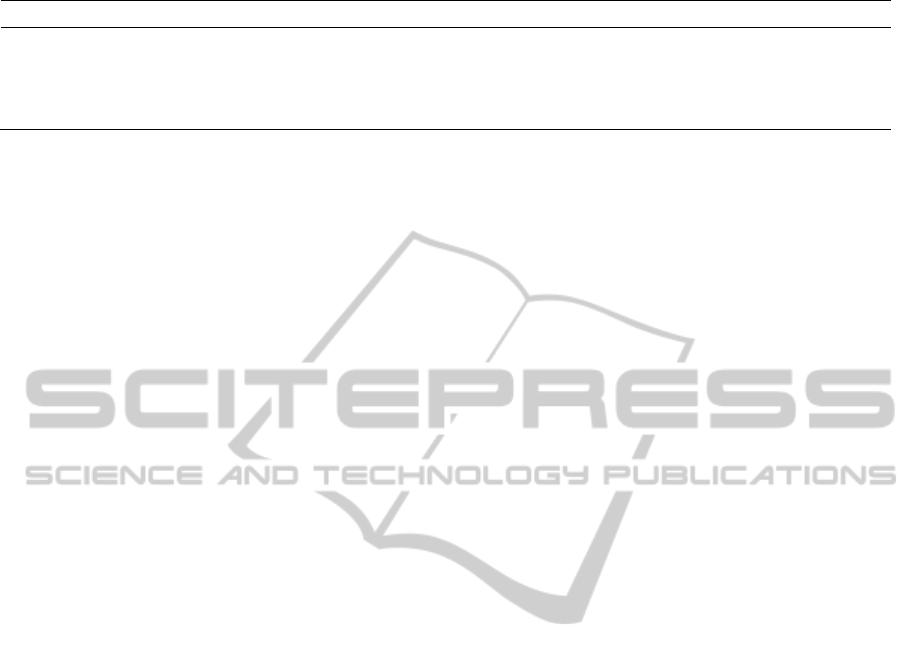
Table 3: Bias-corrected predictive performance for five different models.
MI Final Model* MI Clinical Model* C-C Final Model C-C Clinical Model C-C Split Models*
C-index 0.744 [0.742-0.744] 0.734 [0.733-0.738] 0.747 0.731 0.728 [0.683-0.753]
AUC 0.748 [0.747-0.749] 0.738 [0.736-0.741] 0.749 0.732 0.732 [0.678-0.765]
Slope 0.961 [0.954-0.966] 0.981 [0.976-0.992] 0.949 0.975 0.956 [0.67-1.209]
NRI 14.6% [10%-17.9%] / 2.47% / /
* Data are expressed as median [full range].
4 DISCUSSION
In this study, we have given an example to illustrate
the process of prediction model development based
on incomplete data. To get a more stable risk factor
set from clinical and genetic variable list for CHD in
T2DM, we integrated bootstrap and backward varia-
ble selection on imputed data sets.
Incomplete data are commonly encountered in
medical research. Excluding all patients with any
missing values may lose useful information and re-
duce the power of prediction model, which leads to
some variables not attaining statistical significance,
such as for the systolic BP and rs4607106 in our MI
Final Model and C-C Final Model. In our study, the
MI models are very similar to the C-C models, it is
because the missing rates are not high and the sam-
ple sizes are close, but imputation makes it more
powerful to perform variable selection.
Combining bootstrap resampling with variable
selection will be benefit to the stability of selected
variables. Through bootstrap and variable selection,
variables with strong effects on the outcome will be
selected more frequently than those with no or weak
effects. To validate a model, data-splitting as a sim-
ple method is commonly used, but the model per-
formance will vary greatly with different splits, and
bias will be introduced. Our results showed the boot-
strapping bias-corrected indicators of performance
were close to the median indicators produced by
multiple times training/test splits. Therefore, to en-
sure an honest model evaluation, we would better
evaluate the models by generating multiple pairs of
training/test sets or use bias-corrected method.
Importantly, three SNPs (rs2568958, rs7754840
and rs4607103 located at NEGR1, CDKAL1 and
ADAMTS9 gene, respectively) were selected with
high inclusion frequencies and the NRI results indi-
cated they contributed to the CHD prediction. There-
fore, these three T2D-related SNPs may also have
association effects with CHD. To validate the effect
of these SNPs, we will try to do some further anal-
yses, such as replication study.
In conclusion, this cohort study illustrated the
MICE and bootstrap can be benefit to the develop-
ment of prediction model based on dataset contain-
ing clinical and genetic variables. An informative
risk factor set for CHD, including three T2D-related
SNPs, was successfully identified from CHD pro-
spective cohort of Hong Kong Chinese patients with
T2DM. Future research will be needed to validate
the effect of these selected SNPs.
ACKNOWLEDGEMENTS
This work was supported by the Innovation and
Technology Fund (ITS/487/09FP), RGC Central
Allocation Scheme (CUHK 1/04C), RGC Ear-
marked Research Grant (CUHK4724/07M), and the
CUHK Direct Grant (2150476 and 2141611).
REFERENCES
Harrel Jr, F. E. a. L., K. L. and Mark, D. B. 1996. Tutorial
in biostatistics: multivariable prognostic models:
issues in developing models, evaluating assumptions
and adequacy, and measuring and reducing error.
Statistics in Medicine, 361–387.
Laakso, M. 2001. Cardiovascular disease in type 2
diabetes: challenge for treatment and prevention. J
Intern Med, 249, 225-35.
Vaarhorst, A. A., Lu, Y., Heijmans, B. T., Dolle, M. E.,
Bohringer, S., Putter, H., Imholz, S., Merry, A. H., van
Greevenbroek, M. M., Jukema, J. W., Gorgels, A. P.,
van den Brandt, P. A., Muller, M., Schouten, L. J.,
Feskens, E. J., Boer, J. M. & Slagboom, P. E. 2012.
Literature-based genetic risk scores for coronary heart
disease: the Cardiovascular Registry Maastricht
(CAREMA) prospective cohort study. Circ
Cardiovasc Genet, 5, 202-9.
van Buuren, S., Boshuizen, H. C. & Knook, D. L. 1999.
Multiple imputation of missing blood pressure
covariates in survival analysis. Stat Med, 18, 681-94.
Yang, X., So, W. Y., Kong, A. P., Ma, R. C., Ko, G. T.,
Ho, C. S., Lam, C. W., Cockram, C. S., Chan, J. C. &
Tong, P. C. 2008. Development and validation of a
total coronary heart disease risk score in type 2
diabetes mellitus. Am J Cardiol, 101, 596-601.
DevelopmentofPredictionModelsunderMultipleImputationforCoronaryHeartDiseaseinType2DiabetesMellitus
315