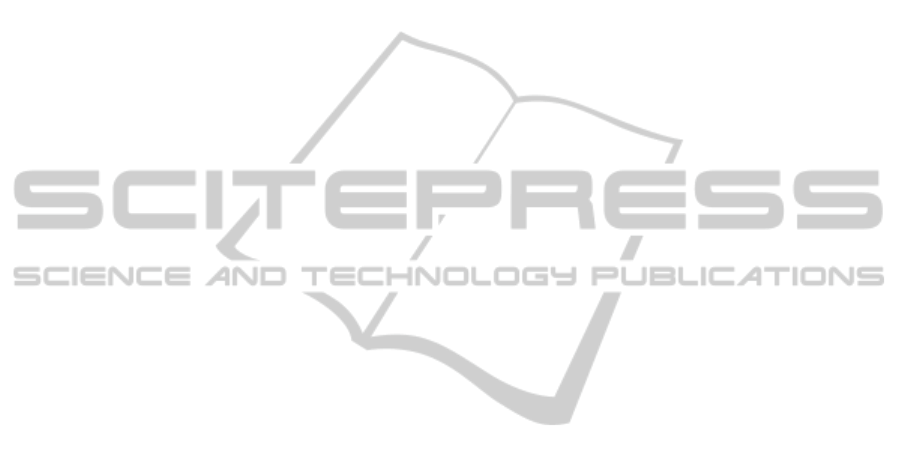
program and perform the recommended exercises
correctly. The recommended physical exercises were
of the following kind: biceps curl, squatting, torso
bending, etc. The involved feature detail level was
high with prevalent use of the topological
representation. Performed exercises were correctly
recognized achieving 99.2% and 95.6% of
specificity and sensitivity, respectively. The most
computationally expensive steps were pre-
processing, feature extraction, and posture
classification. They were evaluated in terms of
processing time that was constant for pre-processing
and classification resulting respectively in 20 ms and
15 ms per frame. The volumetric descriptor taken an
average processing time of about 20 ms,
corresponding to about 18 fps (frame-per-second).
The topological approach, on the other hand,
required a slightly increasing processing time among
hierarchical levels from an average value of 40 ms to
44 ms due to the incremental occurrence of self-
occlusions, achieving up to 13 fps.
4 CONCLUSIONS
The main contribution of this work is to design and
evaluate a unified solution for TOF SN-based in-
home monitoring suitable for different AAL
application scenarios. An open (OpenAAL inspired)
computational framework has been suggested able to
classify a large class of postures and detect events of
interest accommodating easily (i.e. with self-
calibration), at the same time, wall-mounting sensor
installations more convenient to cover home
environments avoiding large occluding objects.
Moreover, the suggested computational framework
was optimized and validated for embedded
processing to meet typical in-home application
requirements, such as low-power consumption,
noiselessness and compactness. The system was able
to adapt effectively to four different AAL scenarios
exploiting an application-driven multilevel feature
extraction to reliably detect several relevant events
and overcoming, at the same time, well-known
problems affecting traditional monitoring systems in
a privacy preserving way. The ongoing work
concerns the on-field validation of the system that
will be deployed in elderly dwellings at support of
two different ambient assisted living scenarios
concerning the detection of dangerous events and
abnormal behaviours.
ACKNOWLEDGEMENTS
The presented work has been carried out within the
BAITAH project funded by the Italian Ministry of
Education, University and Research (MIUR).
REFERENCES
Debnath R., Takahide N., Takahashi H., 2004. A decision
based one-against-one method for multi-class support
vector machine. Pattern Analysis and
Applications;7(2):164-175.
Diraco, G., Leone, A., Siciliano, P., 2011. Geodesic-based
human posture analysis by using a single 3D TOF
camera. In: Proc of ISIE;1329-1334.
Fuxreiter, T., Mayer, C., Hanke, S., Gira, M., Sili, M.,
Kropf, J., 2010. A modular platform for event
recognition in smart homes. In: 12th IEEE
Healthcom;1-6.
Khoshelham, K., 2011. Accuracy analysis of Kinect depth
data. In: Proc of ISPRS Workshop on Laser Scanning.
Leone, A., Diraco, G., Siciliano P., 2011. Detecting falls
with 3D range camera in ambient assisted living
applications: a preliminary study. Med Eng
Phys;33(6):770-81.
Li, W., Zhang, Z., Liu, Z., 2010. Action recognition based
on a bag of 3D points. In: Proc of CVPRW;9-14.
Mastorakis, G., Makris, D., 2012. Fall detection system
using Kinect’s infrared sensor. J Real-Time Image
Proc;1-12.
MESA Imaging AG, 2011. SR4000 Data Sheet Rev.5.1.
<http://www.mesa-imaging.ch>.
Park, S., Kautz, H., 2008. Privacy-preserving recognition
of activities in daily living from multi-view silhouettes
and RFID-based training. In: AAAI symposium on AI
in eldercare, Arlington, Virginia USA.
Schäfer, J., 2010. A Middleware for Self-Organising
Distributed Ambient Assisted Living Applications. In:
Workshop Selbstorganisierende, Adaptive,
Kontextsensitive verteilte Systeme (SAKS).
UniversAAL project 2012. http://www.universaal.org/.
Wientapper, F., Ahrens, K., Wuest, H., Bockholt, U.,
2009. Linear-projection-based classification of human
postures in time-of-flight data. In: Proc of
ICSMC;559-564.
Wolf, P., Schmidt, A., Parada Otte, J., Klein, M.,
Rollwage, S., Koenig-Ries, B., Dettborn, T.,
Gabdulkhakova, A., 2010. openAAL - the open source
middleware for ambient-assisted living (AAL). In:
AALIANCE conference, Malaga, Spain.
Won-Seok, C., Yang-Shin, K., Se-Young, O., Jeihun, L.,
2012. Fast iterative closest point framework for 3D
LIDAR data in intelligent vehicle. In: IEEE Intelligent
Vehicles Symposium (IV);1029–1034.
Xiao, Y., Siebert, P., Werghi, N., 2004. Topological
segmentation of discrete human body shapes in
various postures based on geodesic distance. In: Proc
of ICPR 2004;3:131-135.
AAL-OrientedTOFSensorNetworkforIndoorMonitoring
239