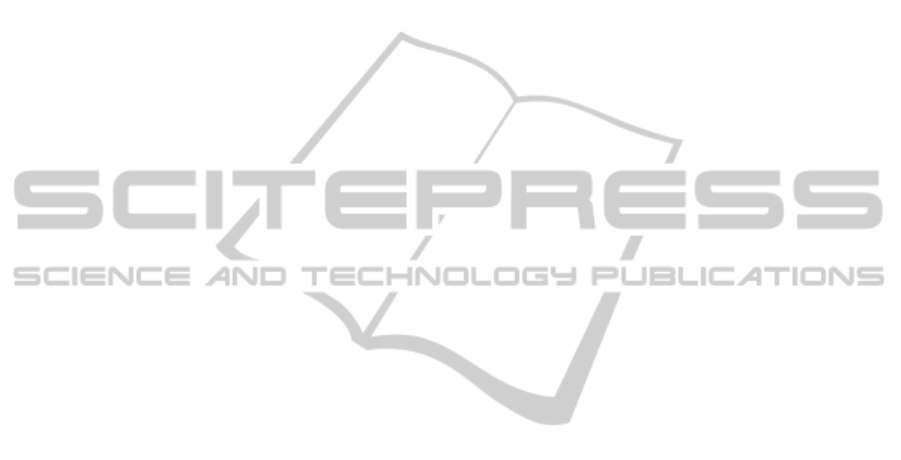
centralized manner! A fully decentralized variant of
COHDA
2
, however, could be realized by including
the ability to detect termination in a self-organizing
way, as well as the capability to spontaneously nomi-
nate a spokesperson from the population of agents, in
order to announce the optimization result.
An important future subject will be to study the in-
fluence of the altruism parameter on the heuristic, i.e.:
How does the resulting global fitness depend on the
setting of α
i
? If the agents are allowed to define this
value on their own, how can we guarantee that the sys-
tem does not collapse? Future work will also include
the analysis of adaptivity, i.e. spontaneously changing
decisions of agents in already converged configura-
tions, or repeatedly varying optimization targets. Ad-
ditionally, we will address the embedding of the math-
ematical representation of device’s action spaces, as
formulated in (Bremer and Sonnenschein, 2012), in
order to circumvent the currently existing premise of
enumerated local search spaces in COHDA
2
with its
disadvantages as described in Section 4.2.3.
ACKNOWLEDGEMENTS
Due to the vast amounts of simulations needed, all
experiments have been conducted on HERO, a multi-
purpose cluster installed at the University of Olden-
burg, Germany. We would like to thank the main-
tenance team from HERO for their valuable service.
We also thank Ontje L
¨
unsdorf for providing the asyn-
chronous message passing framework used in our
simulation environment, and J
¨
org Bremer for provid-
ing the CHP simulation model.
REFERENCES
Bremer, J. and Sonnenschein, M. (2012). A distributed
greedy algorithm for constraint-based scheduling of
energy resources. In SEN-MAS’2012 Workshop, Proc.
of the Federated Conference on Computer Science and
Information Systems, pages 1285–1292, Wrocław,
Poland. IEEE Catalog Number CFP1285N-ART.
Gellings, C. (2009). The Smart Grid: Enabling Energy Ef-
ficiency and Demand Response. The Fairmont Press,
Inc.
Gershenson, C. (2007). Design and Control of Self-
organizing Systems. Copit-Arxives.
Hinrichs, C., Lehnhoff, S., and Sonnenschein, M. (2012).
A Decentralized Heuristic for Multiple-Choice Com-
binatorial Optimization Problems. In Operations
Research Proceedings 2012 – Selected Papers of
the International Conference on Operations Research
(OR 2012), Hannover, Germany. Springer.
Hirayama, K. and Yokoo, M. (1997). Distributed Partial
Constraint Satisfaction Problem. In Principles and
Practice of Constraint Programming, pages 222–236.
H
¨
olldobler, B. and Wilson, E. O. (1990). The Ants. Belknap
Press of Harvard University Press.
Jones, J. C., Myerscough, M. R., Graham, S., and Oldroyd,
B. P. (2004). Honey bee nest thermoregulation: di-
versity promotes stability. Science (New York, N.Y.),
305(5682):402–4.
Jordan, U. and Vajen, K. (2001). Influence Of The DHW
Load Profile On The Fractional Energy Savings: A
Case Study Of A Solar Combi-System With TRNSYS
Simulations. Solar Energy, 69:197–208.
Kaddoum, E. (2011). Optimization under Constraints
of Distributed Complex Problems using Cooperative
Self-Organization. Phd thesis, Universit
´
e de Toulouse.
Kok, J. K., Warmer, C. J., and Kamphuis, I. G. (2005). Pow-
erMatcher. In Proceedings of the fourth international
joint conference on Autonomous agents and multia-
gent systems - AAMAS ’05, page 75, New York, New
York, USA. ACM Press.
Kroeker, K. L. (2011). Biology-inspired networking. Com-
munications of the ACM, 54(6):11.
Li, J., Poulton, G., and James, G. (2010). Coordination of
Distributed Energy Resource Agents. Applied Artifi-
cial Intelligence, 24(5):351–380.
Lust, T. and Teghem, J. (2012). The multiobjective multi-
dimensional knapsack problem: a survey and a new
approach. International Transactions in Operational
Research, 19(4):495–520.
Modi, P., Shen, W., Tambe, M., and Yokoo, M. (2005).
ADOPT: Asynchronous Distributed Constraint Opti-
mization with Quality Guarantees. Artificial Intelli-
gence, 161(1-2):149–180.
Penya, Y. (2006). Optimal Allocation and Scheduling of
Demand in Deregulated Energy Markets. Phd, Vienna
University of Technology.
Pournaras, E., Warnier, M., and Brazier, F. M. (2010). Local
agent-based self-stabilisation in global resource utili-
sation. International Journal of Autonomic Comput-
ing, 1(4):350.
Reynolds, C. W. (1987). Flocks, herds and schools: A
distributed behavioral model. SIGGRAPH Comput.
Graph., 21(4):25–34.
Serugendo, G., Gleizes, M.-P., and Karageorgos, A. (2005).
Self-organisation in multi-agent systems. The Knowl-
edge Engineering Review, 20(2):65–189.
Strogatz, S. H. (2001). Exploring Complex Networks. Na-
ture, 410(March):268–276.
Tero, A., Takagi, S., Saigusa, T., Ito, K., Bebber, D. P.,
Fricker, M. D., Yumiki, K., Kobayashi, R., and Nak-
agaki, T. (2010). Rules for biologically inspired
adaptive network design. Science (New York, N.Y.),
327(5964):439–42.
ICAART2013-InternationalConferenceonAgentsandArtificialIntelligence
34