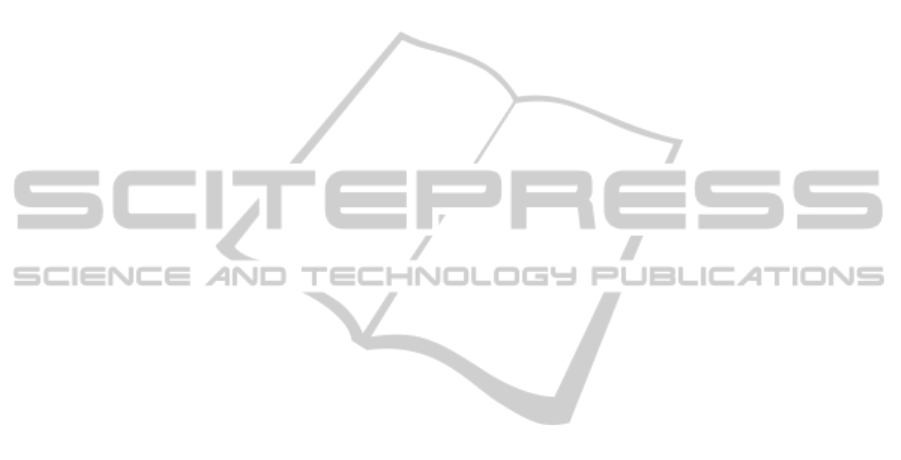
for the GMM and GMM-UBM classifiers (Table 1)
are comparable to previous findings using MFCC
parametrization (Blanco-Murillo et al., 2011b), re-
inforcing our understanding on the role of speech
spectral envelope for automatic detection of OSA. In
Addition, since the GMM-UBM approach on top of
a MFCC parametrization apparently outperforms the
same scheme when using LPC, but not when the al-
ternative GMM-SVM scheme is considered, the role
of the symbiosis between the features set and the clas-
sification scheme is highlighted.
The influence of the training database on the clas-
sification rates achieved by the GMM-UBM scheme
had been addressed in (Blanco-Murillo et al., 2011b);
concluding that better classification results are to be
expected when the characteristics of the database used
to train the UBM match those of the final classifica-
tion task. Nevertheless, by the time the experiments
in (Blanco-Murillo et al., 2011b) were developed, the
UPM database was not available and was worth veri-
fying this conclusion on a LPC-parametrization. The
results obtained have shown that GMM-based classi-
fiers trained on these databases outperform those for a
specific but smaller database, matching perfectly what
had been concluded. Nonetheless, the limitations im-
posed by the apnea database are hard due to the usage
of the /a/ sound, which might not be the best choice
for OSA-related phenomena.
Moreover, as shown in Table 1 the best classifica-
tion results were obtained when following the GMM-
SVM approach, outperforming the GMM and GMM-
UBM schemes (up to 10% absolute improvement,
though the large confidence intervals must be taken
into account). This same pattern is observed for the
AUC. These had already been described in (Wang
et al., 2011), and has been verified for the OSA de-
tection on sustained speech. On the other hand, the
scheme including NAP technique, which was intro-
duced to minimize the effects of undesired variabil-
ity observed in the GMM-SVM classifier, was found
sit in between the previous. The limited performance
of the NAP method might be explained by the dif-
ficulty in finding the spurious sources of variability
within the supervector space, which should have con-
tributed to an improvement in classification. Since
the methodology for a correct discrimination of OSA-
related phenomena is still an open issue, specially re-
garding the selection of the features, accuracy rates
may be enhanced in a number of alternative ways. Re-
sults in this paper suggest that improvement should be
expected on the basis of more complex classifiers and
by focusing on spectral resonances analysis.
ACKNOWLEDGEMENTS
This research was carried out under grants: TEC2009-
14123-C04 from the Spanish Ministry of Education;
AL11-P(I+D)-022 and Ayudas para la realizaci
´
on del
doctorado (RR01/2011) from Universidad Polit
´
ecnica
de Madrid, Spain; and partially funded by the Span-
ish Ministry of Science and Innovation as part of the
TEC2009-14719-C02-02 (PriorSpeech) project.
REFERENCES
Alc
´
azar, J., Fern
´
andez, R., Blanco, J., Hern
´
andez, L.,
L
´
opez, L., Linde, F., and Torre-Toledano, D. (2009).
Automatic speaker recognition techniques: A new
tool for sleep apnoea diagnosis. Am. J. Respir. Crit.
Care Med.
Blanco-Murillo, J., Hern
´
andez, L., Fern
´
andez, R., and
Ramos, D. (2011a). Introducing non-linear analy-
sis into sustained speech characterization to improve
sleep apnea detection. Advances in Nonlinear Speech
Processing, pages 215–223.
Blanco-Murillo, J. L., Fern
´
andez-Pozo, R., Torre-Toledano,
D., Caminero, J., and L
´
opez, E. (2011b). Analyz-
ing training dependencies and posterior fusion in dis-
criminant classification of apnea patients based on
sustained and connected speech. In INTERSPEECH,
pages 3033–3036.
Campbell, W., Campbell, J., Reynolds, D. A., Singer, E.,
and Torrescarrasquillo, P. (2006). Support vector ma-
chines for speaker and language recognition. Com-
puter Speech & Language, 20(2-3):210–229.
Elisha, O., Tarasiuk, A., and Zigel, Y. (2011). Detection of
obstructive sleep apnea using speech signal analysis.
In MAVEBA.
Fern
´
andez-Pozo, R., Blanco-Murillo, J. L., Hern
´
andez-
G
´
omez, L., L
´
opez-Gonzalo, E., Alc
´
azar Ram
´
ırez, J.,
and Toledano, D. T. (2009). Assessment of severe
apnoea through voice analysis, automatic speech, and
speaker recognition techniques. EURASIP J. Adv. Sig-
nal Process, 2009:6:1–6:11.
Fox, A. W., Monoson, P. K., and Morgan, C. D. (1989).
Speech dysfunction of obstructive sleep apnea. a
discriminant analysis of its descriptors. Chest,
96(3):589–95.
Kinnunen, T. and Li, H. (2009). An Overview of Text-
Independent Speaker Recognition: from Features to
Supervectors. Image Processing.
Puertas, F. J., Pin, G., Mar
´
ıa, J. M., and Dur
´
an, J. (2005).
Documento de consenso nacional sobre el s
´
ındrome
de apneas-hipopneas del sue
˜
no. Grupo Espa
˜
nol De
Sue
˜
no.
Wang, X., Zhang, J., and Yan, Y. (2011). Discrimination
between pathological and normal voices using GMM-
SVM approach. Journal of voice, 25(1):38–43.
GMM-basedClassifiersfortheAutomaticDetectionofObstructiveSleepApnea
367