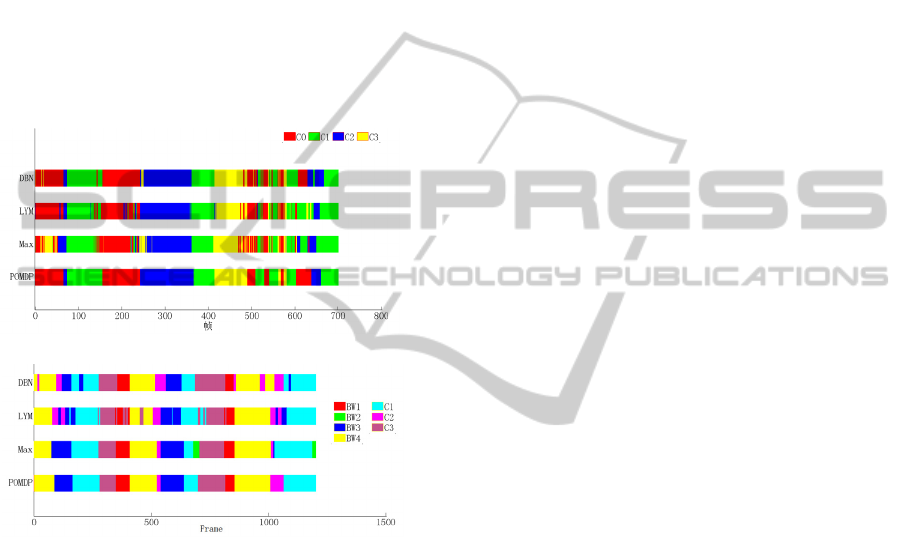
POM Terrace1 sequence on the basis of the camera
quality measures described in Section 4.2, and the
best camera was selected for the other sequences at
intervals of 5 frames. The camera selection results
indicate that there are some frequent camera
switches using LYM and DBN owing to false
selection because errors were introduced in the
motion detection process when there are two people
in the scene. Moreover, the LYM method was
especially prone to frequent switching when the
person utility is approximated to zero. In contrast,
our method effectively predicted future trends of the
visual information scores on the basis of history, and
this reduced the number of false selections, resulting
in smoother visual effects.
(a) POM Terrace1 selection results
(b) HUMANEVA Walk1 selection results
Figure 2: Selection results for the different methods.
5 CONCLUSIONS
Real-time selection of the most informative video
stream from a number of video streams has become
one of the key issues in visual analysis and
processing. The experimental results show that our
proposed POMDP-based method has a higher degree
of accuracy and is more stable than other methods.
In addition, we have proposed a visual information
score function for extracting and scoring visual
features associated with global motion, object
properties, and special events, and this function can
accurately reflect and describe the visual
information in a scene.
ACKNOWLEDGEMENTS
This work is supported by The National Natural Scie
nce Foundation of China (61272219, 61100110 and
61021062), The National High Technology Researc
h and Development Program of China (2007AA01Z
334), The Program for New Century Excellent Talen
ts in University of China (NCET-04-04605) and Th
e Science and technology program of Jiangsu Provin
ce (BE2010072, BE2011058 and BY2012190).
REFERENCES
Soro S, Heinzelman W. 2009. A survey of visual sensor
networks. Advances in Multimedia.
Li Y, Bhanu B. 2009. Utility-Based Camera Assignment
in a Video Network: A Game Theoretic Framework.
IEEE Sensors Journal 11(3).
Daniyal F., Taj M., Cavallaro 2010. A Content and task-
based view selection from multiple video streams.
Multimedia Tools and Applications ,46.
Bimbo A. D., Pernici F. 2006. Towards on-line saccade
planning for high-resolution image sensing. Pattern
Recognition Letters, 27(15).
Tessens L., Morbee M., Huang Lee, Philips W., Aghajan
H. 2008. Principal view determination for camera
selection in distributed smart camera networks. In
Second ACM/IEEE International Conference on
Distributed Smart Cameras.
Littman M. 2009. A tutorial on partially observable
markov decision processes. Journal of Mathematical
Psychology, 53(3).
Spaan M, Vlassis N. 2005. Perseus: Randomized point-
based value iteration for POMDPs. Journal of
Artificial Intelligence Research, vol 24.
Zhang S., Ding H., and Zhang W. 2011. Background
Modeling and Object Detection Based on Two-Model.
Journal of Computer Research and
Development,48(11).
Hu W. M., Hu M., Zhou X., et al. 2006. Principal axis-
base correspondence between multiple cameras for
people tracking. IEEE Transactions on Pattern
Analysis and Machine Intelligence, 28(4).
Laptev I. 2005. On space-time interest points.
International Journal of Computer Vision. Vol 64.
Fleuret F., Berclaz J., Lengane R., Fua P. 2008. Multi-
camera people tracking with a probabilistic occupancy
map. IEEE Transaction on Pattern Analysis and
Machine Intelligence, vol 30(2).
Leonid S., Alexandru O., Michael J. 2010. HUMANEVA:
Synchronized Video and Motion Capture Dataset and
Baseline Algorithm for Evaluation of Articulated
Human Motion. Journal of Computer Vision.
APOMDP-basedCameraSelectionMethod
751