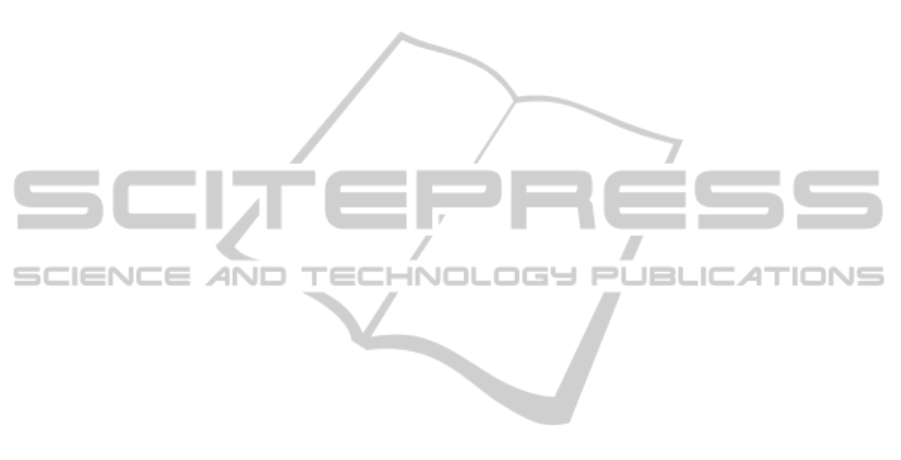
methods of PROST (Jakob Santner, 2010),
MIL_TR004_c (B. Babenko, 2009), FragTrack (A.
Adam, 2006), and GRAD (Klein, 2011). It shows
that our method achieves a best performance in
sequences of board and lemming, and a slightly less
good performance than PROST in sequences of
liquor and box. An average PASCAL score of our
method over the four sequences is 88.75%, which is
much better than the average of 80.375% for PROST
method.
5 CONCLUSIONS
This paper described a novel tracking method based
on a 2D DNF of weak classifiers. The data of the
DNF cell classifiers are constituted by pairwise
combinations of the data of weak classifiers, and
therefore the DNF can be utilized on top of any
weak classifiers. The image patch is determined to
belong to the object category or the background
category by an ensemble of weak classifiers and
DNF cell classifiers. The experiments demonstrate
that our method provides a good performance
compared to other methods but sometimes the center
difference is somewhat large due to the unvaried
object bounding box. For better tracking, we will
continue the present line of research with a scalable
object bounding box in the future.
ACKNOWLEDGEMENTS
This work was supported by the Basic Science
Research Program through the National Research
Foundation of Korea (NRF) funded by the Ministry
of Education, Science and Technology (No. 2009-
0090165, 2011-0017228).
REFERENCES
Avidan S., 2004. Support Vector Tracking. In IEEE Trans.
On Pattern Analysis and Machine Intelligence.
Stauffer, C. and E. Grimson, 2000. Learning Patterns of
Activity Using Real-Time Tracking. In PAMI,
22(8):747-757.
S. Avidan, 2005. Ensemble tracking. In Proc. CVPR,
volume 2, pages 494–501.
H. Grabner and H. Bischof, 2006. On-line boosting and
vision. In Proc. CVPR, volume 1, pages 260–267.
H. Grabner, C. Leistner, and H. Bischof, 2008. Semi-
supervised on-line boosting for robust tracking. In
Proc. ECCV.
S. Stalder, H. Grabner, and L. van Gool, 2009. Beyond
Semi-Supervised Tracking: Tracking Should Be as
Simple as Detection, But Not Simpler than
Recognition. In Proc. Workshop Online Learning in
Computer Vision.
O. Danielsson, B. Rasolzadeh, and S.Carlsson, 2011.
Gated Classifiers: Boosting under High Intra-Class
Variation. In Proc. CVPR.
N. Oza and S. Russell, 2001. Online bagging and boosting.
In Proc. Artificial Intelligence and Statistics, pages
105–112.
Freund, Y. Schapire, R. E, 1995. A decision-theoretic
generalization of on-line learning and an application to
boosting. In Computational Learning Theory:
Eurocolt 95, pp 23-37.
Comanciu, D., Visvanathan R., Meer. P, 2003. Kernel-
Based Object Tracking. In IEEE Trans. on Pattern
Analysis and Machine Intelligence (PAMI), 25:5, pp
564-575.
Levi, K., Weiss, Y, 2004. Learning Object Detection from
a Small Number of Examples: The Importance of
Good Features. In IEEE Conf. on Computer Vision
and Pattern Recognition.
P. Viola and M. Jones, 2001. Rapid object detection using
a boosted cascade of simple features. In Proc. CVPR,
volume I, pages 511–518.
Papageorgiou, Oren and Poggio, 1998. A general
framework for object detection. In International
Conference on Computer Vision.
T. Ahonen, A. Hadid, and M. Pietikäinen, 2004. Face
Recognition with Local Binary Patterns. In Proc.
Eighth European Conf. Computer Vision, pp. 469-481.
T. Parag, F. Porikli, and A. Elgammal, 2008. Boosting
adaptive linear weak classifiers for online learning and
tracking. In Proc. CVPR.
K. Tieu and P. Viola, 2000. Boosting image retrieval. In
Proc. CVPR, pages 228–235.
Z. Kalal, J. Matas, and K. Mikolajczyk, 2010. P-N
Learning: Bootstrapping Binary Classifiers by
Structural Constraints. In Proc. CVPR.
Jakob Santner, Christian Leistner, Amir Sa_ari, Thomas
Pock, and Horst Bischof, 2010. Prost: Parallel robust
online simple tracking. In Computer Vision and
Pattern Recognition (CVPR), 2010 IEEE Conference
on, pages 723-730, 13-18.
B. Babenko, M.-H. Yang, and S. Belongie, 2009. Visual
Tracking with Online Multiple Instance Learning. In
CVPR.
Klein, Cremers, 2011. Boosting Scalable Gradient
Features for Adaptive Real-Time Tracking. In Int.
Conf. on Robotics and Automation (ICRA).
A. Adam, E. Rivlin, and I. Shimshoni, 2006. Robust
fragments based tracking using the integral histogram.
In CVPR.
R. Collins, Y. Liu, and M. Leordeanu, 2005. Online
selection of discriminative tracking features. In PAMI,
27(10):1631–1643.
M. Everingham, L. Van Gool, C. K. I. Williams, J. Winn,
and A. Zisserman, 2009. The Pascal Visual Object
Classes (VOC) Challenge. In Int. J. Comput. Vision,
88(2):303–308.
VISAPP2013-InternationalConferenceonComputerVisionTheoryandApplications
146