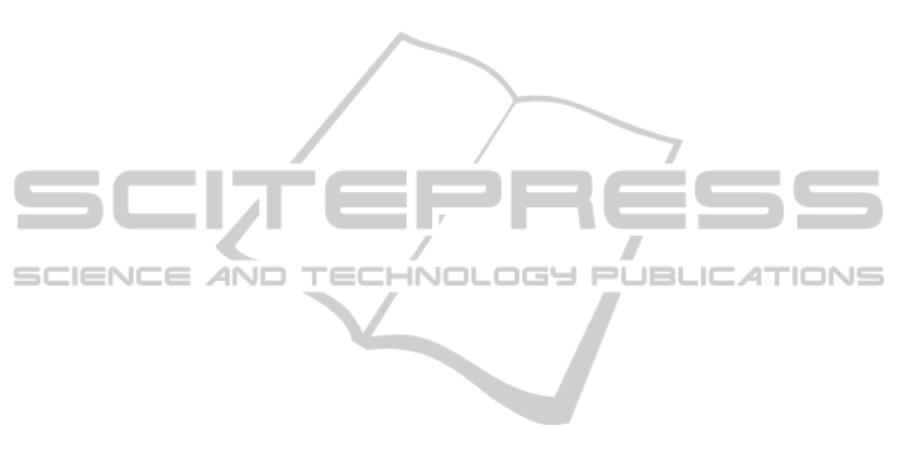
lems in classifying the non-normal heartbeats, for
Dataset B. In Dataset A, the normal class is one of the
most difficult (Table 1). However, we think we can
improve our method by improving the correct identifi-
cation of S1 and S2 in the segmentation and by finding
new features that take advantage of this identification.
5 CONCLUSIONS
In this paper, we present the methodology that won
the Classifying Heart Sounds PASCAL Challenge.
We proposed an algorithm for S1 and S2 heart sound
identification (without ECG reference). The segmen-
tation is accomplished by using the envelope of Shan-
non energy and an algorithm for peak detection. De-
spite of the good performance for the correct detec-
tion of S1 and S2 sounds in the signal, we need to
improve the criteria for identifying S1 and S2 (which
is which). After the segmentation, we used J48 and
MLP algorithms (using Weka) to train and classify the
computed features into Normal, Murmur or Extra sys-
tole for Dataset B and Normal, Murmur, Extra sound
and Artifact for Dataset A. We also compare results
obtained by the other two teams present at the final of
the competition with ours. Stanford obtained the best
results (for Dataset A) on Challenge 1 but did not pro-
vide an answer for Challenge 2. Our method, as well
as the method followed by the UCL team worked bet-
ter for Dataset B (the dataset with less noise) than for
Dataset A. We think these approaches and this com-
parative study provide a good basis for further anal-
ysis of the heart sound signals. In Challenge 2, our
approach with MLP had the highest total precision.
Nevertheless, the UCL team performed better in some
of the partial success measures.
ACKNOWLEDGEMENTS
We would like to acknowledge the financial sup-
port of Fundac¸
˜
ao para a Ci
ˆ
encia e Tecnologia for
the DigiScope project with reference PTDC/EIA-
CCO/100844/2008. We also thank the PASCAL Net-
work of Excellence for supporting the Classifying
Heart Sounds Challenge and Workshop.
REFERENCES
Bentley, P., Nordehn, G., Coimbra, M., and Man-
nor, S. (2011). The PASCAL Classifying Heart
Sounds Challenge 2011 (CHSC2011) Results.
www.peterjbentley.com/heartchallenge.
Billauer (2011). www.billauer.co.il/peakdet.html.
Deng, Y. and Bentley, P. J. (2012). A robust
heart sound segmentation and classification algo-
rithm using wavelet decomposition and spectro-
gram. http://www.peterjbentley.com/heartworkshop/
challengepaper3.pdf.
Groch, M. W., Domnanovich, J. R., and Erwin, W. D.
(1992). A new heart-sounds gating device for medical
imaging. IEEE Transactions on Biomedical Engineer-
ing, 39:307–310.
Gupta, C. N., Palaniappan, R., Swaminathan, S., and Kr-
ishnan, S. M. (2007). Neural network classification of
homomorphic segmented heart sounds. Applied Soft
Computing, 7:286–297.
Kampouraki, A., Manis, G., and Nikou, C. (2009). Heart-
beat Time Series Classification With Support Vector
Machines. IEEE Transactions on Information Tech-
nology in Biomedicine, 13:512–518.
Karraz, G. and Magenes, G. (2006). Automatic Classi-
fication of Heartbeats using Neural Network Classi-
fier based on a Bayesian Framework. In Annual In-
ternational Conference of the IEEE Engineering in
Medicine and Biology Society, pages 4016–4019.
Kumar, D., Carvalho, R., Antunes, M., Gil, R., Henriques,
J., and Eugenio, L. (2006). A New Algorithm for De-
tection of S1 and S2 Heart Sounds. In International
Conference on Acoustics, Speech, and Signal Process-
ing, volume 2.
Liang, H., Lukkarinen, S., and Hartimo, I. (1997). Heart
sound segmentation algorithm based on heart sound
envelogram. Proceedings of The IEEE, pages 105–
108.
MATLAB (2010). version 7.10.0 (R2010a). The Math-
Works Inc., Natick, Massachusetts.
Palm, D., Burns, S., Pasupathy, T., Deip, E., Blair, B.,
Flynn, M., Drewek, A., Sjostrand, M., Stephenson, B.,
and Nordehn, G. (2010). Artificial Neural Network
Analysis of Heart Sounds Captured From an Acous-
tic Stethoscope and Emailed Using iStethoscopePro.
Journal of Medical Devices, 4(2):027531+.
Pereira, D., Hedayioglu, F., Correia, R., Silva, T., Dutra,
I., Almeida, F., Mattos, S., and Coimbra, M. (2011).
Digiscope - unobtrusive collection and annotating of
auscultations in real hospital environments. In En-
gineering in Medicine and Biology Society, EMBC,
2011 Annual International Conference of the IEEE,
pages 1193 –1196.
Stanford, S. (2012). http://www.peterjbentley.com/ heart-
workshop/challengepaper2.pdf.
Strunic, S. L., Rios-gutirrez, F., Alba-flores, R., Nordehn,
G., and Burns, S. (2007). Detection and Classifica-
tion of Cardiac Murmurs Using Segmentation Tech-
niques and Artificial Neural Networks. In IEEE Sym-
posium on Computational Intelligence and Data Min-
ing, pages 128–133.
Witten, I. H. and Frank, E. (2005). Data Mining: Practi-
cal Machine Learning Tools and Techniques. Morgan
Kaufmann.
Yoder, N. C. (2009). www.mathworks.com/matlabcentral.
HEALTHINF2013-InternationalConferenceonHealthInformatics
340