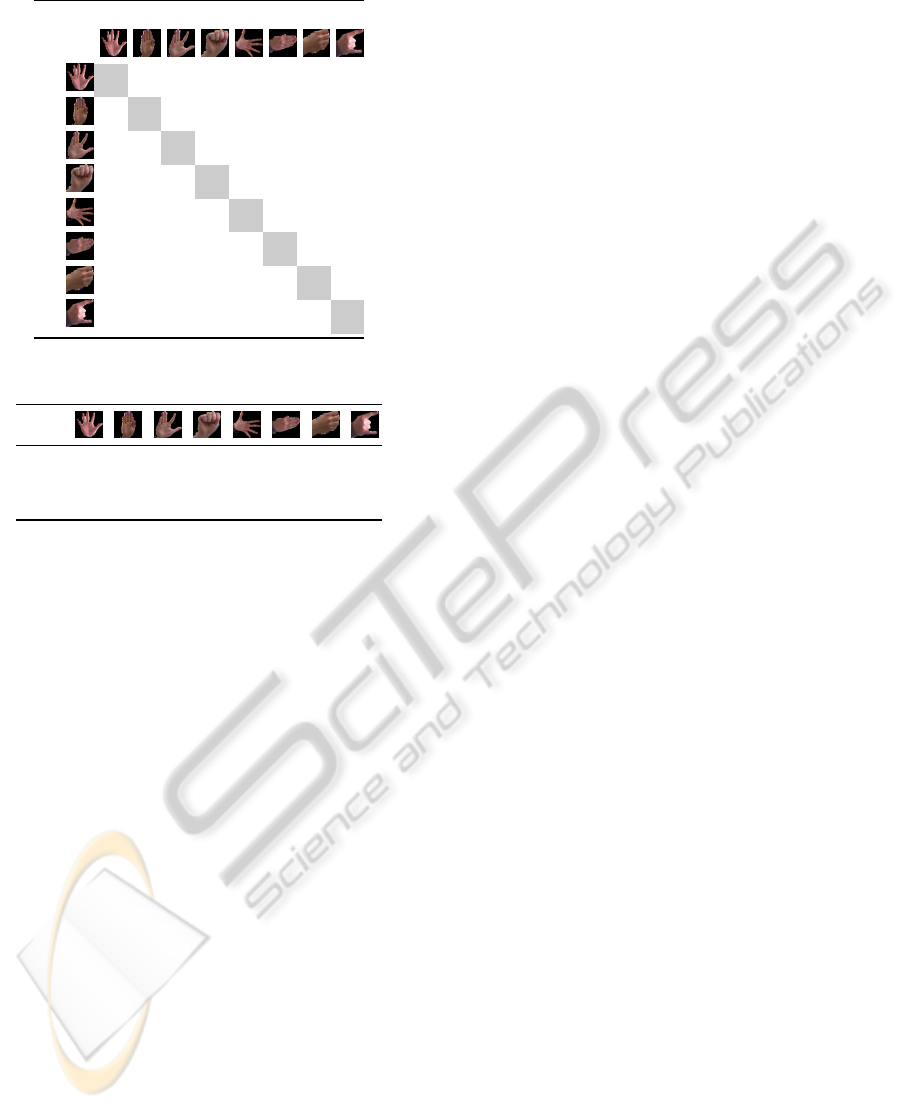
Table 1: Confusion matrix for nearest neighbor approx.
Predicted
Correct
90%10%0% 0% 0% 0% 0% 0%
4% 96%0% 0% 0% 0% 0% 0%
2% 4% 56%38%0% 0% 0% 0%
0% 6% 0% 94%0% 0% 0% 0%
0% 0% 2% 0% 96%0% 2% 0%
0% 0% 0% 0% 0% 70%30%0%
0% 0% 0% 0% 0% 2% 98%0%
0% 0% 0% 0% 4% 42%2% 52%
Table 2: Performance results for nearest neighbor approx.
R
90% 96% 56% 94% 96% 70% 98% 52%
P
94% 83% 97% 71% 96% 61% 74% 100%
Acc
98% 97% 94% 95% 99% 91% 96% 94%
‘1v0v0l0l0’. This is, indeed, much closer to Posture
4. Also, the data used for training showed that esti-
mating folded vs. stretched fingers is not completely
possible using 2D information solely. This can be im-
proved by considering also depth information.
Comparison to Related Work. We compare
against recently introduced 3D SURF (Knopp et al.,
2010), which has not been investigated yet for hand
gesture recognition. Our aim is to show that, although
depth information is essential for robust hand seg-
mentation and may provide benefits for the posture
recognition on its own, 3D descriptors, in their cur-
rent state, do not pay-off for certain problems. We
show that using our approach we obtain better results
than using a more expensive 3D descriptor, which re-
quires both more data and computational time to train
a model. We consider a bag of words approach to-
gether with a multi-class SVM classifier, similarly
as in (Knopp et al., 2010). We obtain the follow-
ing results for 3D SURF: R = 64.5%, P = 71.0% and
Acc = 87.88%, as apposed to R = 81.5%, P = 84.5%
and Acc = 95.5% for our rule-based approach.
REFERENCES
Altun, O. and Albayrak, S. (2011). Turkish fingerspelling
recognition system using generalized hough trans-
form, interest regions, and local descriptors. Pattern
Recognition Letters, 32(13):1626–1632.
Barczak, A. L. C. and Dadgostar, F. (2005). Real-time hand
tracking using a set of cooperative classifiers based on
haar-like features. In Research Letters in the Informa-
tion and Mathematical Sciences, pages 29–42.
Breiman, L., Friedman, J. H., Olshen, R. A., and Stone,
C. J. (1984). Classification and Regression Trees.
Wadsworth.
Darom, T. and Keller, Y. (2012). Scale-invariant features
for 3-d mesh models. IEEE Transactions on Image
Processing, 21(5):2758 –2769.
Erol, A., Bebis, G., Nicolescu, M., Boyle, R. D., and
Twombly, X. (2007). Vision-based hand pose estima-
tion: A review. CVIU, 108(1-2):52–73.
Guyon, I. and Athitsos, V. (2011). Demonstrations and live
evaluation for the gesture recognition challenge. In
ICCV Workshops, pages 461–462.
Guyon, I., Athitsos, V., Jangyodsuk, P., Hamner, B., and Es-
calante, H. J. (2012). Chalearn gesture challenge: De-
sign and first results. In CVPR Workshop on Gesture
Recognition and Kinect Demonstration Competition.
Holt, B., Ong, E.-J., Cooper, H., and Bowden, R. (2011).
Putting the pieces together: Connected Poselets for
Human Pose Estimation. In Workshop on Consumer
Depth Cameras for Computer Vision.
Izadi, S., Kim, D., Hilliges, O., Molyneaux, D., Newcombe,
R., Kohli, P., Shotton, J., Hodges, S., Freeman, D.,
Davison, A., and Fitzgibbon, A. (2011). Kinectfu-
sion: real-time 3d reconstruction and interaction using
a moving depth camera. In ACM symposium on User
interface software and technology, UIST.
Keskin, C., Kira, F., Kara, Y. E., and Akarun, L. (2011).
Real time hand pose estimation using depth sensors.
In Computational Methods for the Innovative Design
of Electrical Devices, pages 1228–1234.
Knopp, J., Prasad, M., Willems, G., Timofte, R., and
Van Gool, L. (2010). Hough transform and 3d surf
for robust three dimensional classification. In ECCV.
Liwicki, S. and Everingham, M. (2009). Automatic recog-
nition of fingerspelled words in British sign language.
In IEEE Workshop for Human Communicative Behav-
ior Analysis, pages 50–57.
Mo, Z. and Neumann, U. (2006). Real-time Hand Pose
Recognition Using Low-Resolution Depth Images.
IEEE Computer Society Conference on Computer Vi-
sion and Pattern Recognition, 2(c):1499–1505.
Pugeault, N. and Bowden, R. (2011). Spelling It Out: Real–
Time ASL Fingerspelling Recognition. In Workshop
on Consumer Depth Cameras for Computer Vision.
Ren, Z., Meng, J., Yuan, J., and Zhang, Z. (2011a). Robust
hand gesture recognition with kinect sensor. In ACM,
MM, pages 759–760, New York, NY, USA. ACM.
Ren, Z., Yuan, J., and Zhang, Z. (2011b). Robust hand
gesture recognition based on finger-earth mover’s dis-
tance with a commodity depth camera. In ACM, MM,
pages 1093–1096, New York, NY, USA. ACM.
Suryanarayan, P., Subramanian, A., and Mandalapu, D.
(2010). Dynamic hand pose recognition using depth
data. In ICPR, pages 3105–3108.
Van den Bergh, M. and Van Gool, L. J. (2011). Combin-
ing rgb and tof cameras for real-time 3d hand gesture
interaction. In WACV, pages 66–72.
ICPRAM2013-InternationalConferenceonPatternRecognitionApplicationsandMethods
542