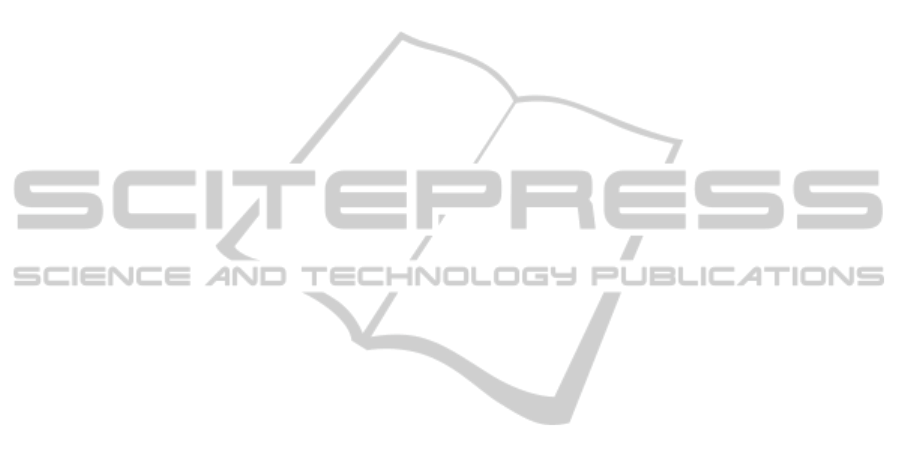
compared to the prediction time of VAR, one may pre-
fer VAR for embedded systems, since the calculation
of the square root for the STD is computational very
expensive on such devices. However, the decision de-
pends on the application, if the earliness is very im-
portant, one would use STD for preprocessing. Fur-
ther, by combining VAR or STD with the adaptive
threshold very good results for movement prediction
could be achieved. In summary, a simple and compu-
tational very efficient way of predicting movements
using EMG data can thus be realized.
For some subjects rather large variations in predic-
tion times, especially for slow movements, could be
observed. Mainly two reasons could have led to these
results. First, it is possible that the subjects somehow
pretensioned there muscles, even if they were told to
move right away without any preparation. This could
have led to an earlier movement prediction. Hence,
if the subjects did so for some movements and for
other not, this could explain the variation in predic-
tion times. Second, the only constraint for slow move-
ments was a minimum time of 1 s for the movement
from the flat board to the buzzer Section 2.1. The
subjects were asked to perform the movements with a
steady speed. However, the subjects may have varied
the initial movement speed, e.g., fast start followed
by a slower movement. Movements with fast initial
speed may be detected later compared to those with
slow initial speed. Thus, the differences in predic-
tion time could be explained by the variation of initial
movement speeds.
Our results show that it is possible to predict
both slow as well as fast movements. We found
that for slow movements earlier prediction times were
achieved. Whether this is a real effect, or might be
due to experimental setup, i.e., datasets from slow and
fast movements were merged for training, cannot fi-
nally answered here. Due to our focus on a real ap-
plication, parameters were not optimized for a certain
speed of movement. This was done since in applica-
tions one cannot relay on a certain movement speed
and the methods will have to deal with both, fast and
slow movements.
ACKNOWLEDGEMENTS
Work was funded by the German Ministry of Eco-
nomics and Technology (grant no. 50 RA 1011 and
grant no. 50 RA 1012). We want to thank Su Kyoung
Kim for her help with the statistics.
REFERENCES
Bonato, P., D’Alessio, T., and Knaflitz, M. (1998). A sta-
tistical method for the measurement of muscle activa-
tion intervals from surface myoelectric signal during
gait. IEEE Transactions on Biomedical Engineering,
45(3):287–299.
Cavanagh, P. R. and Komi, P. V. (1979). Electromechanical
delay in human skeletal muscle under concentric and
eccentric contractions. European Journal of Applied
Physiology and Occupational Physiology, 42(3):159–
163.
Folgheraiter, M., Jordan, M., Straube, S., Seeland, A., Kim,
S.-K., and Kirchner, E. A. (2012). Measuring the im-
provement of the interaction comfort of a wearable ex-
oskeleton. International Journal of Social Robotics,
4(3):285–302.
Hodges, P. W. and Bui, B. H. (1996). A comparison
of computer-based methods for the determination of
onset of muscle contraction using electromyography.
Electroencephalography and Clinical Neurophysiol-
ogy, 101(6):511–519.
Kaiser, J. F. (1990). On a simple algorithm to calculate the
‘energy’ of a signal. In Proc. IEEE International Con-
ference on Acoustics, Speech and Signal Processing
ICASSP-90. Conf, pages 381–384.
Li, X., Zhou, P., and Aruin, A. S. (2007). Teager-kaiser en-
ergy operation of surface EMG improves muscle ac-
tivity onset detection. Annals of Biomedical Engineer-
ing, 35(9):1532–1538.
Nikolic, M. and Krarup, C. (2011). EMGTools, an adaptive
and versatile tool for detailed EMG analysis. Biomed-
ical Engineering, IEEE Transactions on, 58(10):2707
–2718.
Semmaoui, H., Drolet, J., Lakhssassi, A., and Sawan, M.
(2012). Setting adaptive spike detection threshold for
smoothed TEO based on robust statistics theory. IEEE
Transactions on Biomedical Engineering, 59(2):474 –
482.
Solnik, S., Rider, P., Steinweg, K., DeVita, P., and Horto-
bgyi, T. (2010). Teager-kaiser energy operator signal
conditioning improves EMG onset detection. Euro-
pean Journal of Applied Physiology, 110(3):489–498.
Velez, D. R., White, B. C., Motsinger, A. A., Bush, W. S.,
Ritchie, M. D., Williams, S. M., and Moore, J. H.
(2007). A balanced accuracy function for epista-
sis modeling in imbalanced datasets using multifac-
tor dimensionality reduction. Genetic Epidemiology,
31(4):306–315.
Zhou, S., Lawson, D. L., Morrison, W. E., and Fairweather,
I. (1995). Electromechanical delay in isometric mus-
cle contractions evoked by voluntary, reflex and elec-
trical stimulation. European Journal of Applied Phys-
iology and Occupational Physiology, 70(2):138–145.
EMGOnsetDetection-ComparisonofDifferentMethodsforaMovementPredictionTaskbasedonEMG
247