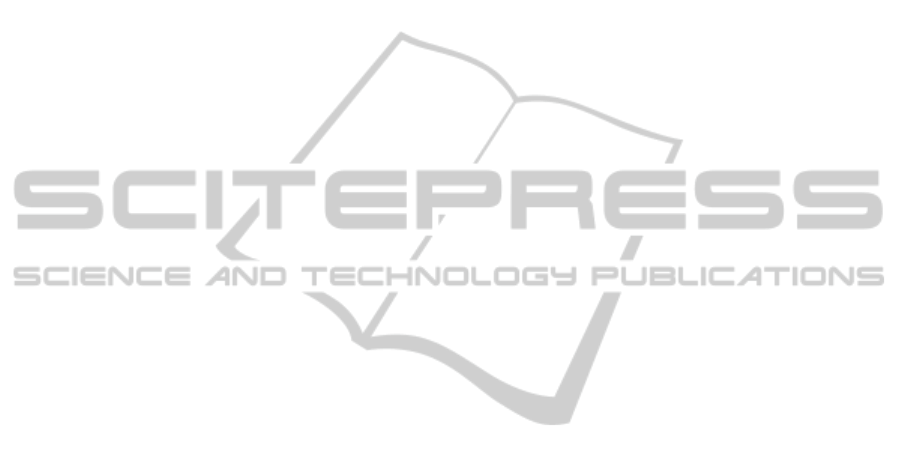
4 CONCLUSIONS; FUTURE
WORK
This paper has proposed a technique for dynamical
modeling of time-series with infrequent changes, and
has applied it to the study of human sleep data. The
technique, collective dynamical modeling and clus-
tering (CDMC), is based on adaptive pooling of data,
through iteration of clustering and dynamical mod-
eling steps. CDMC is a general algorithm that al-
lows a variety of probabilistic state space paradigms
(e.g., Markov chains, HMM, semi-Markov chains,
and HSMM) to be used as the dynamical models. Re-
sults over Markov mixture data show that the CDMC
algorithm converges rapidly, and that it successfully
identifies the statistical structure underlying the data
generation process. Preliminary results have been ob-
tained over human sleep data, using a compressed
data representation that captures the temporal order-
ing of stage transitions but not the stage bout dura-
tions. These results demonstrate convergence of the
CDMC algorithm over real clinical data, with good
cluster separation. The clusters found are shown to be
characterized by distinct sleep-dynamical properties.
Work in progress by the authors builds on the
present paper by including detailed stage bout timing
information, using semi-Markov chains as the spe-
cific dynamical models in the CDMC algorithm. In
future work, there will be a need to systematically as-
sess convergence, as well as clustering stability with
respect to initial parameter values. The effect on
convergence of alternative strategies for initialization
should also be examined. The applications to sleep
dynamics of the CDMC algorithm proposed in the
present paper should be explored in greater detail.
REFERENCES
Bianchi, M. T., Cash, S. S., Mietus, J., Peng, C.-K.,
and Thomas, R. (2010). Obstructive sleep apnea al-
ters sleep stage transition dynamics. PLoS ONE,
5(6):e11356.
Burns, J. W., Crofford, L. J., and Chervin, R. D. (2008).
Sleep stage dynamics in fibromyalgia patients and
controls. Sleep Medicine, 9(6):689–696.
Cadez, I., Gaffney, S., and Smyth, P. (2000). A general
probabilistic framework for clustering individuals. In
In Proceedings of the sixth ACM SIGKDD Interna-
tional Conference on Knowledge Discovery and Data
Mining, pages 140–149.
Cadez, I., Heckerman, D., Meek, C., Smyth, P., and White,
S. (2003). Model-based clustering and visualization of
navigation patterns on a web site. Data Min. Knowl.
Discov., 7:399–424.
Cavallero, C., Cicogna, P., Natale, V., Occhionero, M., and
Zito, A. (1992). Slow wave sleep dreaming. Sleep,
15(6):562–6.
Dempster, A. P., Laird, N. M., and Rubin, D. B. (1977).
Maximum likelihood from incomplete data via the
EM algorithm. Journal of the Royal Statistical So-
ciety, Series B, 39(1):1–38.
Iber, C., Ancoli-Israel, S., Chesson, A., and Quan, S.
(2007). The AASM Manual for the Scoring of Sleep
and Associated Events: Rules, Terminology, and Tech-
nical Specifications. American Academy of Sleep
Medicine, Westchester, Illinois, USA.
Liao, T. W. (2005). Clustering of time series data – a survey.
Pattern Recognition, 38(11):1857 – 1874.
Lo, C.-C., Amaral, L. A. N., Havlin, S., Ivanov, P. C., Pen-
zel, T., Peter, J.-H., and Stanley, H. E. (2002). Dynam-
ics of sleep-wake transitions during sleep. Europhys.
Lett., 57(5):625–631.
Moser, D., Anderer, P., Gruber, G., Parapatics, S., Loretz,
E., Boeck, M., Kloesch, G., Heller, E., Schmidt,
A., Danker-Hopfe, H., Saletu, B., Zeitlhofer, J., and
Dorffner, G. (2009). Sleep classification according to
AASM and Rechtschaffen & Kales: Effects on sleep
scoring parameters. Sleep, 32(2):139–149.
Rabiner, L. R. (1989). A tutorial on hidden markov models
and selected applications in speech recognition. Pro-
ceedings of the IEEE, 77(2):257–286.
Ramoni, M., Sebastiani, P., and Cohen, P. (2001). Bayesian
clustering by dynamics. Machine Learning, pages 1–
31.
Rand, W. M. (1971). Objective criteria for the evaluation of
clustering methods. Journal of the American Statisti-
cal Association, 66(336):846–850.
Rechtschaffen, A. and Kales, A., editors (1968). A Manual
of Standardized Terminology, Techniques, and Scor-
ing System for Sleep Stages of Human Subjects. US
Department of Health, Education, and Welfare Public
Health Service – NIH/NIND.
Sivriver, J., Habib, N., and Friedman, N. (2011). An
integrative clustering and modeling algorithm for
dynamical gene expression data. Bioinformatics,
27(13):i392–i400.
Smyth, P. (1997). Clustering sequences with hidden
Markov models. In Mozer, M. C., Jordan, M. I., and
Petsche, T., editors, Advances in Neural Information
Processing 9, pages 648–654. MIT Press.
Zhong, S. and Ghosh, J. (2003). A unified framework
for model-based clustering. J. Mach. Learn. Res.,
4:1001–1037.
BIOSIGNALS2013-InternationalConferenceonBio-inspiredSystemsandSignalProcessing
214