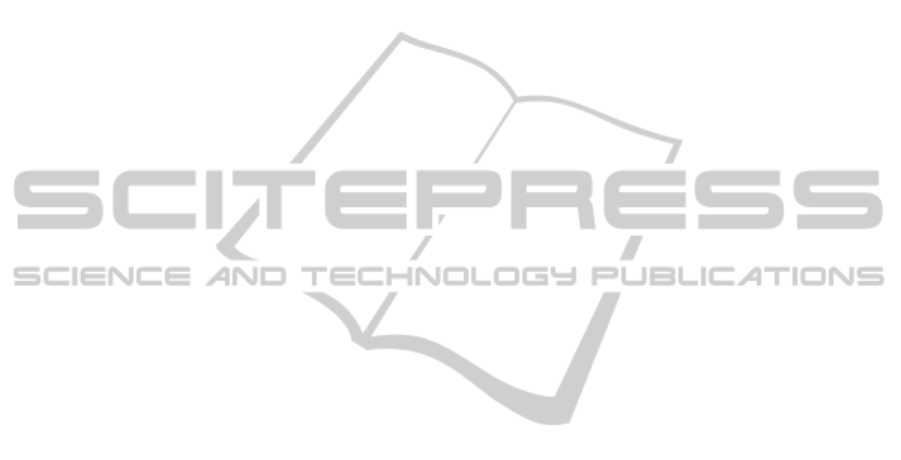
influences the accuracy significantly. While 9 train-
ing examples is used, the recognition rate is 72.7%. If
we use only 3 training examples the recognition rate
is only 21.3%.
In the last two experiments we employed the con-
fidence measure to post-process the recognition re-
sults. We compared the results when 7 training ex-
amples and 3 examples are used. The results show
that using confidence measure is very beneficial for
AFR under real-world conditions.
It is obvious that the AFR methods are nowadays
capable to recognize faces perfectly under the condi-
tion: the acquisition of the face images must be con-
trolled. If this condition is not accomplished, the task
is much more difficult. Therefore the perspectives of
the further work on recognition of real-world data lay
more in the detection step than in the recognition it-
self. Further increase of image quality will ensure
much better accuracy of the recognition. Using higher
quality images and utilising the confidence measure
will help to create a reliable recognition system.
ACKNOWLEDGEMENTS
This work has been partly supported by the UWB
grant SGS-2010-028 Advanced Computer and Infor-
mation Systems and by the European Regional De-
velopment Fund (ERDF), project NTIS - New Tech-
nologies for Information Society, European Centre of
Excellence, CZ.1.05/1.1.00/02.0090. We also would
like to thank Czech New Agency (
ˇ
CTK) for support
and for providing the photographic data.
REFERENCES
Aly, M. (2006). Face recognition using sift features.
CNS/Bi/EE report 186.
Belhumeur, P. N., Hespanha, J. a. P., and Kriegman, D. J.
(1997). Eigenfaces vs. fisherfaces: Recognition using
class specific linear projection. IEEE Transactions on
Pattern Analysis and Machine Intelligence.
Bolme, D. S. (2003). Elastic Bunch Graph Matching. PhD
thesis, Colorado State University.
Jiang, H. (2005). Confidence measures for speech recog-
nition: a survey. Speech Communication, 45(4):455–
470.
Kepenekci, B. (2001). Face Recognition Using Gabor
Wavelet Transform. PhD thesis, The Middle East
Technical University.
Kr´al, P., Cerisara, C., and Kleˇckov´a, J. (2006). Automatic
Dialog Acts Recognition based on Sent ence Struc-
ture. In ICASSP’06, pages 61–64, Toulouse, France.
Krizaj, J., Struc, V., and Pavesic, N. (2010). Adaptation of
sift features for robust face recognition.
Lades, M., Vorbr¨uggen, J. C., Buhmann, J., Lange, J., and
von der Malsburg, C. (1993). Distortion invariant
object recognition in the dynamic link architecture.
IEEE Transactions On Computers.
Lawrence, S., Giles, S., Tsoi, A., and Back, A. (1997).
Face recognition: A convolutional neural network ap-
proach. IEEE Trans. on Neural Networks.
Lenc, L. and Kr´al, P. (2011). Confidence measure for au-
tomatic face recognition. In International Conference
on Knowledge Discovery and Information Retrieval,
Paris, France.
Lenc, L. and Kr´al, P. (2012a). Gabor wavelets for automatic
face recognition. In 38th International Conference on
Current Trends in Theory and Practice of Computer
Science,
ˇ
Spindler˚uv Ml´yn, Czech Republic.
Lenc, L. and Kr´al, P. (2012b). Novel matching methods
for automatic face recognition using sift. In 8th AIAI
(Artificial Intelligence Applications and Innovations)
Confence, Halkidiki, Greece.
Li, S. and Jain, A. (2005). Handbook of face recognition.
Springer-Verlag.
Lleida, E. and Rose, R. C. (1996). Likelihood Ratio Decod-
ing and Confidence Measures for Continuous Speech
Recognition. In ICSLP’96, volume 1, pages 478–481,
Philadelphia, USA.
Lowe, D. (2004a). Software for sift.
Lowe, D. G. (1999). Object recognition from local scale-
invariant features. In International Conference on
Computer Vision.
Lowe, D. G. (2004b). Distinctive image features from scale-
invariant keypoints. International Journal of Com-
puter Vision, 2.
Nefian, A. V. and Hayes, M. H. (1998). Hidden markov
models for face recognition. In IEEE International
Conference on Acoustics, Speech, and Signal Process-
ing.
Samaria, F. and Young, S. (1994). Hmm-based architecture
for face identification. Image and Vision Computing.
Shen, L. (2005). Recognizing Faces - An Approach Based
on Gabor Wavelets. PhD thesis, University of Not-
tingham.
Shen, L. and Bai, L. (2006). A review on gabor wavelets
for face recognition. Pattern Analysis & Applications.
Tan, P.-N., Steinbach, M., and Kumar, V. (2005). Introduc-
tion to Data Mining. Addison-Wesley.
Turk, M. A. and Pentland, A. P. (1991). Face recognition
using eigenfaces. In IEEE Computer Society Confer-
ence on In Computer Vision and Pattern Recognition.
Computer Vision and Pattern Recognition.
Viola, P. and Jones, M. (2001). Rapid object detection using
a boosted cascade of simple features. In Conference
on Computer Vision and Pattern Recognition.
Wiskott, L., Fellous, J.-M., Kr¨uger, N., and von der Mals-
burg, C. (1999). Face recognition by elastic bunch
graph matching. Intelligent Biometric Techniques in
Fingerprint and Face Recognition.
ICAART2013-InternationalConferenceonAgentsandArtificialIntelligence
256