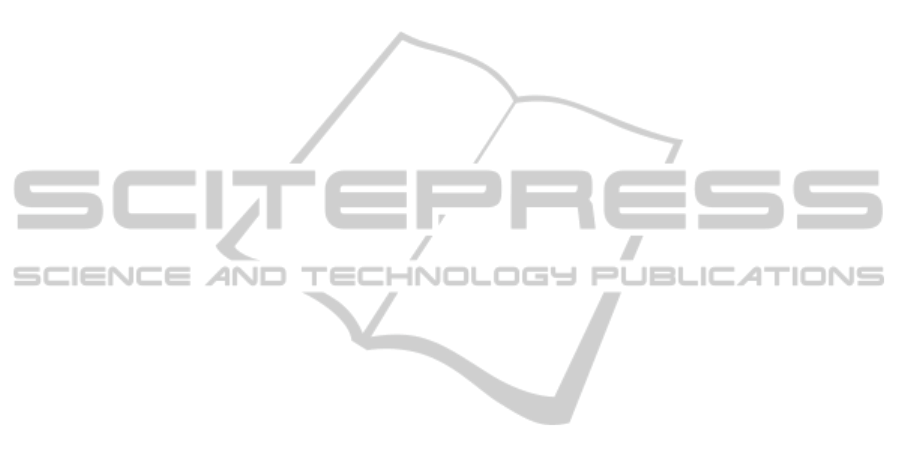
the approach plan synthesis from causal models by
means of precondition and effect rules is competitive
with state of the art methods for AR.
6 DISCUSSION AND OUTLOOK
To summarize the experiments: the results showed
that using geometric observation models based on the
room topology instead of trained observation mod-
els, does not hinder the AR and is competitive to the
trained models. Even more, in the case of the gener-
ated models SCM and MCM combined with the ge-
ometric models, they outperformed the same causal
models combined with trained observation models.
This was observed in the experiments performed on
both datasets, thus supporting hypothesis H
1
.
Additionally, both scenario-specific and generic
synthesis-based models are able to achieve a perfor-
mance at a similar level as the trained model, pro-
vided that suitable observation and duration models
are used. Thus we consider hypothesis H
2
as sup-
ported. Furthermore, the generic multi-agent model
is able to correctly recognize not only dataset D
1
but also dataset D
2
and at a high performance level
(98.9% accuracy on the team level and 98.6% accu-
racy on the agent level), providing evidence for hy-
potheses H
3
. Finally, the data show that the use of
multi-agent modeling does not decrease performance,
but indeed is able to increase it, supporting hypothe-
ses H
4
. The multi-agent model allows to temporally
decouple state changes of agents, thus providing a fine
grained state estimation at the agent level. The data
show a solid performance of 94% (D
1
) resp. 98%
(D
2
) for this agent-level state estimation.
We thus conclude with the statement that plan-
synthesis approaches indeed allow the construction of
reusable models. Furthermore, our experiences show
approximate inference is feasible, enabling realistic
problem sizes and multi-agent interactions – however,
a successful application of these techniques beyond
short term activities requires the ability to support ap-
propriate duration models. Finally, the usage of train-
ing free observation models allows competitive to the
trained models inference performance.
As next step, we intend to show that the modeling
approach proposed here is also applicable to other do-
mains, such as recognizing activities of daily living.
While we believe this to cause no fundamental prob-
lem, we expect to gather new experiences on model-
ing methodology. As the approach uses the Bayesian
inference paradigm, adding additional inference tasks
from this paradigm, such as prediction and parameter
estimation, are, at least in principle, tasks for which
solutions exist. (We look forward to results of learn-
ing the weights of action selection features from data,
as this would provide empirical data on the weight of
heuristics in human action selection strategies.)
REFERENCES
Bonet, B. and Geffner, H. (2005). mGPT: A Probabilistic
Planner Based on Heuristic Search. J. Artif. Intell. Res.
(JAIR), 24:933–944.
Hein, A., Burghardt, C., Giersich, M., and Kirste, T.
(2009). Model-based Inference Techniques for detect-
ing High-Level Team Intentions. In Gottfried, B. and
Aghajan, H., editors, Behaviour Monitoring and In-
terpretation, volume 3. IOS Press.
Hiatt, L. M., Harrison, A. M., and Trafton, J. G. (2011).
Accommodating Human Variability in Human-Robot
Teams through Theory of Mind. In Proc 22nd Intl J
Conf on Artif. Intell. (IJCAI).
Kaiser, K. and Miksch, S. (2004). Treating Temporal Infor-
mation in Plan and Process Modeling. Technical Re-
port Asgaard-TR-2004-1, Institute of Software Tech-
nology and Interactive Systems, Vienna University of
Technology, Vienna.
Kirste, T. and Kr
¨
uger, F. (2012). CCBM-A tool for activ-
ity recognition using Computational Causal Behavior
Models. Technical Report CS-01-12, Institut f
¨
ur Infor-
matik, Universit
¨
at Rostock, Rostock, Germany. ISSN
0944-5900.
Okeyo, G., Chen, L., Wang, H., and Sterritt, R. (2011).
Ontology-Based Learning Framework for Activity
Assistance in an Adaptive Smart Home. In Chen, L.
et al., editors, Activity Recognition in Pervasive Intel-
ligent Environments, volume 4. Atlantis Press.
Ramirez, M. and Geffner, H. (2011). Goal Recognition over
POMDPs: Inferring the Intention of a POMDP Agent.
In Proc 22nd Intl J Conf on Artif. Intell. (IJCAI).
Roy, P. C. et al. (2011). A Possibilistic Approach for Ac-
tivity Recognition in Smart Homes for Cognitive As-
sistance to Alzheimer’s Patients. In Chen, L. et al.,
editors, Activity Recognition in Pervasive Intelligent
Environments, volume 4. Atlantis Press.
Sadilek, A. and Kautz, H. (2012). Location-Based Reason-
ing about Complex Multi-Agent Behavior. J. Artif.
Intell. Res. (JAIR), 43:87–133.
Storf, H., Becker, M., and Riedl, M. (2009). Rule-based ac-
tivity recognition framework: Challenges, technique
and learning. In Proc. 3rd Intl. Conf. on Pervasive
Computing Technologies for Healthcare.
ICAART2013-InternationalConferenceonAgentsandArtificialIntelligence
288