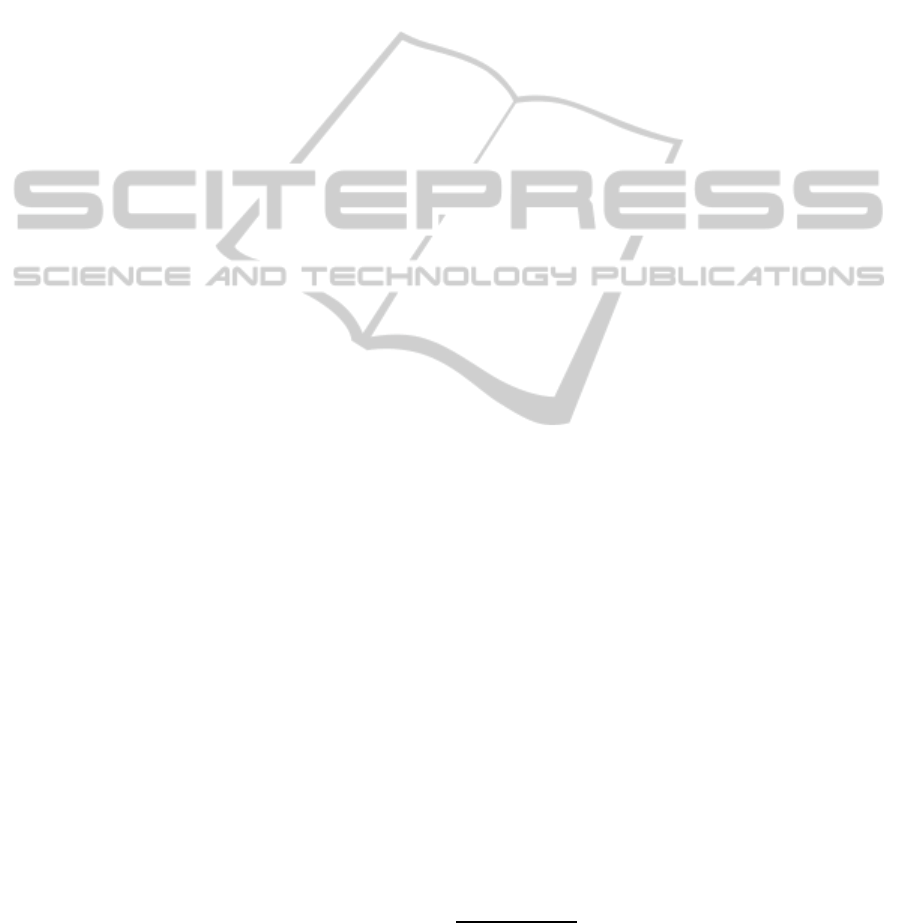
era components out. Next, the consequences of the
template ageing should be distinguished from effects
related to inter- and intra-session matching scores.
Intra-session comparison scores will typically exhibit
a better match among images when compared to the
corresponding inter-session results. However, the
inter-session changes in imaging conditions, e.g., en-
vironmental parameters or subject’s interaction with
the equipment, usually blur more subtle ageing ef-
fects related to the eye biology. From the technolog-
ical point of view, the iris biometric features, not im-
ages, are used to finally judge on the extent to which
the ageing occurs, as we are interested in how this
phenomenon transforms from the image space (pos-
sible to inspect visually) to the feature space (natural
for biometric matching). However, the transforma-
tion between these spaces is always proprietary to an
iris coding method, and the strength of the template
ageing effect may depend on the feature extraction
methodology. Last but not least, we have no guar-
antee that the ageing effect will be evenly observed
across the subjects of different populations. Exper-
iment results obtained with a particular database of
images may be a weak predictor of this phenomenon
for people of different race, health or dietary culture.
The expected stability of the iris pattern may also
be regarded (in a broader sense) as a demand for sta-
tionarity. Stationary time series is characterized by
temporal stability of its statistical properties. How-
ever, stability of one property (e.g., the average value)
does not guarantee the stationarity, as other proper-
ties (e.g., sample variance) may still vary over time.
This makes the research of the ageing phenomenon
even more complicated, as the judgment should not
be based solely on properties of a single statistics (e.g.
monotonic behavior of the average matching score).
Above aspects related to discovering the truth
about the iris ageing urgently call for experiments car-
ried out for different populations across the world,
performed in different environments, for as many fea-
ture extraction methods as possible and for the longest
possible time lapse between measurements. Answer-
ing this call, we present the iris ageing analysis based
on comparison results obtained for three iris match-
ers and the biometric samples captured even eight
years apart. To our best knowledge, eight years is the
longest time interval characterizing samples used in
the iris ageing analysis up to date worldwide.
2 RELATED WORK
Iris recognition is relatively young discipline, and
thus there is still a shortage of databases of iris images
collected with adequate time intervals to observe the
template ageing phenomenon. This is why the litera-
ture devoted to the iris template ageing is still limited.
Gonzalez et al. first addressed a possibility of in-
fluence of the time lapse onto iris recognition accu-
racy, and presented own experimental study (Tome-
Gonzalez et al., 2008). Theyestimated coding method
parameters using a part of the multimodal BioSec
database, containing samples of 200 subjects. Final
results were generated with the use of the BioSe-
curID database containing iris images captured for
more than 250 volunteers. Although the databases
used were reasonably large, the time lapse between
image captures in the test database was very short
(one to four weeks). As the observation of the age-
ing effects in such a short time period may be diffi-
cult, the authors focused on inter- vs. intra-session
recognition accuracy analysis. According to the ex-
pectations, the genuine intra-session comparisons re-
vealed a better match (e.g. FNMR∈ (0.085, 0.113)
@FMR=0.01)
1
when compared to the inter-session
results (e.g. FNMR∈ (0.224, 0.258) @FMR=0.01).
No significant differences in comparison scores can
be observed for inter-session results with respect to
these very short time intervals. Thus any conclusions
on the iris ageing cannot be drawn based on this work,
yet it supports the intuition related to the importance
of the enrollment procedure that should produce the
enrollment samples predicting, to the maximum pos-
sible extent, the inter-session variations.
The intra- vs. inter-session variations in iris
matching scores were also studied by Rankin et al.,
who used a database of images captured for 238 sub-
jects (Rankin et al., 2012). The sessions were sepa-
rated by three and six months time periods. Results
are presented separately for irises grouped in classes
depending on the iris texture density, and support a
claim on the increase of false rejections when time
interval between samples increases. However, this
study lies slightly next to the main course of biomet-
rics technology, as the images were captured in visi-
ble, not near-infrared light and by a specialized biomi-
croscope, not typically used in iris recognition.
Baker et al. presents the first known to us analy-
sis of the iris ageing under long, four-year time lapse
(Baker et al., 2009). A small database consisted of im-
ages captured for 13 volunteers was used in the anal-
ysis with the iris segmentation inspected manually
(this excludes the segmentation errors from the source
1
FNMR (False Non-Match Rate) is an empirical esti-
mate of the recognition method error relying on falsely re-
jecting a genuine sample; FMR (False Match Rate) is an
empirical estimate of the recognition method error relying
on falsely accepting an impostor sample.
TemplateAgeinginIrisRecognition
71