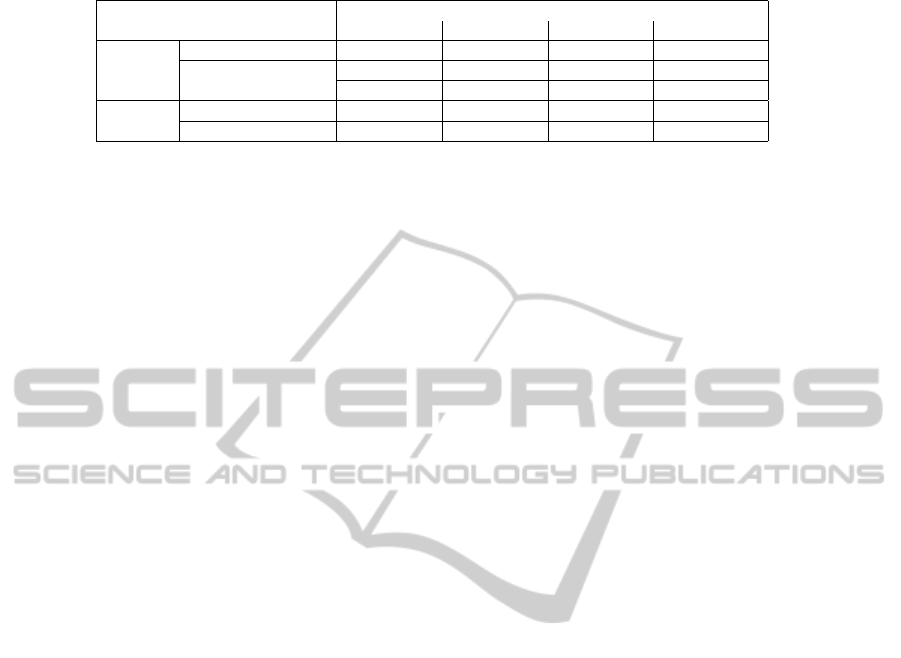
Table 2: Frame rates attained for different image sizes and different devices.
Frames per second (fps)
Image size in pixels
1024 × 128 1024 × 256 1024 × 512 1024 × 1024
Desktop
CPU: i7-2600 23 20.1 16.1 11.5
GPU: GTX 560 Ti 60 60 60 26.8
GPU: GTX 480 60 60 60 40.3
Laptop
CPU: 620M 16 8.5 4.6 2.4
GPU: 3100M 60 32.6 16 7.8
the frame rates are capped at the screen refresh rate
(60 Hz). It is not a limitation incurred by the utilized
algorithms.
In summary, it was shown that using our fuzzy algo-
rithm is beneficial for detecting viruses in PAMONO
data: It increases positive agreement of the detection
results to synthetic ground-truth data. The algorithm,
along with the remainder of the processing pipeline,
achieves real-time performance on a portable device.
The utilization of GPGPU techniques is mandatory
because state-of-the art CPUs do not provide suffi-
cient processing power to satisfy the real-time con-
straint. Using the GPU furthermore saves energy.
5 DISCUSSION
With the increasing global spread of human viral in-
fections and the emergence of highly virulent norovi-
ral strains, the availability of fast, reliable and inex-
pensive methods for virus detection is urgently nec-
essary for screening at e.g. airports or in crisis ar-
eas. The proposed “Portable PAMONO Unit” ful-
fills these requirements. The unit consists of a small
case containing the novel PAMONO biosensor and of
an off-the-shelf laptop computer running specialized
signal analysis software. Besides allowing for ubiqui-
tous availability of virus detection, it accelerates diag-
noses because results are produced in real-time while
viruses attach to the sensor surface.
Future research aims at a further miniaturization
of the “Portable PAMONO Unit” and at running real-
time data analysis on tablet computers and smart
phones. Due to their low cost, a deployment of a large
number of cooperating “Portable PAMONO Units” is
conceivable. A network of such units allows for draw-
ing conclusions about the large-scale propagative be-
havior of pathogens in the human environment.
ACKNOWLEDGEMENTS
Part of the work on this paper has been supported
by Deutsche Forschungsgemeinschaft (DFG) within
the Collaborative Research Center SFB 876 “Provid-
ing Information by Resource-Constrained Analysis”,
project B2. URL: http://sfb876.tu-dortmund.de
REFERENCES
Abbe, E. (1873). Beitr
¨
age zur Theorie des Mikroskops
und der mikroskopischen Wahrnehmung. Archiv f
¨
ur
Mikroskopische Anatomie, 9:413–418.
Cicchetti, D. V. and Feinstein, A. R. (1990). High agree-
ment but low kappa: II. Resolving the paradoxes.
Journal of Clinical Epidemiology, 43(6):551–558.
Erickson, D., Mandal, S., Yang, A., and Cordovez, B.
(2008). Optofluidic, Electrical and Mechanical Ap-
proaches to Biomolecular Detection at the Nanoscale.
Microfluid. Nanofluid., 4:33–52.
Mairhofer, J., Roppert, K., and Ertl, P. (2009). Microflu-
idic systems for pathogen sensing: A review. Sensors,
9(6):4804–4823.
M
´
elange, T. and et al. (2011). Fuzzy random impulse noise
removal from color image sequences. IEEE Transac-
tions on Image Processing, 20(4):959–970.
Mittermayr, C., Nikolov, S., Hutter, H., and Grasserbauer,
M. (1996). Wavelet denoising of Gaussian peaks:
A comparative study. Chemometrics and Intelligent
Laboratory Systems, 34(2):187–202.
Siedhoff, D., Weichert, F., Libuschewski, P., and Timm, C.
(2011). Detection and classification of nano-objects
in biosensor data. Microscopic Image Analysis with
Applications in Biology (MIAAB 2011).
Timm, C., Libuschewski, P., Siedhoff, D., Weichert, F.,
M
¨
uller, H., and Marwedel, P. (2011a). Improving
nanoobject detection in optical biosensor data. Proc.
5th International Symposium on Bio- and Medical In-
formation and Cybernetics (BMIC 2011), 2:236–240.
Timm, C., Weichert, F., Marwedel, P., and M
¨
uller, H.
(2011b). Design space exploration towards a realtime
and energy-aware GPGPU-based analysis of biosen-
sor data. Computer Science – Research and Develop-
ment, Springer, pages 1–9.
Weichert, F., Gaspar, M., Timm, C., Zybin, A., Gurevich,
E., Engel, M., M
¨
uller, H., and Marwedel, P. (2010).
Signal analysis and classification for surface plasmon
assisted microscopy of nanoobjects. Sensors and Ac-
tuators B: Chemical, Elsevier, 151:281–290.
Zybin, A. and et al. (2010). Real-time detection of single
immobilized nanoparticles by surface plasmon reso-
nance imaging. Plasmonics, 5:31–35.
BIOSIGNALS2013-InternationalConferenceonBio-inspiredSystemsandSignalProcessing
174