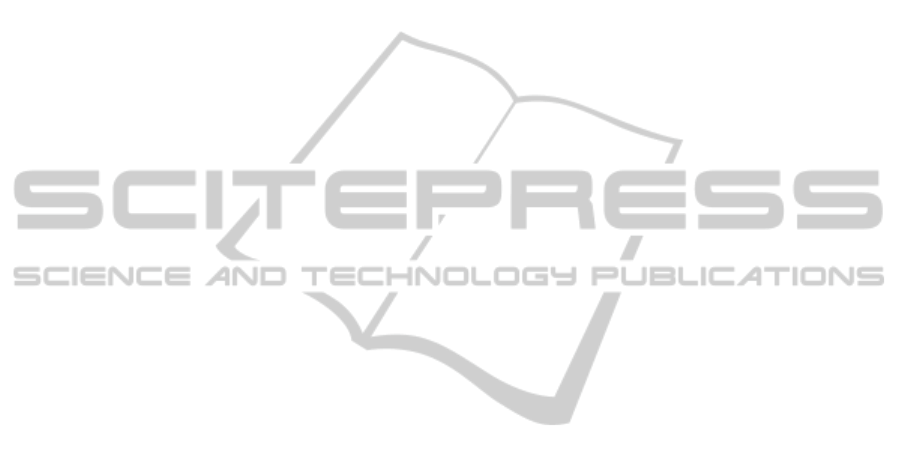
extracted from the documents, we propose to exploit
three different vector spaces.
The reminder of the paper is structured as follows:
in Section 2 we explain how we built the syntactic
and semantic vector space models; in Section 3 we
report the proposed clustering ensemble methodology
reporting some experimental results in Section 4. Fi-
nally in Section 5 some conclusion and future work
are drawn.
2 A METHODOLOGY FOR
BUILDING SYNTACTIC AND
SEMANTIC VECTOR SPACE
MODELS
In this work we adopt three vector space models that
include syntactic and semantic aspects based respec-
tively on frequencies of terms, lemmas and concepts.
In order to represent our document collection in the
vector space models, we extracted a set of terms
from the documents corpus, and therefore, the set of
synonyms corresponding to them. For this aim we
adopted a semantic methodology proposed in (Amato
et al., 2011) for the automatic extraction of concepts
of interest.
The implemented set of procedures aiming at ex-
tracting terms, the corresponding lemmas and the as-
sociated concepts from the input documents are de-
scribed in the following.
Extracting Terms (I Criterium). Starting from the
input documents, by using Text Tokenization proce-
dures, text is arranged into tokens, sequences of char-
acters delimited by separators. Applying Text Nor-
malization procedures,variations of the same lexical
expression are reported in a unique way.
Tokenization and Normalization procedures per-
form a first grouping of the extracted text, introduc-
ing a partitioning scheme that establishes an equiva-
lence class on terms. At this point we built the doc-
features matrix, having a column for each term in
the terms list, which contains the evaluation, for each
document, of the TF-IDF value for every terms in the
list. The TF-IDF values are computed taking into ac-
count both the number of occurrences of each term
for every documents and the terms distribution in the
whole document corpus. This matrix is considered as
input for the clustering algorithm according to the I
Criterium.
Extracting Lemmas (II Criterium). In order to
obtain the lemmas starting from the list of relevant
text, we applied the procedures of Part-Of-Speech
(POS) Tagging and Lemmatization. These procedures
aim at enriching the text with syntactical aspects, aim-
ing at performing a second type of grouping of the
words, on the basis of reduction of terms in a basic
form, independently from the conjugations or decli-
nations in which they appear. Part-Of-Speech (POS)
Tagging consists in the assignment of a grammatical
category to each lexical unit, in order to distinguish
the content words representing noun, verb, adjective
and adverb from the functional words, made of arti-
cles, prepositions and conjunctions, denoting not use-
ful information.
Text Lemmatization is performed in order to re-
duce all the inflected forms to the respective lemma,
or citation form. Lemmatization introduces a second
partitioning scheme on the set of extracted terms, es-
tablishing a new equivalence class on it.
We built a doc-features matrix, having a column
for each lemma in the list, which contains, for each
document, the TF-IDF value of each lemma compar-
ing in it. This value is computed considering the sum
of the number of occurrences of each term that can be
taken back to the same lemma appearing in the doc-
ument. The lemma based doc-features matrix is con-
sidered as input for the clustering algorithm according
to the II Criterium.
Extracting Concepts (III Criterium). In order to
identify concepts, not all words are equally useful:
some of them are semantically more relevant than oth-
ers, and among these words there are lexical items
weighting more than others. In order to “weight” the
importance of a term in a document, we recurred to
TF-IDF index.
Having the list of relevant terms, concepts are
detected by relevant token sets that are semanti-
cally equivalent (synonyms, arranged in sets named
synset). In order to determine the synonym relation
among terms, we exploit external resources (Moscato
et al., 2009) like thesaurus, codifying the relationship
of synonymy among terms.
The number of occurrence of a concept in a doc-
ument is given by the sum of the number of occur-
rences of all terms in its synonyms list that appear
in the document. We built the concept based doc-
features matrix, containing, for each document, the
TF-IDF of every concepts comparing in it. The TF-
IDF values of such matrix is then evaluated on the
basis of the sum of the number of occurrences of each
terms that is synonymous of the input terms, i.e. that
is included in the synonyms list. The concept based
doc-features matrix is considered as input for the clus-
tering algorithm according to the III Criterium.
CombiningSyntacticandSemanticVectorSpaceModelsintheHealthDomainbyusingaClusteringEnsemble
383