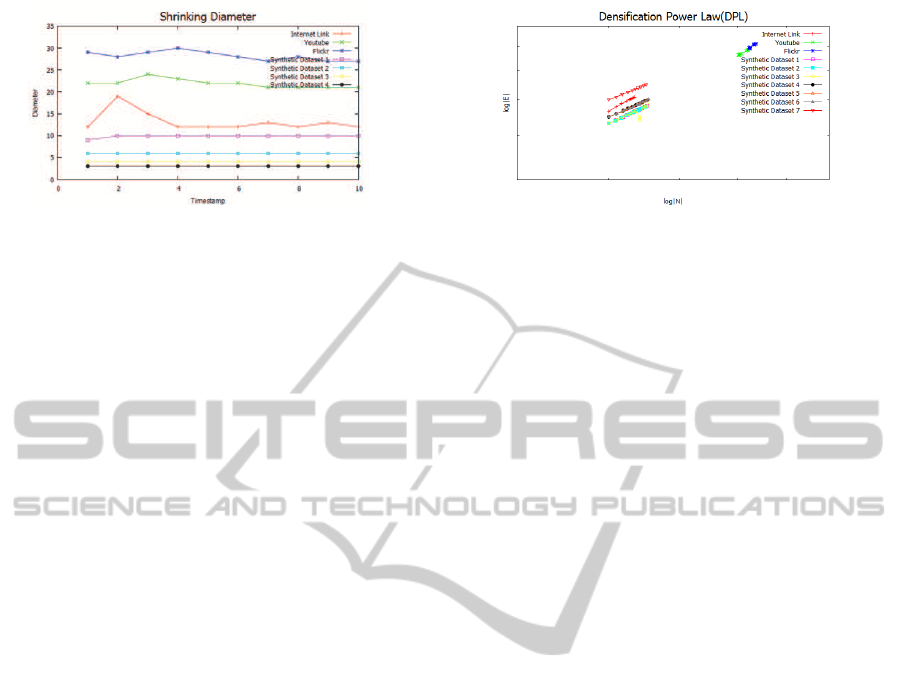
Figure 2: Shrinking Diameter.
4.3 Results of Dynamic Properties
Shrinking Diameter. Figure 2 shows plots of the
change in diameter values of the datasets over time
with timestamp value on x axis and diameter value
on y axis. The results of real graphs conform the
shrinking diameter property. In synthetic graphs, we
obtained stabilized diameter results over time, some
times even after a small increase. This is because of
the nature of the generator model which has prefer-
ential attachment property where the diameter grows
slowly with the number of nodes n.
Densification Power Law (DPL). Our DPL results
are shown in Figure 3. All our real and synthetic
graphs obey the DPL, with exponents ranging be-
tween 1.06 and 1.24. The power-law exponent being
greater than 1 shows that there is a superlinearity be-
tween the number of nodes and the number of edges
in the graph.
5 CONCLUSIONS
In this paper, we presented a study on realistic so-
cial graph generation using Barabasi-Albert model.
We used static and dynamic graph properties to ana-
lyze synthetically generated graphs and measure how
fit the generated graphs to the real one. We gen-
erated graphs with different parameter settings and
compared them to the real graphs.
Results indicate that greater preferential attach-
ment power value cause small diameter and radius
values with disrupting the power law degree distri-
bution of the graphs. The parameter of the number
of edges to add in each step effects the number of
edges in each timestamp and results in greater den-
sification power law degree. Synthetically generated
graphs do not obey shrinking diameter property of
dynamic social networks. However, they have bet-
ter clustering coefficient degree with smaller diameter
than real graphs. Furthermore, these synthetic graphs
have power law degree distributions and fit densifica-
tion power law property of dynamic social networks.
Figure 3: Densification Power Law.
ACKNOWLEDGEMENTS
The authors were supported by the Scientific
and Technological Research Council of Turkey
(TUBITAK) EEEAG project 110E027.
REFERENCES
Barabasi, A.-L. and Albert, R. (1999). Emergence of scal-
ing in random networks.
Chakrabarti, D. and Faloutsos, C. (2006). Graph min-
ing: Laws, generators, and algorithms. ACM Comput.
Surv., 38(1).
Erd¨os, P. and R´enyi, A. (1959). On random graphs i. Pub-
licationes Mathematicae Debrecen, 6:290.
Leskovec, J., Chakrabarti, D., Kleinberg, J. M., and Falout-
sos, C. (2005a). Realistic, mathematically tractable
graph generation and evolution, using kronecker mul-
tiplication. In Jorge, A., Torgo, L., Brazdil, P., Cama-
cho, R., and Gama, J., editors, PKDD, volume 3721 of
Lecture Notes in Computer Science, pages 133–145.
Springer.
Leskovec, J. and Faloutsos, C. (2007). Scalable modeling
of real graphs using kronecker multiplication. In Pro-
ceedings of the 24th international conference on Ma-
chine learning, ICML ’07, pages 497–504, New York,
NY, USA. ACM.
Leskovec, J., Kleinberg, J., and Faloutsos, C. (2005b).
Graphs over time: densification laws, shrinking diam-
eters and possible explanations. In Proceedings of the
eleventh ACM SIGKDD international conference on
Knowledge discovery in data mining, KDD ’05, pages
177–187, New York, NY, USA. ACM.
Mislove, A. (2009). Online Social Networks: Measurement,
Analysis, and Applications to Distributed Information
Systems. PhD thesis, Rice University, Department of
Computer Science.
Mislove, A., Koppula, H. S., Gummadi, K. P., Druschel,
P., and Bhattacharjee, B. (2008). Growth of the flickr
social network. In Proceedings of the 1st ACM SIG-
COMM Workshop on Social Networks (WOSN’08).
Travers, J. and Milgram, S. (1969). An Experimental Study
of the Small World Problem. Sociometry, 32(4):425–
443.
Watts, D. J. and Strogatz, S. H. (1998). Collective dynamics
of ’small-world’ networks. Nature, 393(6684):440–
442.
ICAART2013-InternationalConferenceonAgentsandArtificialIntelligence
526