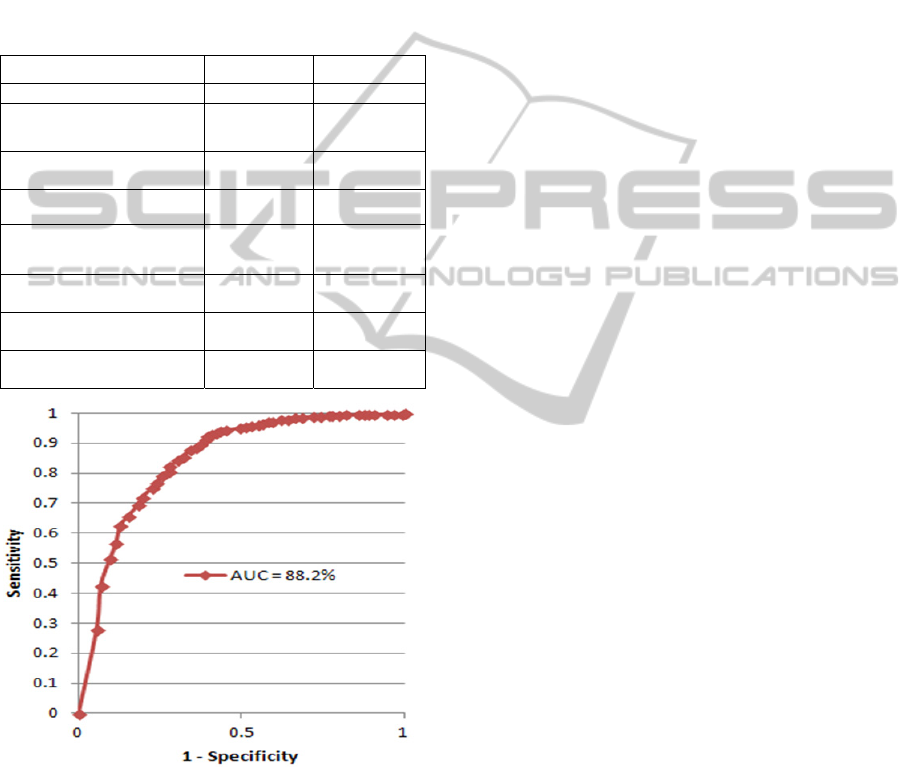
analysis were analyzed by the Statistical Analysis
System (SAS Institute, Cary, NC). The total number
of candidate pixels analyzed by logistic regression
was 1005 candidates from the seborrheic keratosis
images and 473 candidates from the melanoma
images. Significant features determined by logistic
regression (Table I) and the corresponding receiver
operating characteristic curve (Figure 4) are
displayed. The area under the ROC curve for MLC
vs. mimic discrimination is 88.2%.
Table 1: Significant features of logistic regression model.
Parameter Chi-Square Pr > Chi-Sq
Star size 24.6856 < .0001
First correlation coefficient
with ideal star template
29.1704 < .0001
Intensity difference between
star and surround
25.5965 < .0001
Mean of 11x11 star center 8.6837 0.0032
Variance of 11x11 star
center
10.0570 0.0015
Rise time of 11x11 star
center
30.8251 < .0001
Correlation coefficient to
the star shape template
11.1896 < .0008
Equalized correlation
coefficient
29.2425 < .0001
Figure 4: Receiver operating characteristic (ROC) curve
for detection of starry MLCs vs. mimics.
8 CONCLUSION AND FUTURE
WORK
This research was conducted to correctly classify
benign SKs based on automatically detected starry
MLCs. Well-known image processing techniques
were employed. Yet these techniques were
employed in novel ways: 1) new details for region of
interest (ROI) analysis of the centers of star
candidates, 2) template matching for size
determination, 3) a new method for determining
shape of hazy objects, and 4) performance of
multiple template correlations using unprocessed
ROIs, shape-limited ROIs, and histogram-equalized
ROIs. Other methods such as subtraction of a
filtered image from the original image were
attempted, i.e. the LaPlacian of Gaussian method as
a “tuning” filter for the fineness of detection.
However, the differences between star ROIs and
mimics are so tiny that fine details such as central
star range are needed. Because of the fine
discriminations needed, no simple filter or set of
simple blob feature suffices to discriminate starry
MLCs, and subtle intra-blob features were required.
The accuracy and the area under the receiver
operating characteristic curve show that the
algorithm presented here can identify most starry
MLCs and allow accurate classification. Future work
could increase MLC detection accuracy and benign
vs. melanoma discrimination by investigating the
following: 1) using a larger number of images, 2)
using finer gradation of MLC sizes, 3) finding
features of MLC mimics, e.g.. scales, 4) improving
bubble masks, and 5) using additional color planes.
REFERENCES
Braun, R. P., Rabinovitz, H. S., Krischer, J., Kreusch, J.,
Oliviero, M, Naldi, L., … Saurat, J.H. (2002).
Dermoscopy of Pigmented Seborrheic Keratosis: A
Morphological Study. Archives of Dermatology, 138,
1556-1560.
Cheng, Y., Swamisai, R., Umbaugh, S. E., Moss, R. H.,
Stoecker, W.V., Teegala, S., Srinivasan, S.K. (2008).
Skin lesion classification using relative color features.
Skin Research and Technology, 14(1), 53-64.
Gonzalez, R. C. & Woods, R. E. (2002). Digital Image
Processing. 2
nd
ed. New Jersey: Prentice Hall, pp.
698-704.
Lee, T. K. (2001). Measuring border irregularity and shape
of cutaneous melanocytic lesions. Ph.D. Thesis,
Simon Fraser University,Vancouver, British
Columbia.
MATLAB version 7.8.0 (R2009a). 2009. Image
Processing Toolbox. Natick, Massachusetts: The
MathWorks Inc.
Menzies, S. W., Kreusch, J., Byth, K., Pizzichetta, M. A.,
Marghoob, A., Braun, R., …Johr, R. (2008).
Dermoscopic evaluation of amelanotic and
hypomelanotic melanoma. Archives of Dermatology,
144(9), 1120-7.
TemplateMatchingforDetectionofStarryMilia-LikeCystsinDermoscopicImages
447