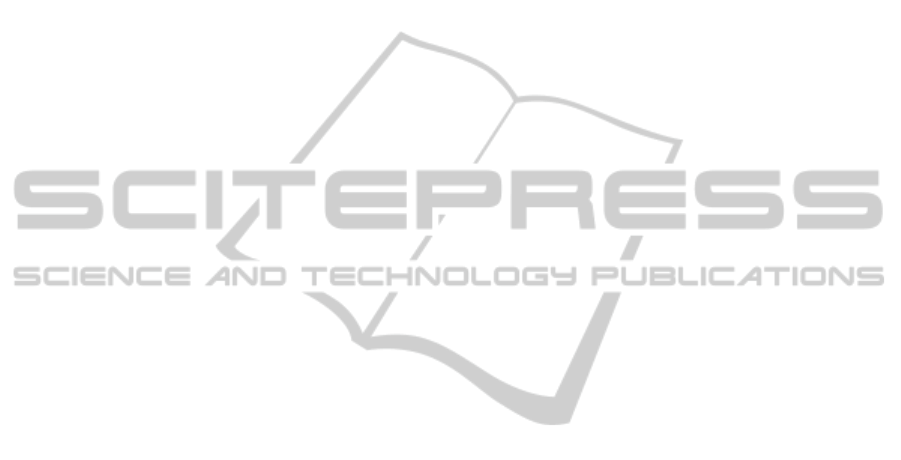
The second order model could be decomposed
into two first order models and suggest that two
major sub-processes underlie mGluRLTD: one slow
and one fast sub-process (see Table 3). A parallel
circuit and a feedback circuit were suggested as
candidate configurations of these two sub-processes.
Possibly, the fast time constants describes the
fast processes immediately after induction mediated
by activation of the ERK/MAPK pathway and
tyrosine dephosphorylation (e.g. of GluR2) with the
tyrosine phosphatase striatal-enriched tyrosine
phosphatase (STEP) as a main player.
The slow time constant, in contrast, is likely to
reflect structural changes, for example in spine
number and morphology, that were demonstrated in
other models of synaptic plasticity to be protein-
synthesis-dependent and to occur on a time-scale of
hours (Fukazawa et al., 2003; Raymond, 2007).
Many studies show the presence of feedback loops
in cellular control systems (Mitrophanov &
Groisman, 2008). Neural mechanisms are known to
contain many non-linearities, but our modelling
results confirm other studies in which discrete-time
linear system identification techniques were
succesfully used for modelling brain signals (e.g.
Liu et al., 2003; Westwick et al., 2006; Behrend et
al., 2009).
5 CONCLUSIONS
Discrete-time TF models are interesting to
investigate mGlu receptor-dependent LTD, because
of their computational and conceptional simplicity
and since they are able to combine the advantages of
a data-based approach (accurate models) with a
mechanistic approach (meaningful parameters). This
study suggests that the dynamic data-based
modelling approach can be a valuable tool for
reverse engineering of mGluR-dependent LTD
responses. Moreover, this approach can also be
extended to other forms of LTD and LTP using
other induction protocols as input for the TF models.
It is expected that such system identification
methods can aid to unravel the complexities of
synaptic function and its role in disease.
REFERENCES
Akaike, H. (1974). A new look at the statistical model
identification. IEEE Transactions on Automatic
Control, 19, 716–723.
Balschun, D., Wolfer, D.P., Gass, P., Mantamadiotis, T.,
Welzl, H., Schutz, G., Frey, J. U., & Lipp, H. P.
(2003). Does cAMP response element-binding protein
have a pivotal role in hippocampal synaptic plasticity
and hippocampus-dependent memory? Journal of
Neuroscience, 23, 6304-6314.
Behrend, C.E., Cassem, S.M., Pallone, M.J.,
Daubenspeck, J.A., Hartov, A., Roberts, D.W., &
Leiter J.C. (2009). Toward feedback controlled deep
brain stimulation: dynamics by metabotropic
glutamate receptors. Journal of Neuroscience
Methods, 23, 6304-6314.
Bellone, C., Lüscher, C., & Mameli, M. (2008).
Mechanisms of synaptic depression triggered by
metabotropic glutamate receptors. Cellular and
Molecular Life Sciences, 65, 2913–23.
Collingridge, G. L., Peineau, S., Howland J. G., & Wang
Y. T. (2010). Long-term depression in the CNS.
Nature Reviews Neuroscience, 11, 459–73.
Fukazawa, Y., Saitoh, Y., Ozawa, F., Ohta, Y., Mizuno,
K., & Inokuchi, K. (2003). Hippocampal LTP is
accompanied by enhanced F-actin content within the
dendritic spine that is essential for late LTP
maintenance in vivo. Neuron, 38, 447–460.
Hou, L., Antion, M.D., Hu, D., Spencer, C.M., Paylor, R.,
& Klan, E. (2006). Dynamic translational and
proteasomal regulation of fragile X mental retardation
protein controls mGluR-dependent long-term
depression. Neuron, 51, 441–454.
Izhikevich, E.M. (2007). Dynamical systems in
Neuroscience. MIT press, Cambridge
Jarvis, A.J., Stauch, V.J., Schulz, K., & Young, P.C.
(2004). The seasonal temperature dependency of
photosynthesis and respiration in two deciduous
forests. Global Change Biology, 10, 939–950.
Kandel, E.R. (2001). The molecular biology of memory
storage: a dialogue between genes and synapses.
Science, 294, 1030–1038.
Kondo, S., & Okabe, S. (2011). Turnover of Synapse and
Dynamic Nature of Synaptic Molecules In Vitro and
In Vivo. Acta Histochem Cytochem, 44, 9–15.
Liu, Y., Birch, A.A., & Allen, R. (2003), Dynamic
cerebral autoregulation assessment using an ARX
model: comparative study using step response and
phase shift analysis. Cell and Tissue Research, 25,
647-653.
Ljung, L. (1987). System identification: theory for the
user. Prentice-Hall, Englewood Cliffs, N.J.,
Lüscher, C., & Huber, K. (2010). Group 1 mGluR-
Dependent Synaptic Long-Term Depression:
Mechanisms and Implications for Circuitry and
Disease. Neuron, 65, 445–459.
Malenka, R.C., & Bear, M.F. (2004). LTP and LTD: an
embarrassment of riches. Neuron, 44, 5–21.
Manninen, T., Hituri, K., Kotaleski, J. H., Blackwell, K.
T., & Linne, M.-L. (2010). Postsynaptic Signal
Transduction Models for Long-Term Potentiation and
Depression. Frontiers in Computational Neuroscience,
4, 152.
Massey, P. V., & Bashir, Z. I. (2007). Long-term
depression: multiple forms and implications for brain
BIOSIGNALS2013-InternationalConferenceonBio-inspiredSystemsandSignalProcessing
52