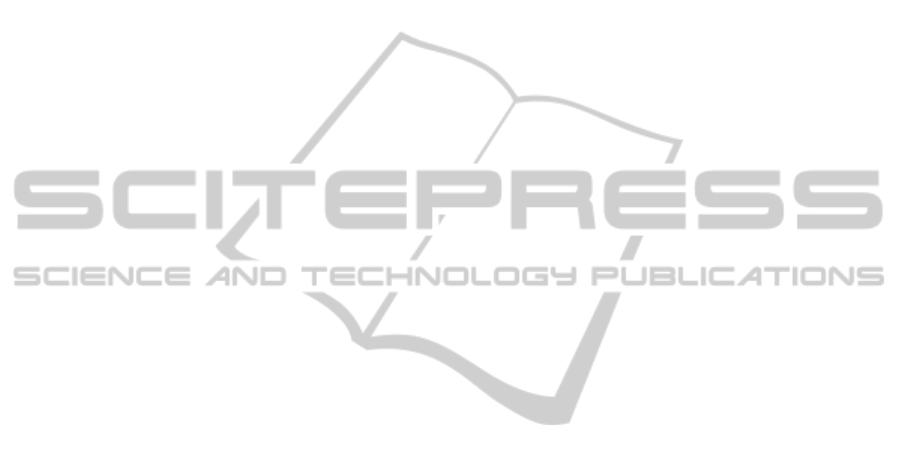
a proper selection of the most relevant features, out-
performs the best accuracy achievable with SVM on
the same dataset (II) and even with those obtained by
SVM on dataset III, specifically optimized for that
technique with a two-step FS process. Therefore,
our experiments shows the capabilities of SDA to de-
scribe in a more precise way the underlying distribu-
tions of each of the staining pattern class, improving
their classification accuracies.
5 CONCLUSIONS
In this paper we proposed the comparison of two ap-
proaches, based on SVM and SDA, for the automatic
classification of staining patterns in HEp-2 cell IIF
images. Texture descriptors based on GLCM and
DCT coefficients are first exploited to extract a 372-
size characteristic vector for each cell. Then, a fea-
ture selection algorithm is applied to obtain a reduced
candidate feature set that improves the classification
accuracies of the two methods.
Feature selection is based on the mRMR algo-
rithm, which sorts the features that are most rele-
vant for characterizing the classification variable. The
50 top-ranked features were selected. In the case
of SVM-based method, a two-steps feature selection
procedure, coupling mRMR with SFS algorithm, is
implemented in order to further improve classification
accuracies.
The two approaches provide average classifica-
tion accuracies of about 87% and 91%, respectively.
These results are comparable with those of human
specialists. Conversely, they are completely repeat-
able since our automated technique does not depend
on the subjectivity of the operator. Moreover, our ex-
periments show the effectiveness of SDA into describ-
ing more precisely, compared to SVM, the underlying
distributions of each of the staining pattern class.
As future steps, we plan to work on:
1) a better characterization of cell patterns, which
can be insensitive to changes in size, rotation and in-
tensity;
2) an improvement of the SDA classifier in terms
of computational efficiency. For this purpose, meth-
ods selecting a priori the classes that effectively needs
to be partitioned, like the one described in (Sang-
Woon Kim, 2010), will be investigated;
Moreover, we plan to develop a pipeline for auto-
matic cells segmentation in IIF images and to com-
bine it with our pattern classification algorithm in or-
der to obtain a complete automated approach for the
computer-aided diagnosis (CAD) of autoimmune dis-
eases.
REFERENCES
Ahmed, N. and Natarajan, T. and Rao, K. R. Discrete
Cosine Transform. IEEE Trans. Computers, 90–93,
1974.
Belhumeur, P. N. and Hespanha, J. P. and Kriegman, D. J.
Eigenfaces vs. Fisherfaces: Recognition Using Class
Specific Linear Projection. IEEE Trans. Pattern Anal-
ysis and Machine Intelligence, vol. 19, no. 7, pp. 711-
720, July 1997.
Boulgouris, N. V. and Plataniotis, K. N. and Micheli-
Tzanakou, E. Discriminant Analysis for Dimensional-
ity Reduction: An Overview of Recent Developments.
In Biometrics: Theory, Methods, and Applications,
Wiley, 2009
Chang , C.-C. and Lin, C.-J. Libsvm: A library for support
vector machines. ACM Trans. Intell. Syst. Technol.,
2(3):27:1–27:27, May 2011.
Clausi, D. A., An analysis of co-occurrence texture statis-
tics as a function of grey level quantization. Can. J.
Remote Sensing 28(1):45–62, 2002.
Creemers, C. and Guerti, K. and Geerts, S. and Van Cot-
them, K. and Ledda, A. and Spruyt, V. HEp-2 cell
pattern segmentation for the support of autoimmune
disease diagnosis. ISABEL 2011, Proc. of, 28:1–5,
2011.
Di Cataldo, S. and Bottino, A. and Ficarra, E. and Macii,
E. Applying Textural Features to the Classification
of HEp-2 Cell Patterns in IIF images. 21st Inter-
national Conference on Pattern Recognition (ICPR
2012), Tsukuba, Japan, November 11-15, 2012.
Egerer, K. and Roggenbuck, D. and Hiemann, R. and
Weyer, M. G. and Buttner, T. and Radau, B. and
Krause, R. and Lehmann, B. and Feist, E. and
Burmester, G. R. Automated evaluation of autoan-
tibodies on human epithelial-2 cells as an approach
to standardize cell-based immunofluorescence tests.
Arthritis Research & Therapy, 12(2):1–9, 2010
Etemad, K. and Chellapa, R. Discriminant Analysis for
Recognition of Human Face Images. J. Optical Soc.
Am. A, vol. 14, no. 8, pp. 1724-1733, 1997.
Fukunaga, K. Introduction to Statistical Pattern Recogni-
tion. second ed. Academic Press, 1990.
Gkalelis, N. and Mezaris, V. and Kompatsiaris, I. Mixture
subclass discriminant analysis IEEE Signal Process-
ing Letters, vol. 18, no. 5, pp. 319-322, May 2011.
Haralick, R. M. and Shanmugam, K. and Dinstein, I.. Tex-
tural features for image classification. Systems, Man
and Cybernetics, IEEE Transactions on, 3(6):610–
621, nov. 1973.
Hsieh, R. Y. and Huang, Y. C. and Chung, C. W. and Huang,
Y. L. HEp-2 Cell Classification in Indirect Immuno-
fuorescence Images. ICICS 2009, Proc. of, 26:211–
214, 2009.
Kurgan, L. A. and Cios, K. J. Caim discretization algorithm.
IEEE Trans. on Knowl. and Data Eng., 16(2):145–
153, Feb. 2004.
Martinez, A. M. and Zhu, M. Where Are Linear Feature
Extraction Methods Applicable? IEEE Trans. Pattern
BIOINFORMATICS2013-InternationalConferenceonBioinformaticsModels,MethodsandAlgorithms
60