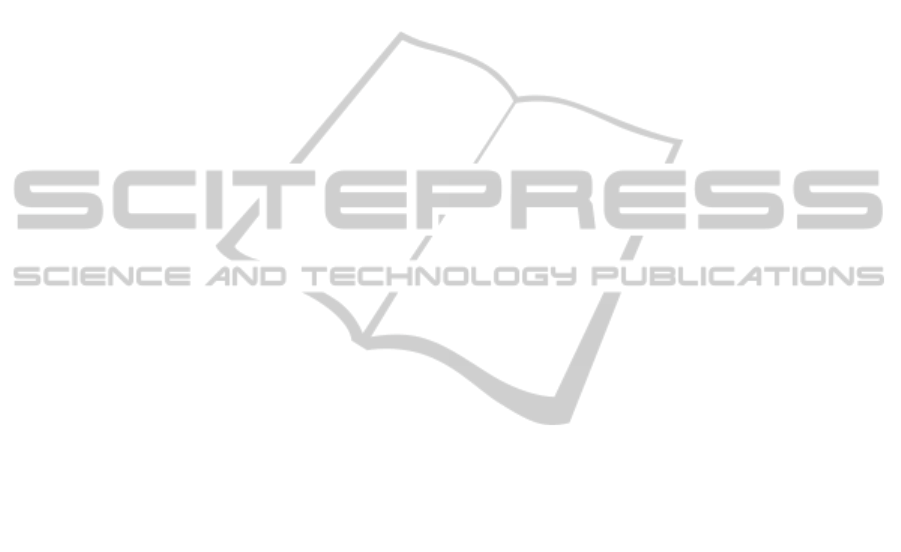
On the other hand, for the IEigHB method, both the
mean and SD increase with lower energy values.
4.7 K- Nearest Neighbours (K-NN)
As depicted in Figure 6, the number of K-Nearest
Neighbors used in the decision method does not seem
to influence significantly the error probability’s
mean. However, there is a general tendency that for
lower energys and low values of k, the better results
are obtained by increasing the KNN. This indicates
that when the data is poorer (less segments, less
energy) it is advisable to use a higher number of
neighbours in the decision process in order to lower
the identification error.
5 CONCLUSION
In this paper we presented a framework and
methodology for user identification from ECG
signals exploring PCA and K-NN classification,
combined with outlier removal. Two main
approaches were proposed, either using eigen-
heartbeats that model the overall population, or using
individualized eigen-heartbeats per user. Overall,
both methods have the potential of successfully
identifying individuals using their finger/hands ECG
signal. Using 30 heartbeat segments as templates,
and 10 segments for accessing the system, both
methods lead to a 0% identification error. However,
the OEigHB method has shown, in general, lower
sensitivity to the design parameters, presenting the
lowest error values. Emotional and pathological
states may create intra subject variability through
time, lowering the accuracies obtained. This topic
has not been thoroughly studied in the context of
biometrics; however some work is already trying to
account for these factors (Agrafioti, 2011).
The down side of ECG-based biometric methods
is that longer enrolment and identification time is
needed to achieve better accuracies (around 30 sec.
of enrollment and 10 sec. of access time for a 0%
error). However, this biometric modality is less
prone to forging than more conventional modalities,
such as the fingerprint, and can verify aliveness and
stress level, which can be useful to prevent
unwillingly identification. Ongoing work includes a
further enlargement of the database, and extending
this study to situations of multiple acquisitions at
distinct time instants.
ACKNOWLEDGEMENTS
This work was partially funded by Fundação para a
Ciência e Tecnologia (FCT) under grants SFRH/
BD/65248/2009 and SFRH/PROTEC/49512/2009.
REFERENCES
Agrafioti, Foteini (2011). PhD thesis. Univ. of Toronto.
Biel, L.; Pettersson, O.; Philipson, L. and Wide, P. (2001)
ECG analysis – a new approach in human
identification. IEEE Transactions on Instrumentation
and Measurement, 50(3):808–812.
Chan, A.; Hamdy, M.; Badre, A. and Badee, V. (2008).
Wavelet distance measure for person identification
using electrocardiograms. IEEE Transactions on
Instrumentation and Measurement, 57(2):248–253,
Engelse, W. A. H.; and Zeelenberg, C. (1979). “A single
scan algorithm for QRS-detection and feature
extraction,” Comp.in Card., vol. 6, pp. 37–42.
Irvine, J.; A., S. (2009). eigenPulse: Robust Human
Identification from Cardiovascular Function. The
Draper Technology Digest , pp. 50-59.
Israel, S., M., J. (2010). The Heartbeat: the Living
Biometric. Chapter in Biometrics: theory, Methods and
Applications, pp. 429-459. Wiley
Jain, A. and Flynn, P. and Ross, A. (2008). Handbook of
Biometrics. Springer.
Lourenço, A.; Silva, H.; Fred, A. (2011). Unveiling the
Biometric Potential of Finger-Based ECG Signals.
Computational Intelligence and Neuroscience
Lourenço, A.; Silva, H.; Leite, P.; Lourenco, R., and Fred,
A. (2011). “Real time electrocardiogram segmentation
for finger based ECG biometric,” in Proc. of
BIOSIGNALS 2012, pp. 49–54.
Silva, H., Fred, A. L., and Lourenço, A. (2011). Check
Your Biosignals Here: Experiments on Affective
Computing and Behavioral Biometrics. RecPad.
Silva, H.; Gamboa, H. and Fred, A. (2007). One lead ECG
based personal identification with feature subspace
ensembles. In Proc. of the MLDM ‘07, pp. 770–783.
EigenHeartbeatsforUserIdentification
355