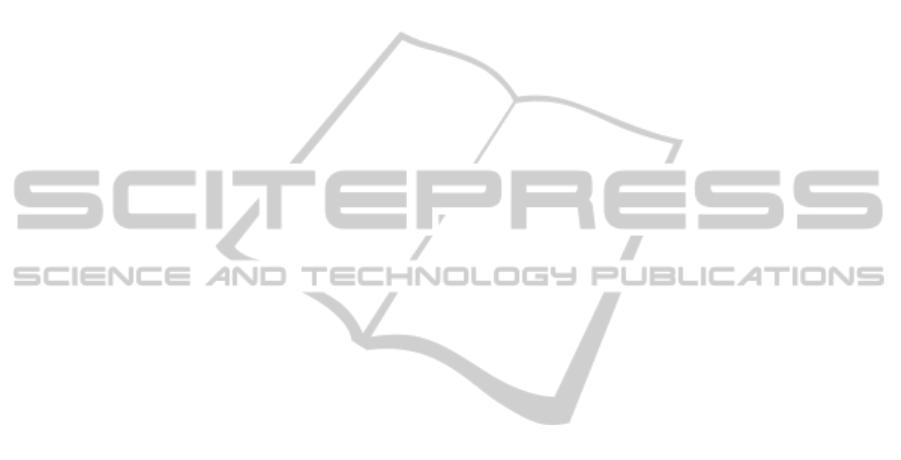
process enables the subject to learn how to better use
the motor imagery control. Some researchers consid-
ering a feedback stage and this training can provide a
performance improvement (Veen, 2009).
In this work no feedback was provided to the sub-
ject and a 2.5 s data period was considered, that com-
paratively to others (Xu and Song, 2008; Kumar and
Fumitoshi, 2010; Huang and Wu, 2010; Dolezal et al.,
2011) is a short period. Nevertheless, the classifica-
tion rates were consistent or even higher than those
achieved by other systems.
On the other hand, the lower accuracies achieved
for the classification between right and left arm, right
and left limbs, and also to distinguish the four motor
imagery set up, situations that were not found in the
literature for comparison, need further investigation.
Maybe a higher period of time and other electrodes
could be considered to provide more information to
the classifier. Other features and classifiers might be
tested in order to evaluate their performances under
this environment set up.
4 CONCLUSIONS
This work presented a motor imagery classification
system that uses a non-conventional electrode set up
and a spatial feature selection aiming at distinguish-
ing up to four upper limb motor imagery. The results
suggest that in addition to the motor areas (C3 and
C4) other brain areas should be considered. New sets
of experiments were proposed to classify between left
arm and left hand movement imagination and to dis-
criminate between arms and hands, resulting in high
classification accuracy. Furthermore, the classifica-
tion of 4 upper limb motor imagery was evaluated
and, for that, the results have shown that further im-
provements, such as the use of more electrodes, the
increase of the data period, and the use of other fea-
tures and classifiers are required. Finally, in order to
generalize the results, experiments with more subjects
are necessary.
ACKNOWLEDGEMENTS
The authors would like to thank FEI, CNPq and
FAPESP for supporting.
REFERENCES
Dolezal, J., Cerny, V., and Stastny, J. (2011). Constructing
a Brain-Computer Interface. In International Confer-
ence on Applied Electronics, pages 1–4.
Hema, C. R., Paulraj, M. P., Yaacob, S., Adom, A. H., and
Nagarajan, R. (2009). EEG Motor Imagery Classifi-
cation of Hand Movements for a Brain Machine In-
terface. Biomedical Soft Computing and Human Sci-
ences, 14(2):49–56.
Hema, C. R., Paulraj, M. P., Yaacob, S., Adom, A. H., and
Nagarajan, R. (2010). An Analysis of the Effect of
EEG Frequency Bands on the Classification of Mo-
tor Imagery Signals. Biomedical Soft Computing and
Human Sciences, 16(1):121–126.
Higashi, H., Tanaka, T., and Funase, A. (2009). Clas-
sification of single trial EEG during imagined hand
movement by rhythmic component extraction. In 31st
Annual International Conference of the IEEE EMBS,
pages 2482–5.
Hoffmann, U., Vesin, J.-M., and Ebrahimi, T. (2007). Re-
cent advances in brain-computer interfaces. In IEEE
International Workshop on Multimedia Signal Pro-
cessing, pages 1–8, Chania, Greece. IEEE.
Huang, S. and Wu, X. (2010). Feature extraction and clas-
sification of EEG for imagery movement based on
mu/beta rhythms. In 3rd International Conference on
Biomedical Engineering and Informatics, volume 2,
pages 891–894. IEEE.
Kumar, M. and Fumitoshi, M. (2010). Relative Spectral
Power ( RSP ) and Temporal RSP as Features for
Movement Imagery EEG Classification with Linear
Discriminant Analysis. In SICE Annual Conference,
pages 439–448, Taipei, Taiwan.
Millan, J. D. R., Rupp, R., Muller-Putz, G. R., Murray-
Smith, R., Giugliemma, C., Tangermann, M., Vidau-
rre, C., Cincotti, F., Kubler, A., Leeb, R., Neuper, C.,
Muller, K. R., and Mattia, D. (2010). Combining
Brain-Computer Interfaces and Assistive Technolo-
gies: State-of-the-Art and Challenges. Frontiers in
Neuroscience, 4:161–193.
Morash, V., Bai, O., Furlani, S., Lin, P., and Hallett, M.
(2008). Classifying EEG signals preceding right hand,
left hand, tongue, and right foot movements and motor
imageries. Clinical Neurophysiology, 119(11):2570–
8.
Veen, E. R. G. V. D. (2009). Survey of state-of-the-art
eeg-based bci systems as reliable computer interface
mechanisms. In 11th Twente Student Conference on
IT, pages 1–7. University of Twente.
Xiao, D., Mu, Z., and Hu, J. (2009). A Linear Discrimi-
nation Method Used in Motor Imagery EEG Classifi-
cation. In Fifth International Conference on Natural
Computation, pages 94–98. IEEE.
Xu, B.-G. and Song, A.-G. (2008). Pattern recognition of
motor imagery EEG using wavelet transform. Journal
of Biomedical Science and Engineering, 1(May):64–
67.
EEGMotorImageryClassificationofUpperLimbMovements
317