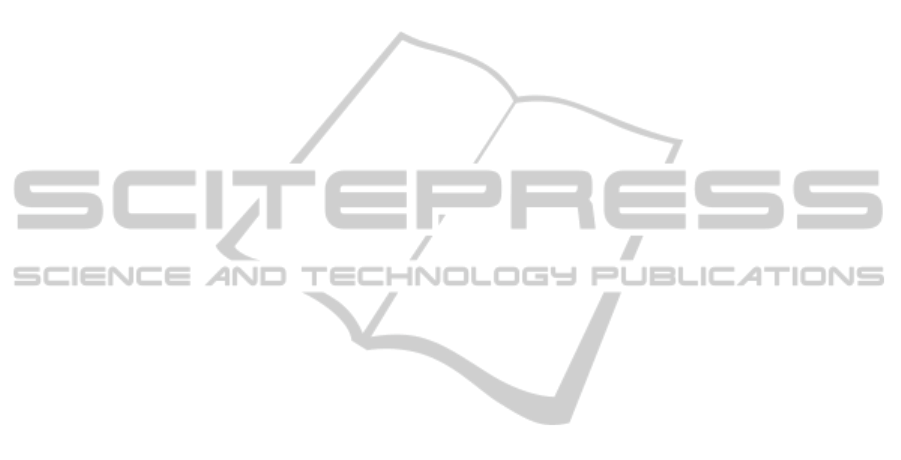
specificity and sensitivity were respectively, 98.43
and 97.28 in the first scenario. Further work should
focus on the extraction of more features from the
residual signal.
ACKNOWLEDGEMENTS
This work was supported by the ministry of higher
education (MOHE) of Malaysia under the
fundamental research grant scheme FRGS.
REFERENCES
Osowski, S., Linh, T. L., 2001. ECG beat recognition
using fuzzy hybrid neural network. IEEE Trans.
Biomed. Eng. 48 (11), 1265-1271.
De Chazal, P., Reilly, R. B.,2006. A Patient-Adapting
Heartbeat Classifier Using ECG Morphology and
Heartbeat Interval Features. IEEE Trans. Biomed. Eng.
53 (12), 2535-2543.
Hu,Y. H., Palreddy, S., Tompkins, W. J .,1997. A patient-
adaptable ECG beat classifier using a mixture of
experts approach. IEEE Trans. Biomed. Eng. 44 (9),
891-900.
Lagerholm, M., Peterson, C., Braccini, G., Edenbrandt, L.,
Sornmo,L.,2000. Clustering ECG complexes using
hermite functions and self-organizing maps. IEEE
Trans. Biomed. Eng. 47 (7), 338-348.
Sigg, D. C., Iaizzo, P. A., Xiao, Y F, He, B.,2010. Cardiac
Electrophysiology Methods and Models.: Springer.
Ge D., Srinivasan, N., Krishnan,S M.,2002. Cardiac
arrhythmia classification using autoregressive
modeling. Biomed Eng Online. 1 (5), 1-12.
Ham, F. M., Han, S.,1996. Classification of cardiac
arrhythmias using fuzzy ARTMAP. IEEE Trans.
Biomed. Eng. 43 (4), 425-430.
Lin, K. P., Chang, W. H.,1989. QRS feature extraction
using linear prediction. IEEE Trans. Biomed. Eng. 35
(10), 1050-055.
De Chazal, P., O’Dwyer, M., Reilly, R .,2004. Automatic
classification of heartbeats using ECG morphology
and heartbeat interval features. IEEE Trans. Biomed.
Eng. 51 (7), 1196-1206.
Lannoy, G. D., François, D., Delbeke, J.,Verleysen, M.,
2011. Weighted SVMs and Feature Relevance
Assessment in Supervised Heart Beat
Classification. Commun. Comput. Inf. Sci. 127, 212-
223.
Clifford, G D., Azuaje, F., McSharry, P., 2006. Advanced
Methods And Tools for ECG Data Analysis: Artech
House, Inc., Norwood.
Makhoul, J.,1975. Linear prediction: A tutorial
review. Proceedings of the IEEE. 63 (4), 561-580 .
Mark, R., Moody,G. (1997). MIT-BIHArrhythmia
Database. Available: http://ecg.mit.edu/dbinfo.html.
Last accessed june 2012.
Atal,B .,1989. A model of LPC excitation in terms of
eigenvectors of the autocorrelation of the impulse
response of the LPC filter. ICASSP. 1, 45-48.
Baali, H., Salami, M. J. E ., Akmeliawati, R., Aibinu, A M
. (2011). Analysis of the ECG Signal using SVD-
Based Parametric Modelling Technique. International
Symposium on Electronic Design, Test and
Application ., 180-184.
Baali, H., Akmeliawati, R., Salami, M. J. E., Aibinu, M.
A., Gani A. (2011). Transform Based Approach for
ECG Period Normalization. Computing in Cardiology.
38, 533-536.
Rifkin R. M.,2002. Everything Old Is New Again: A Fresh
Look at Historical Approaches to Machine
Learning. Phd Thesis, Massachusetts Institute of
Technology
.
Rifkin, R. M., Lippert, R. A. 2007. Notes on Regularized
Least Squares.Computer Science and Artificial
Intelligence Laboratory Technical Report. 1-8.
Bortolan, G., Jekova , I., Christov, I., 2005. Comparison of
Four Methods for Premature Ventricular Contraction
and Normal Beat Clustering.Computing in Cardiology.
31, 921-924.
Mai,V., Khalil, I.,2011. A Cardioid Based Technique to
Identify Premature Ventricular Contractions.
Computing in Cardiology. 38 , 673-676.
Shyu, L. Y., Wu, Y. H ., Hu, W.,2004. Using wavelet
transform and fuzzy neural network for VPC detection
from the Holter ECG. IEEE Trans. Biomed. Eng. 51
(7), 1269-1273.
BIOINFORMATICS2013-InternationalConferenceonBioinformaticsModels,MethodsandAlgorithms
170