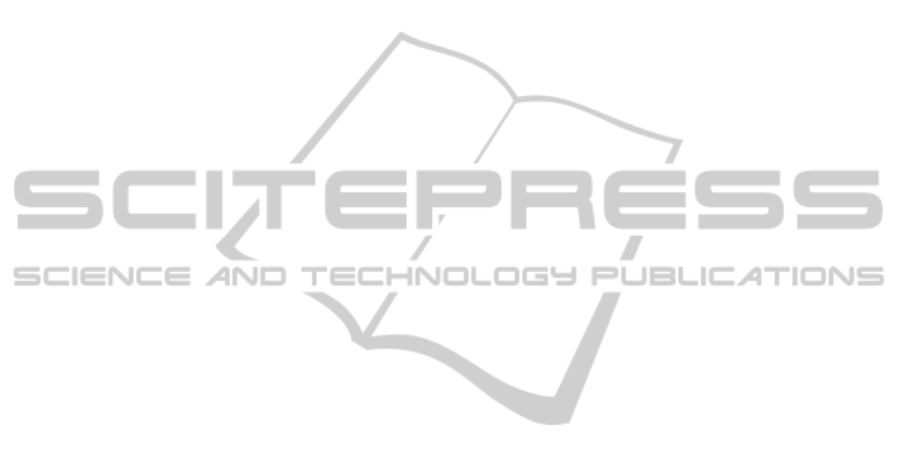
pursuing.
Figure 2(b) shows the cumulative performance on
the test set. The trends in the cumulative performance
curves are similar. This shows the generalizability of
the methods.
6 SUMMARY & FUTURE WORK
In this paper, we investigated a collaborative filter-
ing framework for identifying prescription omission
in ICU admissions. We investigated three different
types of CF methods: a nearest neighbor approach, a
latent factor model and an integrated model. Using
prescription medication data and diagnosis informa-
tion from 19,000 hospital admissions, we tackled the
problem of identifying which medications are likely
to be omitted. The novelty of our work lies in using
CF to investigate multi-scale information, i.e., local-
ized relationships as well as overall prescription pat-
terns, contained in prescription data for the task of
identifying prescription omissions.
All of the CF approaches ranked omitted medica-
tion higher than a prior based algorithm and a simple
co-occurrence approach. Overall, latent factor models
outperformed neighborhood models. Further analy-
sis showed that latent factor models are much better
at identifying omissions of rarely prescribed medica-
tions. Neighborhood models, on the other hand, were
able to yield a slightly better ranking for commonly
prescribed medications. This highlights the relevance
of learning both strong local relationships and overall
patterns from the data for the application of identify-
ing omissions.
For our methods, we envision a use model where
a computerized systems will suggest a list of medica-
tions that are likely to be omitted given the informa-
tion about the patient. A physician then scans through
the top x medications to look for potential omissions.
We conclude with a brief discussion of some fu-
ture work. First, our current integrated approach
optimizes latent factor models independently of the
neighborhood approach. Since our results indicate
that the neighborhood approach and latent factor
model perform better for different kinds of omissions,
an improved approach that intelligently uses localized
relationships and/or prescription patterns will likely
result in better performance. We plan to investigate
advanced models that optimizes latent factor param-
eters, taking into account the information about the
neighbors.
Our work explores CF methods using medication
and diagnosis data for identifying omissions. Clearly,
incorporating other information in electronic medical
records, e.g., a patient’s allergies should yield im-
proved results. Furthermore, we investigated only
data-driven approaches. Hybrid computerized sys-
tems that augment data driven methods with expert
knowledge are also worth pursuing.
ACKNOWLEDGEMENTS
The authors gratefully acknowledge the financial as-
sistance provided by Quanta Computers Inc.
REFERENCES
Aspden, P., Wolcott, J., Bootman, J. L., and Cronenwett,
L. R. (2007). Preventing Medication Errors: Quality
Chasm Series. The National Academies Press.
Cullen, D., Sweitzer, B., Bates, D., Burdick, E., Edmond-
son, A., and Leape., L. (1997). Preventable adverse
drug events in hospitalized patients: a comparative
study of intensive care and general care units. Crit-
ical Care Medicine, 25(8):1289–1297.
Hasan, S., Duncan, G. T., Neill, D. B., and Padman, R.
(2011). Automatic detection of omissions in medi-
cation lists. Journal of the American Medical Infor-
matics Association : JAMIA, 18(4):449–58.
Li, Y., Hu, J., Zhai, C., and Chen, Y. (2010). Improving
one-class collaborative filtering by incorporating rich
user information. In Proceedings of the 19th ACM in-
ternational conference on Information and knowledge
management, CIKM ’10, pages 959–968, New York,
NY, USA. ACM.
Olsen, S., Neale, G., Schwab, K., Psaila, B., Patel, T., Chap-
man, E. J., and Vincent, C. (2007). Hospital staff
should use more than one method to detect adverse
events and potential adverse events: incident report-
ing, pharmacist surveillance and local real-time record
review may all have a place. Quality & safety in health
care, 16(1):40–4.
Rothschild, J., Landrigan, C., Cronin, J., Kaushal, R., Lock-
ley, S., Burdick, E., Stone, P., Lilly, C., Katz, J.,
Czeisler, C., and Bates, D. (2005). The critical care
safety study: The incidence and nature of adverse
events and serious medical errors in intensive care.
Critical Care Medicine, 33(8):1694–1700.
Saeed, M., Lieu, C., Raber, G., and Mark, R. (2002). Mimic
ii: A massive temporal icu patient database to support
research in intelligent patient monitoring. In Comput-
ers in Cardiology, 2002, pages 641 – 644.
Xie, J., Leishman, S., Tian, L., Lisuk, D., Koo, S., and
Blume, M. (2012). Feature Engineering in User‘s Mu-
sic Preference Prediction. Journal of Machine Learn-
ing Research: Workshop and Conference Proceed-
ings, 18:183–197.
Zamir, S. and Gabriel, K. R. (1979). Lower Rank Approxi-
mation of Matrices by Least Squares With Any Choice
of Weights. Technometrics, 21(4).
HEALTHINF2013-InternationalConferenceonHealthInformatics
64