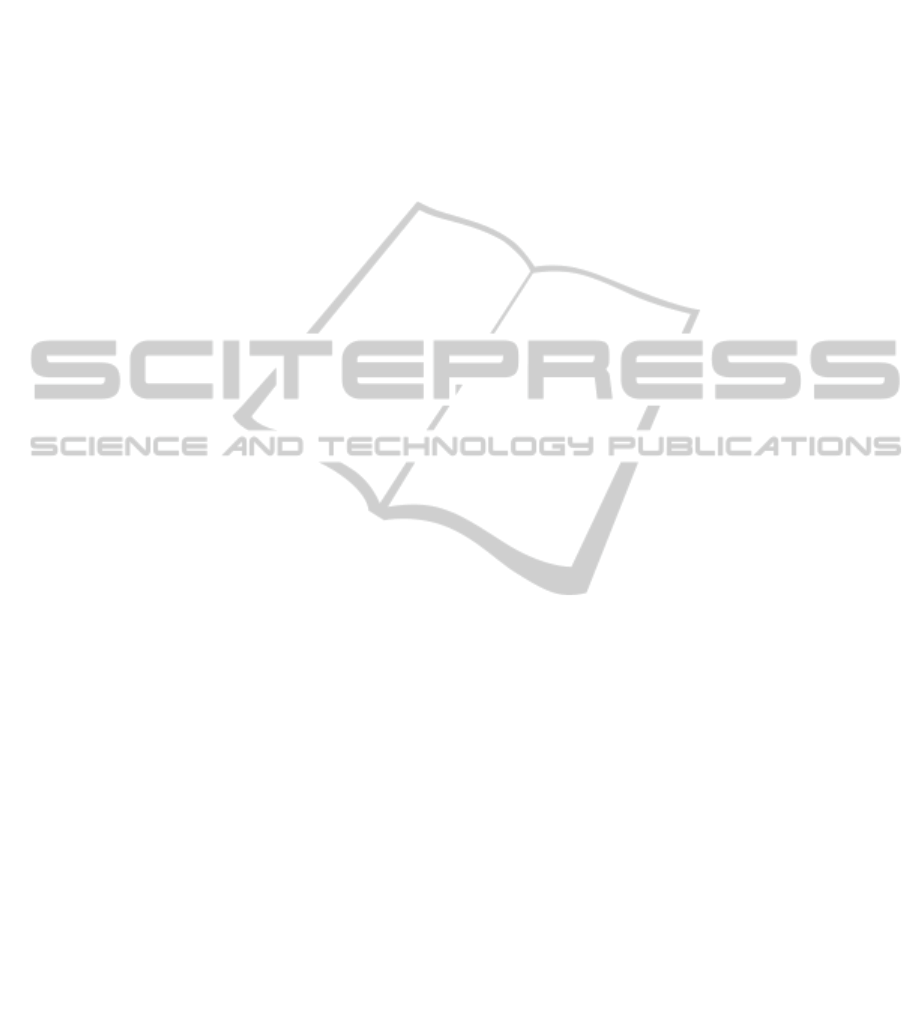
alternative 1 and alternative 3, alternative 2 will
follow it respectively.
5 CONCLUSIONS
In this paper, we present a hybrid model using both
AHP and intitionistic fuzzy TOPSIS (IFT) models
for supplier selection under fuzzy environment to
account for vagueness and uncertainty. In the
evaluation process, the ratings of each alternative,
given with intitionistic fuzzy information, were
represented as IFNs. In this hybrid model, AHP is
used to assign weights to the criteria, while IFT is
employed to calculate the full-ranking of the
alternatives. The AHP-IFT hybrid model was used
to aggregate the rating of DMs. Multiple DMs are
often preferred rather than a single DM to avoid the
minimize the partiality in the decision process.
Therefore, group decision making process for
alternative selection is very useful. The presented
approach not only validates the methods, but also
considers a more extensive list of criteria, suitable
for supplier selection. The AHP-IFT hybrid model
has capability to deal with similar types of the same
situations.
REFERENCES
Atanassov, K. T., 1986. Intuitionistic fuzzy sets. Fuzzy
Sets and Systems. Vol.20, pp. 87–96.
Atanassov, K.T., 1999. Intuitionistic fuzzy sets. Springer,
Heidelberg.
Boran, F. E., Genç, S., Kurt, M., & Akay, D., 2009. A
multi-criteria intuitionistic fuzzy group decision
making for supplier selection with TOPSIS method.
Expert Systems with Applications. 36(8), pp. 11363-
11368.
Boran, F. E., 2011. An integrated intuitionistic fuzzy
Multi-Criteria Decision-Making method for facility
location selection. Mathematical and Computational
Applications. 16(2) pp.487-496.
Boran, F. E., Boran, K., Menlik, T., 2012. The evaluation
of renewable energy technologies for electricity
generation in Turkey using intuitionistic fuzzy
TOPSIS. Energy Sources, Part B: Economics,
Planning and Policy. 7, 81-90.
Humphreys, P., Huang, G., Cadden, T., and Mcivor, R.
2007. Integrating design metrics within the early
supplier selection process. Journal of Purchasing and
Supply Management, 13, pp.42–52.
Rouyendegh, B.D., Erkan, T. E., 2012. Selection the Best
Supplier Using AHP Method. African Journal of
Business Management. 6(4), pp.1455-1462.
Rouyendegh, B. D., 2012. Evaluating Projects Based on
Intuitionistic Fuzzy Group Decision Making. Journal
of Applied Mathematics. Vol. 2012, 16 pages,
doi:10.1155/2012/ 824265.
Shyur, H. J., Shih, H. S., 2006. A hybrid MCDM model
for strategic vendor selection. Mathematical and
Computer Modelling. 44( 7-8), pp. 749-761.
Simchi-Levi, D., Kaminsky, P., 2003. Designing and
Managing Supply Chain. McGraw-Hill Higher
Education, Second Edition.
Vokurka, R. J., Fliedner, G. J., 1998. The journey toward
agility. Industrial Management & Data Systems. 98
(4), pp.165–171.
Xu, Z. S., 2007d. Intuitionistic fuzzy aggregation
operators. IEEE Transaction of Fuzzy Systems. 15 (6),
pp. 1179–1187.
ICAART2013-InternationalConferenceonAgentsandArtificialIntelligence
522