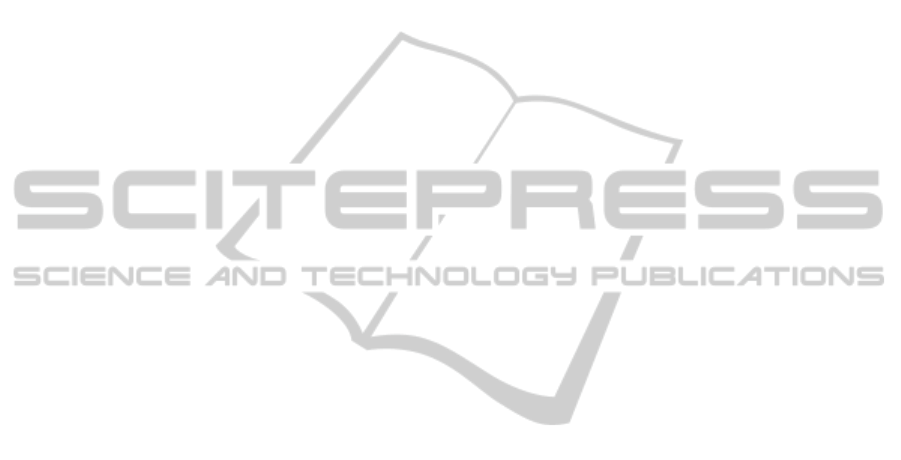
example, in the case of the QRS complex region,
this bandwidth ranges from around 5 to 40 Hz. In
experiments which involve removal of gradient
artefacts residuals from EEG signals, SSD shows to
select higher frequency components associated to
the artefact (Ritter et al., 2010; Ferreira et al. 2012).
Thereby, SSD could be evaluated and proposed as
an alternative time-domain filtering approach.
6 CONCLUSIONS
In this work, we propose a methodology (SSD) for
detection of sharp wave activity in biological signals
based upon differentiation of consecutive samples of
the digital signal.
Our methodology shows to achieve effective
identification of the sharp wave activity associated
to the QRS samples in the ECG signal. Also
evaluation of a QRS detector prototype which makes
use of SSD reveals that the QRS complexes are
localized with sensitivity and positive predictivity
comparable to other methodologies proposed in the
literature. In future work, our methodology shall be
applied and evaluated for detection of sharp wave
activity in other types of biological signals.
ACKNOWLEDGEMENTS
This work has been made possible by a grant from
the European Union and Erasmus Mundus – EBW II
Project, and by a grant from CNPq – Science
without Borders Program.
REFERENCES
Allen, P., Polizzi, G., Krakow, K., Fish, D., Lemieux, L.,
1998. Identification of EEG events in the MR scanner:
the problem of pulse artefact and a method for its
subtraction. NeuroImage. 8, 229-239.
ANSI/AAMI/ISO EC57, 1998. Testing and reporting
performance results of cardiac rhythm and ST segment
measurement algorithms. AAMI Recommended
Practice/ American National Standard.
Arzeno, N., Deng, Z., Poon, C., 2008. Analysis of first-
derivative based QRS detection algorithms. IEEE
Trans. Biomed. Eng. 55 (2), 478-484.
Benitez, D., Gaydecki, P., Zaidi, A., Fitzpatrick, A., 2000.
A new QRS detection algorithm based on the Hilbert
transform. Comput. Cardiol. 27, 379-382.
Clifford, G., 2006. ECG statistics, noise, artifacts, and
missing data. In G. Clifford, F. Azuaje, P. McSharry,
(eds.), Advanced tools for ECG data analysis. Artech
House: Boston, London.
Cluitmans, P., Jansen, J., Beneken, J., 1993. Artefact
detection and removal during auditory evoked
potential monitoring. J. Clin. Monit. 9 (2), 112–120.
Ferreira, J., Cluitmans, P., Aarts, R. M., 2012. Gradient
artefact correction in the EEG signal recorded within
the fMRI scanner. Proceedings of the 5
th
International
Conference on Bio-inspired Systems and Signal
Processing, BIOSIGNALS 2012, Vilamoura, Portugal,
February 1 – 4, 2012. 110-117.
Hamilton, P., Tompkins, W., 1986. Quantitative
investigation of QRS detection rules using the
MIT/BIH Arrhythmia Database. IEEE Trans. Biomed.
Eng. 33 (12), 1157-1165.
Köhler, B., Hennig, C., Orglmeister, R., 2002. The
principles of software QRS detection. IEEE Eng. Med.
Biol. Mag. 21 (1), 42-57.
MIT-BIH, 1998. Database Distribution. Massachusetts
Institute of Technology, Cambridge, MA. Available:
http://ecg.mit.edu/.
Pan, J., Tompkins, W., 1985. A real-time QRS detection
algorithm. IEEE Trans. Biomed. Eng. 32 (3), 230-236.
Rangayyan, R., 2002. Biomedical signal analysis: a case-
study approach. Wiley: New York.
Rezk, S., Join, C., Asmi, S., 2011. An algebraic
derivative-based method for R wave detection.
Proceedings of the 19
th
European Signal Processing
Conference, EUSIPCO 2011, Barcelona, Spain,
August 29 – September 2, 2011. 1578-1582.
Ritter, P., Becker, R., Freyer, F., Villringer, A., 2010. EEG
quality: the image acquisition artifact. In C. Mulert, L.
Limieux (eds.), EEG-fMRI: Physiological basis,
technique and applications. Springer: Verlag, Berlin,
Heidelberg.
Thakor, N., Webster, J., Tompkins, W., 1984. Estimation
of QRS complex power spectra for design a QRS
filter. IEEE Trans. Biomed. Eng. 31 (11), 702-706.
Van de Velde, R., Van Erp, G., Cluitmans, P., 1998.
Detection of muscle artefact in the normal human
awake EEG. Electroencephalogr. Clin. Neurophysiol.
107, 149-158.
BIOSIGNALS2013-InternationalConferenceonBio-inspiredSystemsandSignalProcessing
332