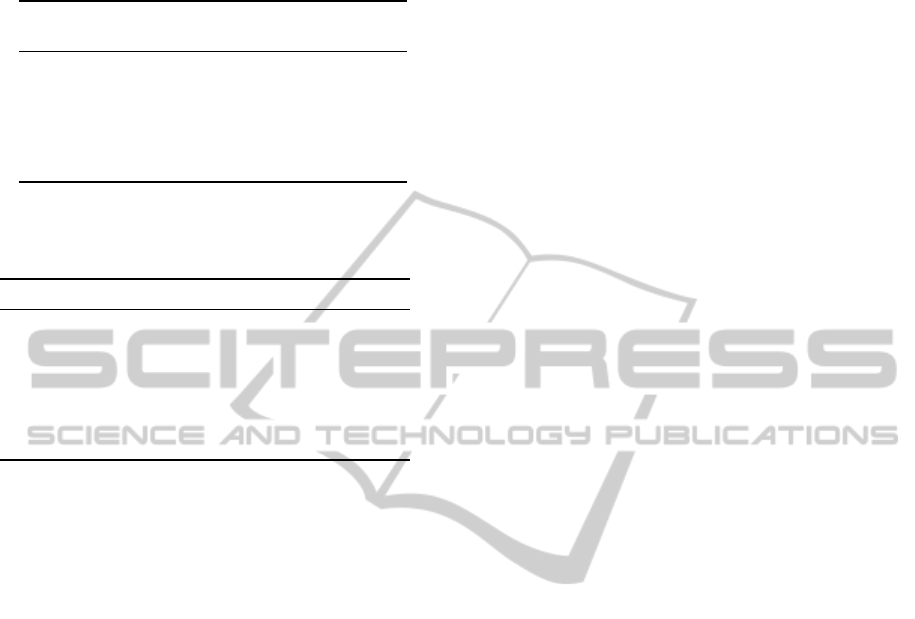
Table 2: Classification accuracy (mean and standard devi-
ation) per subject for the GON&ACC set in a subject’s de-
pendent and independent context.
Classification Accuracy (%)
Subjects Subject dependent Subject independent
1 97.32 ± 0.04 36.13 ± 21.43
2 99.11 ± 0.02 83.19 ± 10.11
3 97.32 ± 0.04 89.08 ± 4.56
4 98.22 ± 0.03 78.15 ± 8.17
5 98.22 ± 0.03 94.12 ± 0.15
6 99.11 ± 0.04 91.52 ± 0.87
Table 3: Confusion matrix for GON&ACC in a subject-
dependent and subject-independent (between brackets) con-
text, in percentage.
W St Si Su Sd A D
W 95(89) 0 0 0 3(4) 1(0) 1(7)
St 0 100(83) 0 0 0(17) 0 0
Si 0 1(0) 99(83) 0(17) 0 0 0
Su 0 0 0 98(92) 2(4) 0(4) 0
Sd 0(4) 0(1) 0(1) 0(9) 98(66) 0(1) 2(18)
A 0(1) 0 0 1(32) 0 99(67) 0
D 0(19) 0 0 0 1(9) 0 99(72)
4.2 Experiment 2: Subject-independent
We evaluate the subject-independent performance
with a leave-one-out cross-validation on the subjects.
That means we test on one subjects data and train
on the data of the remaining subjects. We use all
samples in our database, resulting in 595 samples for
training and 119 samples for testing for each subject.
We reach accuracies between 49% and 79% for
the different signal modalities. Table 1 shows the
results and, analogously to the subject-dependent
evaluation, we can state that the combination
GON&ACC has the highest accuracy. Nevertheless,
compared to the subject-dependent case, the accuracy
is much lower which can be explained by the varia-
tions in human motion for different subjects.
Table 3 shows the confusion matrix for
GON&ACC. We can see that the movements
stairs down and ascend are easily confused with
descend and stairs up, respectively. This is not
surprising since the performed motions are very
similar for these pairs of activities.
Table 2 shows the classification accuracy per subject
for the GON&ACC set.
Concerning the evaluation per subject, the rec-
ognizer performed with an accuracy between 36%
and 94% with an average of 79%. Due to the small
number of subjects, the generalization ability of the
classifier is relatively low and thus we can see a low
performance for subject 1.
5 CONCLUSIONS
In this work we evaluated the possibility to recog-
nize human activities from different biosignal sensors.
We reach a person-dependent accuracy of 98% and
a person-independent accuracy of 79%. The combi-
nation of GON&ACC signals gives the highest accu-
racy. Based on the dataset and the models for the mo-
tion primitives acquired in this work, we will investi-
gate continuous recognition of activities in the future,
which will be the next step towards an active intelli-
gent knee orthosis.
ACKNOWLEDGEMENTS
We would like to thank the KIT Sports Institute for
their support, Plux for supplying biosignal sensors
and the volunteers for the participation.
REFERENCES
Amma, C., Georgi, M., and Schultz, T. (2012). Airwriting:
Hands-free mobile text input by spotting and continu-
ous recognition of 3d-space handwriting with inertial
sensors. In Wearable Computers (ISWC), 2012 16th
International Symposium on, pages 52–59. IEEE.
Lukowicz, P., Ward, J., Junker, H., Stger, M., Trster, G.,
Atrash, A., and Starner, T. (2004). Recognizing work-
shop activity using body worn microphones and ac-
celerometers. In Pervasive Computing, volume 3001
of Lecture Notes in Computer Science, pages 18–32.
Springer.
Mathie, M., Coster, A., Lovell, N., and Celler, B. (2003).
Detection of daily physical activities using a triaxial
accelerometer. Medical and Biological Engineering
and Computing, 41(3):296–301.
Rabiner, L. (1989). A tutorial on hidden markov models
and selected applications in speech recognition. Pro-
ceedings of the IEEE, 77(2):257–286.
Rowe, P., Myles, C., Walker, C., and Nutton, R. (2000).
Knee joint kinematics in gait and other functional
activities measured using flexible electrogoniometry:
how much knee motion is sufficient for normal daily
life? Gait & posture, 12(2):143–155.
Suda, E. (2011). An
´
alise eletromiogr
´
afica comparativa
de tornozelo durante a aterrissagem em jogadores de
v
ˆ
olei com instabilidade cr
ˆ
onica.
Sutherland, D. (2002). The evolution of clinical gait anal-
ysis: Part ii kinematics. Gait & posture, 16(2):159–
179.
Welk, G. and Differding, J. (2000). The utility of the digi-
walker step counter ro assess daily physical activity
patterns. Medicine and Science in Sports and Exer-
cise, 9(32):481–488.
HumanActivityRecognitionforanIntelligentKneeOrthosis
371