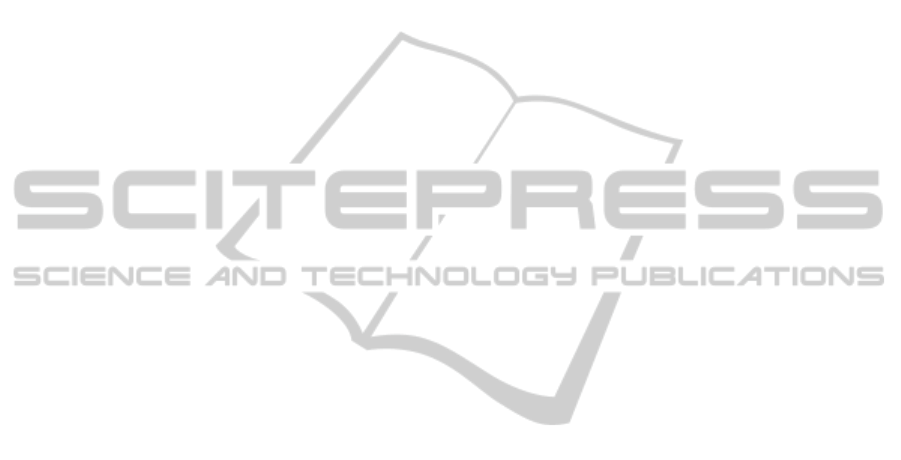
each model we plotted a ROC curve for each TF and
computed an area-under-curve (AUC) value that
allows comparing TFBS recognition quality.
3 RESULTS AND CONCLUSIONS
Figure 1 shows ROC curves comparing diPWMs
versus mononucleotide PWMs constructed from the
same ChIP-Seq data and existing TRANSFAC
PWMs. Motif LOGO representations are given.
AUC values are presented directly on graphs.
diPWMs clearly outperformed models based on
single nucleotide PWMs for all tested datasets (see
Figure 1). However, previously it was shown that
not all TFs profit from diPWMs (Levitsky, 2007) so
a more comprehensive study of various ChIP-Seq
datasets remains highly important.
We have estimated computational performance
of diChIPMunk versus its mononucleotide precursor
using 4 threads for Core i7 CPU. Since a
dinucleotide model has more parameters to train the
default number of starting random seeds and
subsampling runs is doubled for diChIPMunk. The
computationsl performance was acceptable (1 to 8
hours to train the diPWM including length
estimation; the absolute values for mononucleotide
models of ChIPMunk are 2 to 4 times better).
Dinucleotide models derived from ChIP-Seq data
performed significantly better than their
mononucleotide analogs in four independent ChIP-
Seq datasets. Dinucleotide models require more
computational power to be carefully trained, but it is
still possible even using a desktop computer. With
the increasing availability of different types of high-
throughput data we suspect the improved models
becoming widely used. The next step is open for
novel post-processing tools that would allow model
comparison and effective genome-scale prediction of
binding sites.
ACKNOWLEDGEMENTS
This work was supported by a Dynasty Foundation
Fellowship [to I.V.K.]; Russian Foundation for
Basic Research [12-04-32082 to I.V.K.] and [12-04-
01736-a to D.O.]; Presidium of the Russian
Academy of Sciences program in Cellular and
Molecular Biology.
REFERENCES
Stormo, G. D., (2000). DNA binding sites: representation
and discovery. Bioinformatics, 16(1):16-23.
Thomas-Chollier, M., Darbo, E., Herrmann, C., Defrance,
M., Thieffry, D., van Helden, J., (2012). A complete
workflow for the analysis of full-size ChIP-seq (and
similar) data sets using peak-motifs. Nat Protoc.,
7(8):1551-68.
Bi, Y., Kim, H., Gupta, R., Davuluri, R. V., (2011). Tree-
based position weight matrix approach to model
transcription factor binding site profiles. PLoS One.,
6(9):e24210.
SantaLucia., J. Jr., Hicks, D., (2004). The thermodynamics
of DNA structural motifs. Annu Rev Biophys Biomol
Struct., 33:415-40.
Gershenzon, N. I., Stormo, G. D., Ioshikhes, I. P., (2005).
Computational technique for improvement of the
position-weight matrices for the DNA/protein binding
sites. Nucleic Acids Res., 33(7):2290-301.
Levitsky, V. G., Ignatieva, E. V., Ananko, E. A., Turnaev,
I. I., Merkulova, T. I., Kolchanov, N. A., Hodgman, T.
C. (2007). Effective transcription factor binding site
prediction using a combination of optimization, a
genetic algorithm and discriminant analysis to capture
distant interactions. BMC Bioinformatics, 8:481.
Zhao, Y., Ruan, S., Pandey, M., Stormo, G. D. (2012).
Improved models for transcription factor binding site
identification using nonindependent interactions.
Genetics, 191(3):781-90.
Kulakovskiy, I. V., Boeva, V. A., Favorov, A. V.,
Makeev, V. J. (2010). Deep and wide digging for
binding motifs in ChIP-Seq data. Bioinformatics,
26(20):2622-3.
Kulakovskiy I. V., Medvedeva Y. A., Schaefer U.,
Kasianov A. S., Vorontsov I. E., Bajic V.B., Makeev
V. K., (2012) HOCOMOCO: a comprehensive
collection of human transcription factor binding sites
models. Nucleic Acids Res, in press.
Ma, X., Kulkarni, A., Zhang, Z., Xuan, Z., Serfling, R.,
Zhang, M. Q. (2012). A highly efficient and effective
motif discovery method for ChIP-seq/ChIP-chip data
using positional information. Nucleic Acids Res.,
40(7):e50.
Kuttippurathu, L., Hsing, M., Liu, Y., Schmidt, B.,
Maskell, D. L., Lee, K., He, A., Pu, W. T., Kong, S.
W., (2011). CompleteMOTIFs: DNA motif discovery
platform for transcription factor binding experiments.
Bioinformatics, 27(5):715-7.
Matys, V., Kel-Margoulis, O. V., Fricke, E., Liebich, I.,
Land, S., Barre-Dirrie, A., Reuter, I., Chekmenev, D.,
Krull, M., Hornischer, K., Voss, N., Stegmaier, P.,
Lewicki-Potapov, B., Saxel, H., Kel, A. E.,
Wingender, E., (2006). TRANSFAC and its module
TRANSCompel: transcriptional gene regulation in
eukaryotes. Nucleic Acids Res., 34(Database
issue):D108-10.
Touzet, H., Varré, J. S. (2007). Efficient and accurate P-
value computation for Position Weight Matrices.
Algorithms Mol Biol., 11;2:15.
LearningAdvancedTFBSModelsfromChip-SeqData-diChIPMunk:EffectiveConstructionofDinucleotidePositional
WeightMatrices
149