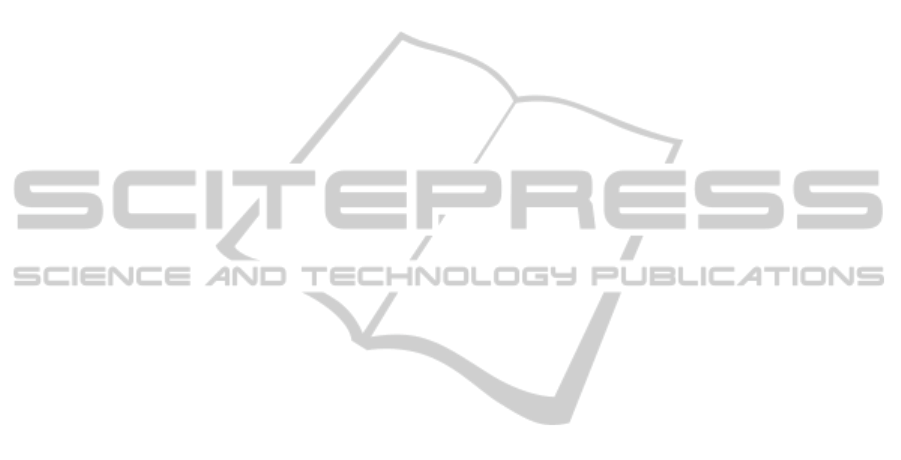
This frequency implies that simulated ABM can
control 20 real robots {R
i
} with an spatial resolution
under the cm, which is acceptable for the laboratory
previously presented, even if working at 25% more
than the maximum throughput of the top current
analyzers (8000 tests/hour). Note that marks and
objects are more than one cm away from each other.
5.2 Synchronization with Reality
The view of the model enables creating a mixed-
reality environment in which it is possible to design,
supervise and control transport systems of
applications.
As already explained, the model records the
actual delays between requests from T
i
and
corresponding acknowledgements from R
i
, but also
compares them to the delays from V
i
.
For every request-ack. pair between T
i
and {R
i
,
V
i
}, if the actual delay is longer, the view of the
corresponding agent remains stand still until the time
gap is covered. On the other side, if the real delay is
shorter than the expected one, the view is updated
for the missed, un-simulated time. This fact implies
that the WCET must be twice as short as the shortest
delay so that these extra periods required by the
simulator to synchronize virtual robots to their
physical counterparts do not cause any loss in inputs
from the actual plant. Therefore this synchronizing
mechanism works fine only if the control loop
period is shorter than half the delays to be measured.
6 CONCLUSIONS
In this work we have focused on the internal
transportation aspect of systems that run applications
on the industrial domain and proposed a framework
to design and deploy the corresponding subsystems.
The framework uses an ABM simulator as a key
tool that is used in the following cases: 1) for
functional validation; 2) for plant characterization,
which includes testing whether real time
requirements are met, parameter identification, and
controller setup, and 3) as a model for the controller
of the transportation system, including a mixed-
reality environment for monitoring and supervising
in human-assisted operation.
We have shown that the higher levels can be
simulated and, thus, verified in a straithforward
manner and that it is possible to synchronize the
model execution with the real plant to use it as an
actual controller.
Preliminar results show that the proposed
strategy minimizes the time-to-prototype as the
development platform is the same that the
deployment one.
In the near future we expect to have complete
experimental results on real-time control with this
framework and to develop strategies to solve
synchronization conflicts when simulation and
reality differ.
REFERENCES
Armendáriz, M., Burguillo, J.C., Peleteiro, A., Arnould,
G., Khadraoui, D. (2011). Carpooling: A multiagent
simulation in Netlogo. In Proc. of the 25th European
Conf. on Modelling and Simulation (ECMS), Krakow
(Poland).
Davidsson, P., Henesey, L., Ramstedt, L., Törnquist, J.,
Wernstedt, F. (2005). An analysis of agent-based
approaches to transport logistics. In Transportation
Research Part C 1. 255–271.
De Wolf, T., Holvoet, T. (2003). Towards Autonomic
Computing: Agent-Based Modelling, Dynamical
Systems Analysis, and Decentralised Control. In Proc.
of the First Int’l. Workshop on Autonomic Computing
Principles and Architectures.
Fernández-Caballero, A., Gascueña, J.M. (2009). Develo-
ping Multi-Agent Systems through Integrating Prome-
theus, INGENIAS and ICARO-T. In Proc. of Int’l.
Conf. on Agents and Artificial Intelligence (ICAART).
Himoff, J., Rzevski, G., Hinton, M., Skobelev, P. (2006).
MAGENTA Technology: Multi-Agent Logistics i-
Scheduler for Road Transportation. In Proc. of
AAMAS, Hokkaido (Japan).
Kashif, A., Binh Le., X.H., Dugdale, J., Ploix, S. (2011).
Agent-based framework to simulate inhabitants’
behaviour in domestic settings for energy
management. In Proc. of Int’l. Conf. on Agents and
Artificial Intelligence (ICAART).
Mathieu, P., Secq, Y. (2012). Environment Updating and
Agent Scheduling Policies in Agent-based Simulators.
In Proc. of Int’l. Conf. on Agents and Artificial
Intelligence (ICAART), 170-175.
Ribas-Xirgo, Ll., Miró-Vicente, A., Chaile, I. F., Velasco-
González, A. J. (2012). Multi-Agent Model of a
Sample Transport System for Modular In-Vitro
Diagnostics Laboratories. In Proc. of Emerging
Technologies and Factory Automation (ETFA),
Krakow (Poland).
Santa-Eulalia, L.A., Halladjian, G., D’Amours, S., Frayret,
J.-M. (2011). Integrated methodological frameworks
for modeling agent-based advanced supply chain
planning systems: A systematic literature review. In J.
Ind. Eng. & Management, JIEM, 2011 – 4(4):624-668.
Schreiber, S., Fay, A. (2011). Requirements for the
benchmarking of decentralized manufacturing control
AnAgent-basedModelofAutonomousAutomated-GuidedVehiclesforInternalTransportationinAutomatedLaboratories
267