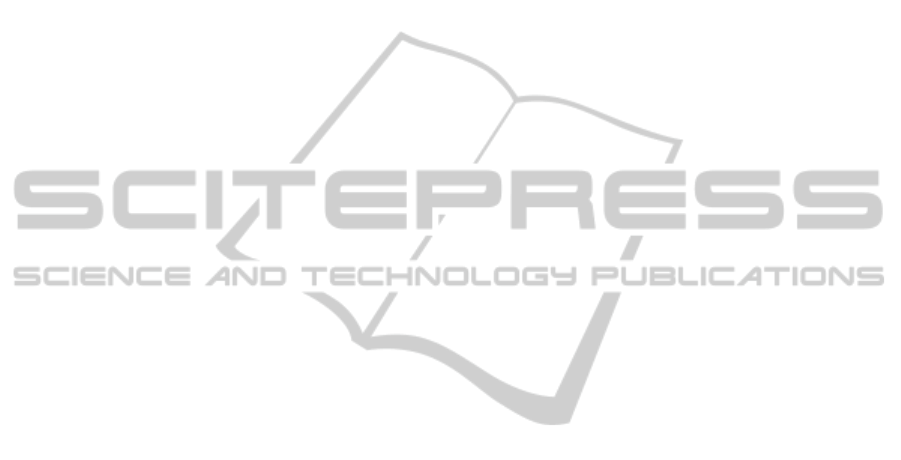
spirometric measures to the expected value for a nor-
mal subject following the guideline provided by the
American Thoracic Society. It was performed as an
attempt to incorporate knowledge from health experts
to the dataset. Our results showed that, despite hav-
ing similar performance in terms of AUC, all classi-
fiers lose the capacity of reduce false negatives when
using the second set of variables.
Among the ANNs models analyzed, RBFNNs ob-
tained similar results in terms of classification power,
but better performance when looking at the classi-
fiers’ sensitivity, for both datasets. This measure tells
us that RBFNN classifiers are more likely to avoid
false negative diagnosis, i.e., cases when a COPD pa-
tient is diagnosed as normal, that may be dangerous.
Such results agree with that obtained by (Mehrabi
et al., 2009) and the performance measures obtained
in our work are slight better, even considering that
other feature set had been considered. The results
obtained with the application of ANN in the classi-
fication of diseases encourage the study of new ap-
plications of such models to help with problems of
biomedicine, pointing out the ANN as a powerful
technique to help with the understanding and diag-
nosing diseases.
In this work, ANNs were used only to identify the
presence or absence of COPD. As future work, it is
intended to apply ANN to classify the level of sever-
ity of the disease as well as to support decision on
treatment, according to this level.
ACKNOWLEDGEMENTS
This work was supported in part by the National
Council for Research and Development of Brazil. We
also thank Prof. Dr. Antonio Fernando Brunetto
(in Memoriam) by his collaboration as the expert in
COPD rehabilitation.
REFERENCES
Azeredo, C. A. C. (2002). Fisioterapia Respirat
´
oria Mod-
erna. Manole, S
˜
ao Paulo, 4 edition.
Er, O. and Temurtas, F. (2008). A study on chronic ob-
structive pulmonary disease diagnosis using multi-
layer neural networks. Journal of Medical Systems,
32:429–432.
Er, O., Yumusak, N., and Temurtas, F. (2010). Chest dis-
eases diagnosis using artificial neural networks. Ex-
pert Systems with Applications, 37(12):7648–7655.
Fawcett, T. (2006). An introduction to ROC analysis. Pat-
tern Recogn. Lett., 27(8):861–874.
Fontenla-Romero, O., Guijarro-Berdinas, B., Alonso-
Betanzos, A., and Moret-Bonillo, V. (2005). A new
method for sleep apnea classification using wavelets
and feedforward neural networks. Artificial Intelli-
gence in Medicine, 34:65–76.
Group, A. W. (2004). Update to the Latin American Tho-
racic Society (ALAT) Recommendations on Infec-
tious Exacerbation of COPD. Archivos de Bronconeu-
mologia, 40:315–325.
Lenfant, C. (1998). Estrat
´
egia global para o diagn
´
ostico, a
conduta e a prevenc¸
˜
ao da doenc¸a pulmonar obstrutiva
cr
ˆ
onica. Technical report, GOLD - Global Initiative
for Chronic Obstructive Lung Disease.
Mehrabi, S., Maghsoudloo, M., Arabalibeik, H., Noor-
mand, R., and Nozari, Y. (2009). Application of mul-
tilayer perceptron and radial basis function neural net-
works in differentiating between chronic obstructive
pulmonary and congestive heart failure diseases. Ex-
pert Systems with Applications, 36(3, Part 2):6956–
6959.
Møller, M. F. (1993). A scaled conjugate gradient algo-
rithm for fast supervised learning. Neural Networks,
6(4):525–533.
Nathell, L., Nathell, M., Malmberg, P., and Larsson, K.
(2007). COPD diagnosis related to different guide-
lines and spirometry techniques. Respiratory Re-
search, 8.
Passold, F., Garcia Ojeda, R., and Muniz Barreto, J. (1996).
Hybrid expert system in anesthesiology for critical pa-
tients. In Electrotechnical Conference, 1996. MELE-
CON ’96., 8th Mediterranean, volume 3, pages 1486–
1489.
Wadie, B. S., Badawi, A. M., Abdelwahed, M., and El-
emabay, S. M. (2006). Application of artificial neu-
ral network in prediction of bladder outlet obstruction:
A model based on objective, noninvasive parameters.
Urology, 68:1211–1214.
Widrow, B. and Hoff, M. E. (1960). Adaptive switching
circuits. In IRE WESCON Convention Record, Part 4,
pages 96–104, New York. IRE.
Yan, H., Jiang, Y., Zheng, J., Peng, C., and Li, Q. (2006). A
multilayer perceptron-based medical decision support
system for heart disease diagnosis. Expert Systems
with Applications, 30(2):272–281.
Zhou, Z.-H. and Jiang, Y. (2003). Medical diagnosis with
c4.5 rule preceded by artificial neural network ensem-
ble. IEEE Transactions on Information Technology in
Biomedicine, 2003, 7(1): 37-42, 7(1):37–42.
HEALTHINF2013-InternationalConferenceonHealthInformatics
214