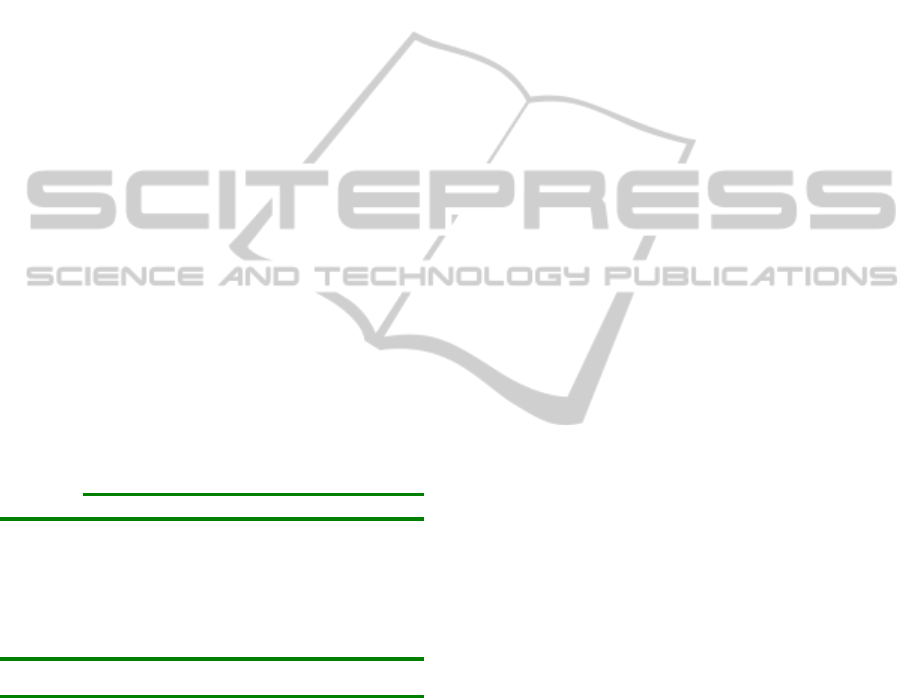
To summarize, the optimum parameters of the
proposed bio-inspired method are to filter the
normalized 128×128 face images by a 4-scale DOG.
Then, compute LBP
U2
histograms on each 32×32
block of the Regions Of Interest and concatenate
them into a single feature vector. Afterward, perform
matching using Chi-square distance weighting both
scales and regions.
5.4 Comparison with IV² Evaluation
Campaign
To evaluate the efficiency of the proposed
algorithm, comparison with five other algorithms
using the same IV² database and protocol is
presented in Table 4 (Mellakh et al., 2009).
According to these results, proposed algorithm
provides the best results in Experiment1 including
small expression variation. It occupies the second
place in Experiments 2 and 3 involving illumination
and quality variations; and it is placed third when
faced to multisession variation in Experiment 4.
It can be seen that proposed method outperforms
conventional algorithms, i.e. PCA1, PCA2 and
LDA, in almost experiments. Besides, when
compared to modular PCA, that makes also use of
Regions Of Interest, proposed algorithm performs
better in all Experiments.
Table 4: Comparative results between proposed algorithm
(green) and IV² first evaluation campaign ones (black).
On the other hand, it is true that both bio-inspired
LBP and LDA/Gabor are multiscale and based on
combining conventional. But it has to be underlined
that, unlike the bio-inspired LBP algorithm, which
does not include any pretreatment for the face
images nor any pretreatment phase, the LDA/Gabor
algorithm performs an anisotropic smoothing on
images, before features extraction, which proved to
be very efficient face to variabilities such as
illumination, quality and multisessions. Also it
includes a learning phase to get the projection space
(Fisherfaces) used afterwards in the test phase. That
explains why LDA/Gabor achieves the best results
for Experiments 2, 3 and 4.
6 CONCLUSIONS AND FUTURE
WORK
Through this work, a bio-inspired approach based on
psychovisual studies has been introduced for 2D-
face authentication. The approach combines LBP
U2
with multiscale DOGs. It has been proved that
considering only relevant regions and weighting
both regions and scales brought sensitive
improvements. Proposed method showed to be
robust not only in controlled environment but also
face to illumination and quality variations.
Future works are intended to investigate
pretreatment before feature extraction. Further
research would consider a learning stage to enhance
performance.
REFERENCES
Belhumeur P. N., Hespanha J. P. and Kriegman D. J.,
1997. Eigenfaces vs fisherfaces: Recognition using
class specific linear projection. In IEEE Trans. on
PAMI, 19(7):711–720.
Gosselin F. and Schyns P. G., 2001. Bubbles: a technique
to reveal the use of information in recognition tasks. In
Vision Research, Elsevier. 41:2261–2271.
Huang D., Shan C., Ardabilian M. and Wang Y., 2011.
Local Binary Patterns and Its Application to Facial
Image Analysis: A Survey. In IEEE Trans. On
Systems, Man, and Cybernetics, 41(6):765-781.
Li S. Z. and Jain A. K., 2005. Handbook of Face
Recognition. Springer.
Mäenpää T., Ojala T., Pietik¨ainen M. & Soriano M.,
2000. Robust texture classification by subsets of local
binary patterns. In Proc. 15th Inter. Conf. on Pattern
Recognition, 3: 947–950.
Mellakh A., Chaari A., Guerfi S. & all., 2009. 2D Face
Recognition in the IV² Evaluation Campaign. In Conf.
on ACIVS, Bordeaux, France.
Ojala T., Pietikäinen M., and Mäenpää T., 2002.
Multiresolution gray-scale and rotation invariant
texture classification with local binary patterns, In
IEEE Trans. on PAMI, 24(7), 971–987.
Petrovska-Delacretaz D., Lelandais S., Colineau J. & all.,
2008. The IV
2
Multimodal Biometric Database
(Including Iris, 2D, 3D, Stereoscopic, and Talking
Face Data), and the IV2- 2007 Evaluation Campaign.
In 2nd IEEE Inter. Conf. on Biometrics: Theory,
Applications and Systems (BTAS), USA.
Pietikäinen M. and Ojala T., 1999. Nonparametric texture
analysis with simple spatial operators. In Proc. on
Algorithms Exp1 Exp2 Exp3 Exp4
PCA1
6.7
(±0.8)
20.7
(±1.3)
20.1
(±1.6)
22.2
(±1.6)
PCA2
7.3
(±0.8)
21.6
(±1.4)
13.6
(±1.4)
16.3
(±1.4)
mod PCA
5.3
(±0.7)
20.7
(±1.4)
19.5
(±1.6)
20.5
(±1.5)
LDA 3.7
(±0.6)
22.5
(±1.4)
21.7
(±1.7)
19.7
(±1.5)
Proposed
method
Bio-inspired
LBP
u2
3.7
(±0.6)
15.2
(±1.2)
12.8
(±1.3)
16.7
(±1.4)
12.0
(±1.1)
8.3
(±1.1)
11.3
(±1.2)
LDA/ Gabor
Previous
Tests IV²
4.2
(±0.6)
Bio-inspiredFaceAuthenticationusingMultiscaleLBP
187