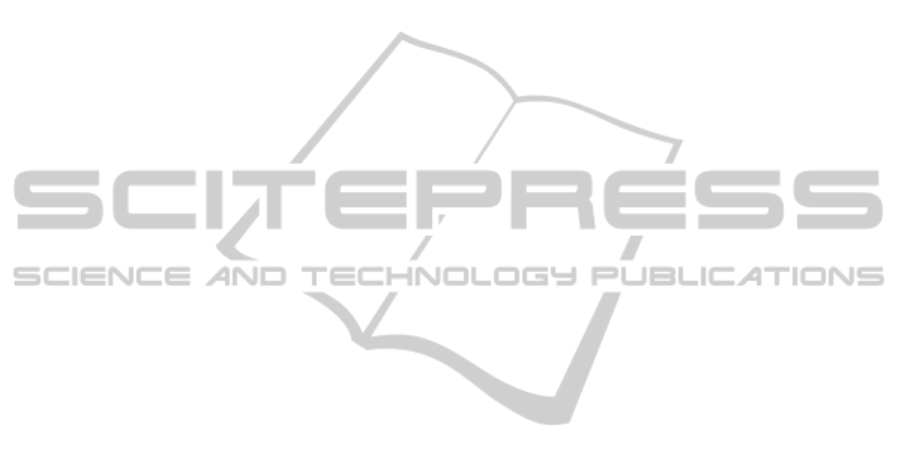
5 CONCLUSIONS
In this study we have laid the basics of a new EMG-
based speech recognition technology, based on elec-
trode arrays instead of single electrodes. We have
presented two basic recognition setups and evaluated
their potential on data sets of different sizes. The
unexpected inconsistency with respect to the optimal
stacking width led us to the introduction of a PCA
preprocessing step before the LDA matrix is com-
puted, which gives us consistent relative Word Error
Rate improvements of 10% to 18%, even for small
training data sets of only 40 sentences.
As a first application of the new array technology,
we have shown that Independent Component Analy-
sis (ICA) typically improves our recognition results.
We also have observed that our method of applying
ICA does not yet always yield satisfactory results: In
one of our setups, we actually observed slightly worse
results than without ICA.
REFERENCES
Belhumeur, P. N., Hespanha, J. P., and Kriegman, D. J.
(1997). Eigenfaces vs Fisherface: Recognition using
Class-specific Linear Projection. IEEE Transactions
on Pattern Analysis and Machine Intelligence, 19:711
– 720.
Bell, A. J. and Sejnowski, T. I. (1995). An Information-
Maximization Approach to Blind Separation and
Blind Deconvolution. Neural Computation, 7:1129 –
1159.
Denby, B., Schultz, T., Honda, K., Hueber, T., and Gilbert,
J. (2010). Silent Speech Interfaces. Speech Commu-
nication, 52(4):270 – 287.
Freitas, J., Teixeira, A., and Dias, M. S. (2012). Towards
a Silent Speech Interface for Portuguese. In Proc.
Biosignals.
Hyvrinen, A. and Oja, E. (2000). Independent Component
Analysis: Algorithms and Applications. Neural Net-
works, 13:411 – 430.
Jorgensen, C. and Dusan, S. (2010). Speech Interfaces
based upon Surface Electromyography. Speech Com-
munication, 52:354 – 366.
Jou, S.-C., Schultz, T., Walliczek, M., Kraft, F., and Waibel,
A. (2006). Towards Continuous Speech Recogni-
tion using Surface Electromyography. In Proc. Inter-
speech, pages 573 – 576, Pittsburgh, PA.
Jung, T., Makeig, S., Humphries, C., Lee, T., Mckeown, M.,
Iragui, V., and Sejnowski, T. (2000). Removing Elec-
troencephalographic Artifacts by Blind Source Sepa-
ration. Psychophysiology, 37:163 – 178.
Lopez-Larraz, E., Mozos, O. M., Antelis, J. M., and
Minguez, J. (2010). Syllable-Based Speech Recog-
nition Using EMG. In Proc. IEEE EMBS.
Maier-Hein, L., Metze, F., Schultz, T., and Waibel, A.
(2005). Session Independent Non-Audible Speech
Recognition Using Surface Electromyography. In
IEEE Workshop on Automatic Speech Recognition
and Understanding, pages 331 – 336, San Juan,
Puerto Rico.
Makeig, S. et al. (2000). EEGLAB: ICA Toolbox for Psy-
chophysiological Research. WWW Site, Swartz Cen-
ter for Computational Neuroscience, Institute of Neu-
ral Computation, University of San Diego California:
www.sccn.ucsd.edu/eeglab/.
Qiao, Z., Zhou, L., and Huang, J. Z. (2009). Sparse Lin-
ear Discriminant Analysis with Applications to High
Dimensional Low Sample Size Data. International
Journal of Applied Mathematics, 39:48 – 60.
Schultz, T. and Wand, M. (2010). Modeling Coarticulation
in Large Vocabulary EMG-based Speech Recognition.
Speech Communication, 52(4):341 – 353.
Ueda, N., Nakano, R., Ghahramani, Z., and Hinton, G. E.
(2000). Split and Merge EM Algorithm for Improving
Gaussian Mixture Density Estimates. Journal of VLSI
Signal Processing, 26:133 – 140.
Wand, M. and Schultz, T. (2010). Speaker-Adaptive Speech
Recognition Based on Surface Electromyography. In
Fred, A., Filipe, J., and Gamboa, H., editors, Biomedi-
cal Engineering Systems and Technologies, volume 52
of Communications in Computer and Information Sci-
ence, pages 271–285. Springer Berlin Heidelberg.
Winter, B. B. and Webster, J. G. (1983). Driven-right-leg
Circuit Design. IEEE Trans. Biomed. Eng., BME-
30:62 – 66.
BIOSIGNALS2013-InternationalConferenceonBio-inspiredSystemsandSignalProcessing
96