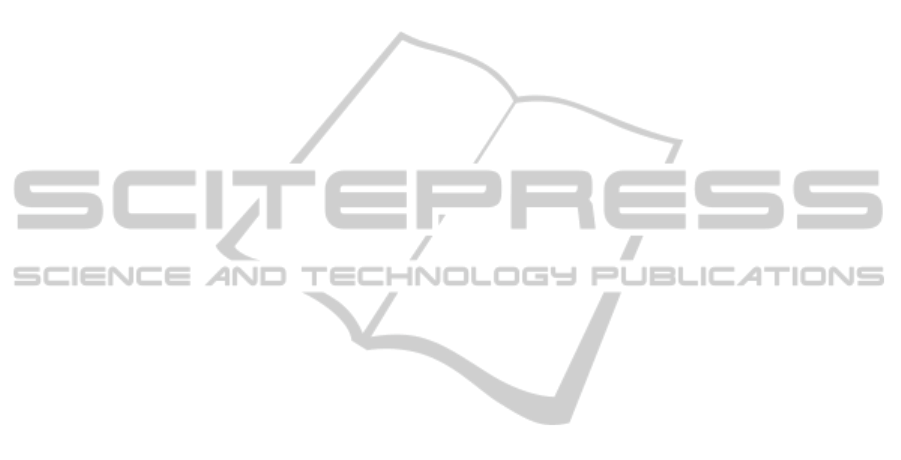
7 CONCLUSIONS
In this paper we describe how the local learning with
the accumulation of individual decisions advocates
the creation of new emergent structure. We suggest a
set of simulations that analyze the positive impact of
individual behavior in the improvement of the global
performance of the system. This individual behavior
that is based on the bio-inspired cognitivemap, allows
the agents to avoid planning problems before a com-
plete exploration of the environment. It also leads to
the same results as a pheromon-based system without
the need to leave a physical trace in the environment.
Thus, the coupling of the individual behavior with the
embodiement of the agents (satisfaction of their moti-
vations) can solve multi-objective planning problems
although formally the algorithm is not able to ”mix
and to merge and to optimize” several objectives. In
conclusion, we suggest an emergent multi-objective
optimization. Finally we suggest the evaluation of
the emergent structures in MAS by comparing our
CMAS, the MAS based on cemetery organization of
ants and the random MAS with a linear programming
approach. The results obtained confirm the perfor-
mance of our emergent behavior based on cognitive
processes which allow us to have adequate solutions
that approximate the linear programming solution. To
study the limits of emergent structures in real world,
we started to validate the adaptive capability of the
cognitive map in a real multi-robot system (Chatty
et al., 2012) and now we are trying to add the deposit
system in the multi-robot system.
ACKNOWLEDGEMENTS
The authors would like to thank the financial sup-
port of the Tunisian General Direction of Scientific
Research and Technological Renovation (DGRSRT),
under the ARUB program 01/UR/11 02, the Institut
Francais de Tunisie
REFERENCES
Billing, E. A. (2010). Cognitive perspectcoives on robot
behavior. In ICAART (2), pages 373–382.
Bonabeau, E. and Theraulaz, G. (1994). Intelligence Col-
lective. Hermes.
Brooks, R. A. (1994). Coherent behavior from many adap-
tive processes. In in SAB, pages 22–29, Cambridge,
MA, USA. MIT Press.
Chatty, A., Gaussier, P., Kallel, I., Laroque, P., and Alimi,
A. (2012). Adaptation capability of cognitive map im-
proves behaviors of social robotics. In in IEEE ICDL-
EpiRob (to appear).
Chatty, A., Kallel, I., Gaussier, P., and Alimi, A. (2011).
Emergent complex behaviors from swarm robotic sys-
tems by local rules. In IEEE Symposium Series on
Computational Intelligence, (RiiSS), pages 69 –76.
Deneubourg, J. L., Goss, S., Franks, N., Franks, A. S., De-
train, C., and Chr´etien, L. (1990). The dynamics of
collective sorting robot-like ants and ant-like robots.
In SAB, pages 356–363, Cambridge, MA, USA. MIT
Press.
Gaussier, P., Revel, A., Banquet, J. P., and Babeau, V.
(2002). From view cells and place cells to cognitive
map learning: processing stages of the hippocampal
system. Biological Cybernetics, 86(1):15–28.
Gaussier, P. and Zrehen, S. (1994). Avoiding the world
model trap: An acting robot does not need to be so
smart! Robotics and Computer-Integrated Manufac-
turing, 11(4):279 – 286.
Laroque, P., Pirard, F., Archambault, F., Quoy, M., and
Gaussier, P. (2010). Comparing multi-agent system
and mixed-integer programming approaches on a sim-
ple optimization problem. In IC-AI, pages 977–981.
Lubenov, E. V. and Siapas, A.G. (2009). Hippocampal theta
oscillations are travelling waves. volume 459, pages
534–539. Nature Publishing Group.
Martinet, L.-E., Sheynikhovich, D., Benchenane, K., and
Arleo, A. (2011). Spatial Learning and Action Plan-
ning in a Prefrontal Cortical Network Model. PLoS
Comput Biol, 7(5).
Mataric, M.-J. (1992). Designing Emergent Behaviors:
From Local Interactions to Collective Intelligence. In
Meyer, J.A., R. H. and Wilson, S., editors, Proceed-
ings of the Second Conference on Simulation of Adap-
tive Behavior, pages 1–6. MIT Press.
Mathieu, P. and Secq, Y. (2012). Environment updating and
agent scheduling policies in agent-based simulators.
In ICAART (2), pages 170–175.
Matsui, T. and Matsuo, H. (2012). Analysis for distributed
cooperation based on linear programming method. In
ICAART (2), pages 228–233.
O’Keefe, J. and Nadel, L. (1978). The hippocampus as
a cognitive map / John O’Keefe and Lynn Nadel.
Clarendon Press ; Oxford University Press, Oxford.
Pepin, N., Simonin, O., and Charpillet, F. (2009). Intelligent
Tiles: Putting Situated Multi-Agents Models in Real
World. In AAAI, A., editor, ICAART, Porto, Portugal.
Portugal, D. and Rocha, R. P. (2012). Extracting topological
information from grid maps for robot navigation. In
ICAART (1), pages 137–143.
Prodan, I., Olaru, S., Stoica, C., and Niculescu, S.-I. (2012).
Predictive control for trajectory tracking and decen-
tralized navigation of multi-agent formations. In Fil-
ipe, J. and Fred, A. L. N., editors, ICAART (2), pages
209–214. SciTePress.
Simonin, O., Ferber, J., and Decugis, V. (1998). Perfor-
mances analysis in collective systems. ICMAS, pages
469–. IEEE Computer Society.
Yadati, C., Witteveen, C., and Zhang, Y. (2010). Coordi-
nating agents - an analysis of coordination in supply-
chain management tasks. In ICAART (2), pages 218–
223.
EvaluationofEmergentStructuresina"Cognitive"Multi-AgentSystembasedonOn-lineBuildingandLearningofa
CognitiveMap
275