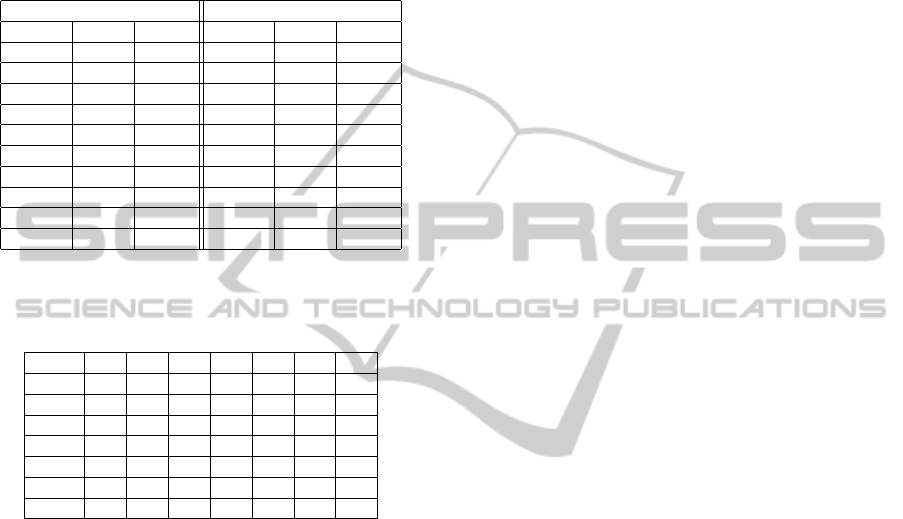
Table 1: Left: Most frequently selected features over all
cross-validation folds. Table shows the selection frequency
in percent for features derived from mean heart rate (MHR),
variance of heart rate (VHR), mean EDA (EDA) and num-
ber of startles (STA): Peak Width (PW), Relative Peak In-
dex (RPI), Average (AVG), Standard Deviation (SD), Max-
imum (Max), Minimum (Min). Right: Same information
for feature selection without peak width, peak position and
centroid.
full feature set restricted feature set
Signal Feat. Freq. Signal Feat. Freq.
MHR PW 53.3 EDA SD 100.0
EDA PW 46.7 MHR Avg 73.3
MHR SD 33.3 MHR SD 73.3
MHR Avg 13.3 MSC Avg 66.7
MHR Min 13.3 VHR Min 40.0
STA PW 13.3 EDA Min 33.3
STA SD 6.7 STA Avg 33.3
VHR RPI 6.7 MHR Max 26.7
MSC Max 26.7
VHR Avg 26.7
Table 2: Average accuracies in percent for pairwise classi-
fication of windows from different minutes (1 = 0s to 60s, 2
= 60s to 120s, . . . ) of the film AKUMI.
Min. 2 3 4 5 6 7 8
1 61 54 67 64 75 70 56
2 52 58 59 72 70 67
3 64 63 77 73 63
4 58 72 70 66
5 70 67 61
6 59 67
7 58
tive power of both modalities separately, we see sim-
ilar recognition rates if we restrict the feature set to
only EDA-based features (97.8%) or only PPG-based
features (96.67%). We conclude that both modalities
carry information on the arousal profile and depend-
ing on the application it may be possible to reduce the
number of required sensors.
Note that some of the employed features (e.g. rel-
ative centroid position) encode information specific
to the dramaturgy of the films. Therefore, the trained
model will not be applicable to different films without
loss of recognition accuracy (albeit, films of similar
dramaturgical structure could work). We therefore re-
peat evaluation after removing the features encoding
relative peak position, relative centroid position and
peak width. As expected, the accuracy drops signifi-
cantly to 73.3%. The merit of this model is that it still
provides reasonable recognition accuracy using much
more generic features which promise generalizability
to different films. The selected features are given in
the right part of Table 1. Again, we identify a number
of features which is repeatedly selected across folds.
As documented in Section 3, significant differ-
ences cannot only be noted between different films
but also during the course of one film. To investigate
the possibility of identifying different parts of the film
based on biosignals, we classify the window-based
features extracted for the process described above for
the movie AKUMI. To each window, we assign a la-
bel based on its position within the film, using one
label for each full minute. Classification is performed
pairwise for each combination of two labels to investi-
gate similarity effects. In this setup, we do not expect
high classification accuracy for each pair of segments.
Instead, we can interprete the recognition accuracy as
a measure of distance between two segments based
on the arousal profile. Table 2 presents the results of
leave-one-person-out cross-validation. As expected,
performance reaches levels of up to 77% for sections
which are dramaturgically very different. For sections
which are similar in this regard (e.g. both from the
fast-paced ending), accuracy drops. This result is in
strong accordance with the observations on Figure 1
and shows that, even given the difficulty induced by
fuzzy class transitions, automatic affective profiling
of a film is possible.
REFERENCES
Bradley, M. and Lang, P. (1994). Measuring emotion: the
self-assessment manikin and the semantic differential.
Journal of behavior therapy and experimental psychi-
atry, 25(1):49–59.
Ekman, P., Friesen, W., and Simons, R. (1985). Is the star-
tle reaction an emotion?. Journal of Personality and
Social Psychology, 49(5):1416.
Lichtenstein, A., Oehme, A., Kupschick, S., and Jrgensohn,
T. (2008). Comparing two emotion models for deriv-
ing affective states from physiological data. In Pe-
ter, C. and Beale, R., editors, Affect and Emotion in
Human-Computer Interaction, volume 4868 of LNCS,
pages 35–50. Springer Berlin / Heidelberg.
Picard, R. (2000). Affective computing. The MIT press.
Picard, R., Vyzas, E., and Healey, J. (2001). Toward ma-
chine emotional intelligence: Analysis of affective
physiological state. Transactions on pattern analysis
and machine intelligence, pages 1175–1191.
Soleymani, M., Chanel, G., Kierkels, J. J. M., and Pun,
T. (2008). Affective characterization of movie scenes
based on multimedia content analysis and user’s phys-
iological emotional responses. Multimedia, Interna-
tional Symposium on, 0:228–235.
BIOSIGNALS2013-InternationalConferenceonBio-inspiredSystemsandSignalProcessing
350