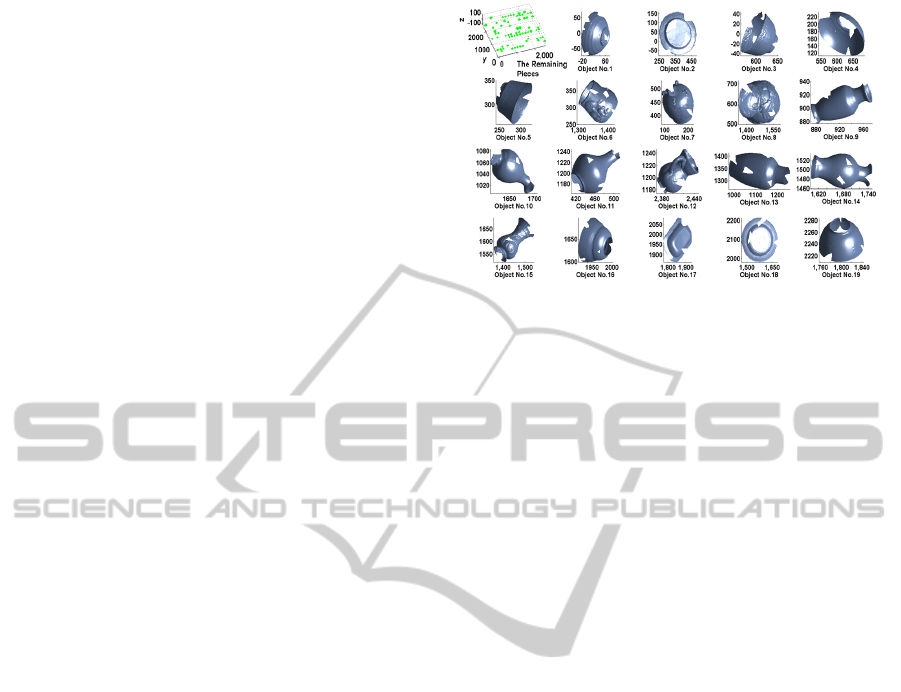
We define the residual error for each fragment as the
mean point-to-point distance map between the
mended fragments and the original unbroken jar.
The mean distance is normalized against the
resolution of the scanner. In all our experiments, the
19 vessels were scanned prior to breaking them into
fragments, with the latter being also scanned
individually.
The described method is tested on 313 fragment
pieces coming from 19 different ceramic objects.
Each fragment is scanned separately.
The mending process for one object is shown in
Figure 2 with all the anchor points showing for two
mended pieces on the vessel. A total of 245 out of
313 fragments were mended properly to their correct
vessels as shown in Figure 6. There were 68 pieces
that could not be mended (See “The Remaining
Pieces” subplot on the upper left of Figure 6) due to
insufficient anchor points.
The entire mending process took 59 seconds on
an Intel i7 processor PC with 18 GB memory for the
mending process, and approximately 60 minutes for
the scanning. This is to be compared to 12 hours for
the stitching done by the experts.
80% fragments were properly mended with
distance map errors at or below the scanner
resolution, i.e. the residual errors normalized by the
scanner resolution were all below or close to 1.
4 CONCLUSIONS
We present a methodology to mend fragments into
vessels based on anchor points on fragment borders.
This work is part of a collaborative project for which
the main objective is to develop and utilize novel
computer vision technology to assist in the
reconstruction of ceramic artifacts recovered from
an excavation site. The work has focused on the use
of one aspect (fragments borders) amongst many
embedded in the fragments. This, in conjunction
with many other aspects such as markings, texture,
or surface information, could be collectively used as
enabling technology helping in the mending process.
This is particularly important if the extracted anchor
points in the paper are absent due to complete
erosion of the fragments borders on abutting
fragments, which would limit the success of such a
method. The whole project as an application of
computer vision in archaeology is unique as an
enabling technology for timely analysis,
interpretation, and presentation of history evidence.
It is also considered as a great need by the U.S.
Department of the Interior National Park Service.
Figure 6: Mended objects.
ACKNOWLEDGEMENTS
This work is supported by the National Science
Foundation IRIS Division under grant #0803670.
REFERENCES
Bratko, I. 1990. Prolog Programming for Arificial
Intelligence. London, UK, Addison Wesley.
Kühnel, W. 2006. Differential geometry : curves - surfaces
- manifolds. Providence, R.I., American Mathematical
Society.
Papaioannou, G. and E. A. Karabassi 2003. "On the
automatic assemblage of arbitrary broken solid
artefacts." Image and Vision Computing 21(5): 401-
412.
Rodriguez, W., M. Last, et al. 2004. "3-Dimensional curve
similarity using string matching." Robotics and
Autonomous Systems 49(3-4): 165-172.
Ucoluk, G. and I. H. Toroslu 1999. "Automatic
reconstruction of broken 3-D surface objects."
Computers & Graphics 23(4): 573-582.
Umeyama, S. 1991. "Least-Square Estimation of
Transformation Parameters Between Two Point
Patterns." IEEE Transactions on Pattern Analysis And
Machine Intelligence 13(4): 376-380.
Willis, A., X. Orriols, et al. 2003. Accurately Estimating
Sherd 3D Surface Geometry with Application to Pot
Reconstruction. CVPR Workshop: ACVA. Madison,
WI, USA, IEEE Computer Society.
ReconstructingArcheologicalVesselsfromFragmentsusingAnchorPointsResidingonShardFragmentBorders
83