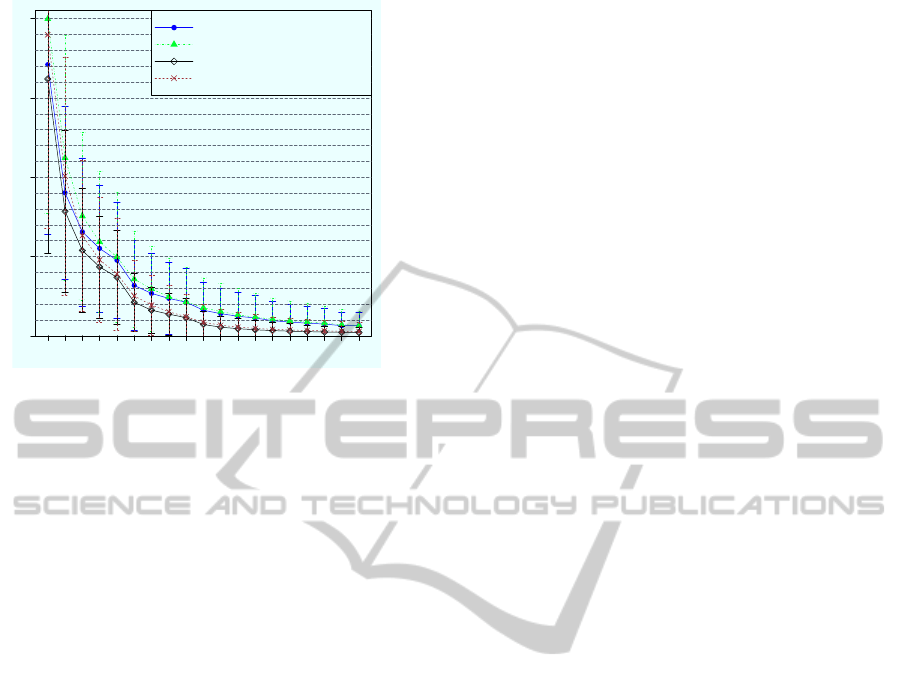
2 3 4 5 6 7 8 9 10 11 12 13 14 15 16 17 18 19 202 3 4 5 6 7 8 9 10 11 12 13 14 15 16 17 18 19 20
Number of Hermite Polynomials
SeekBothChannels
OriginalAnnotations
SeekBothChannelsFiltered
OriginalAnnotationsFiltered
Figure 2: (Lagerholm et al., 2000) error measure.
why more improvement is obtained for a low number
of polynomials is because when using a high number
of polynomials it is posible to represent the beat ac-
curately even if the point chosen as the center of the
fitting window is not the point of maximum symmetry
(see Figure 1).
Filtering provides significant improvements in the
results (see Figure 1 and Figure 2). We have per-
formed independent tests using only high frequency
filtering and only baseline drift removal. The removal
of baseline drift alone produced virtually identical re-
sults to working directly with the unfiltered signal; al-
most all the improvements that can be seen in Figures
1 and 2 when using the filtered signal arise from the
high frequency filtering. This suggests that Hermite
approximation is more affected by high frequency
noise than by baseline drift. For example, a 2% of
NRMSE can be achieved without filtering with 11
polynomials but with filtering only 8 are required; and
we cannot reach a 1% of NRMSE without filtering,
not even with 20 polynomials, while with filtering is
possible to reach this error with 13 (see Figure 1).
Among the papers we have reviewed only (Lager-
holm et al., 2000) reports error results for the Hermite
approximation. Lagerholm et al. calculated the error
with Equation 2. They only report the values for 3,
4, 5 and 6 Hermite polynomials; the errors are 9.7%,
6.8% , 5.5% and 4.5%, respectively. These results are
slightly lower than the results we obtained with our
beat correction algorithm applied over both channels
with the unfiltered signal. However, when using the
filtered signal the errors we obtain are lower than the
results of Lagerholm et al., both when using the orig-
inal beat annotations from the database, and when us-
ing the beat position correction over both channels.
It should be noted that Lagerholm et al. applied no
high-frequency filtering.
In this paper we have determined the accuracy of
the representation with a measure of the error between
the reconstruction obtained from the Hermite polyno-
mials and the original signal. However, if the final
goal of representing beats with Hermite polynomials
is to classify them in different morphological fami-
lies (instead of, for example, compression of the ECG
(Jane et al., 1993)), the features that minimize this
error need not to be those that provide the best sepa-
ration between the different classes of beats. It would
be interesting to study how the features obtained when
representing the beats with a different number of Her-
mite polynomials enable the different beat families to
be separated by an automatic classifier. This will be
one of our lines of future work.
ACKNOWLEDGEMENTS
This work was supported by the Spanish Ministry
of Science and Innovation (MICINN) under grant
TIN2009-14372-C03-03.
REFERENCES
Braccini, G. and Edenbrandt, L. (1997). Self-organizing
maps and Hermite functions for classification of ECG
complexes. in Cardiology 1997, 24:425–428.
De Chazal, P. and Reilly, R. B. (2006). A patient-adapting
heartbeat classifier using ECG morphology and heart-
beat interval features. IEEE Transactions on Biomed-
ical Engineering, 53(12 Pt 1):2535–43.
Hu, Y., Tompkins, W., and Urrusti, J. (1993). Applica-
tions of artificial neural networks for ECG signal de-
tection and classification. Journal of Electrocardiol-
ogy, 26:66–73.
Jane, R., Olmos, S., and Laguna, P. (1993). Adaptive Her-
mite models for ECG data compression: performance
and evaluation with automatic wave detection. Com-
puters in Cardiology.
Lagerholm, M., Peterson, C., Braccini, G., Edenbrandt,
L., and S
¨
ornmo, L. (2000). Clustering ECG com-
plexes using hermite functions and self-organizing
maps. IEEE Transactions on Biomedical Engineer-
ing, 47(7):838–48.
Moody, G. and Mark, R. (2001). The impact of the mit-
bih arrhythmia database. Engineering in Medicine and
Biology Magazine, IEEE, 20(3):45 –50.
Park, K., Cho, B., Lee, D., Song, S., Lee, J., Chee, Y., Kim,
I., and Kim, S. (2008). Hierarchical support vector
machine based heartbeat classification using higher
order statistics and hermite basis function. In 2008
Computers in Cardiology, pages 229–232. IEEE.
OntheAccuracyofRepresentingHeartbeatswithHermiteBasisFunctions
341