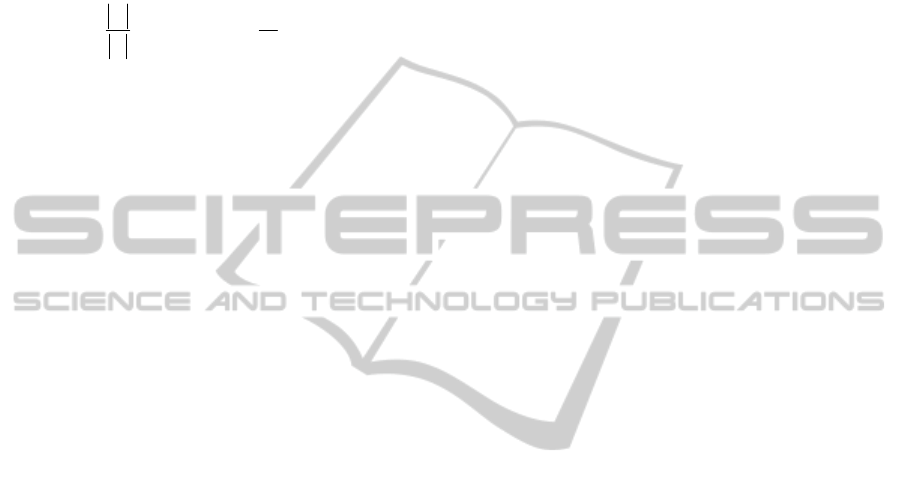
summary information, as well as storage of
individual student data for offline use.
The model computes the quality q and also q
t
of
all subject matters (Eq. 5) for every student. Vector
Q is the graduate profile vector, and K is the
knowledge vector. The quality values are important
for the evaluation of effectiveness and success of the
study process and the quality of the graduates.
t
t
t
q
k
q
Q
K
q ,
(5)
Experiments which were performed were designed
to validate the model using real process of students’
studies. Due to the structure of the admission
procedure it was not possible to use data from it for
setting the student parameters, and initial values of
vectors K, A, G, and M were thus generated from
estimated intervals with Gaussian probability
distributions. The values of course vectors I and O
were obtained from discussions with students. Initial
results confirm the hypothesis, that especially
preconditions together with capacity limits affect the
structure of the student’s study plans.
The main goal of future work is to specify
parameters of the model from data in the faculty
information system and from data contained in the
admissions results.
Our first goal is to determine the parameters for the
probability distributions used for setting of the
student simulation vectors K, A, M a G. The model
can then be used for exploring global study
processes.
In the second step we would like to suggest
mechanisms enabling the use of the proposed system
for modeling real learning process of the students by
utilizing regularly updated data. This would allow
prediction of their study results and limit potentially
problematic situations.
6 CONCLUSIONS
This paper describes the agent-based model for the
simulation of the process of university studies. Its
aim is to contribute to the effectiveness and setting
of study plans and processes. The first experiments
were conducted with the model to verify its
applicability for solving practical problems. Further
development will focus mainly on validating and
fine-tuning the model for accurate simulation of real
world situations and verifying its predictive
capability.
REFERENCES
Ammar, M. B., Neji, M., Alimi, A. M. & Gouardères, G.,
2010. The Affective Tutoring System. Expert Systems
with Applications: An International Journal archive,
37(4), pp. 3013-3023.
AnyLogic Company, 1992-2012. AnyLogic. [Online]
Available at: http://www.anylogic.com
[Accessed 2. 11. 2012].
Argonne National Laboratory, 2012. Repast. [Online]
Available at: http://repast.sourceforge.net/
[Accessed 15. 4. 2012].
Ayala, A. P., 2009. Student Modelling Based on
Ontologies. ACIIDS '09 Proceedings of the 2009 First
Asian Conference on Intelligent Information and
Database Systems, pp. 392-397.
Dimitrova, V., 2003. STyLE-OLM: Interactive Open
Learner Modelling. International Journal of Artificial
Intelligence in Education, 13(1), pp. 35-78.
Eccles, D. W. & Groth, P. T., 2006. Agent coordination
and communication in sociotechnological systems.
Interacting with Computers, 18(6), pp. 1170-1185.
Forrester, J. W., 1961. Industrial dynamics. Cambridge,
MA: MIT Press.
Helgesen, O. & Nesset, E., 2009. Modelling and
Managing Student Loyalty: A Study of a Norwegian
University College. Scandinavian Journal of
Educational Research, 53(4), p. 327 – 345.
Izumi, K., Matsui, H. & Matsuo, Y., 2007. Socially
embedded multi agent based simulation of financial
market. Proceedings of the 6th international Joint
Conference on Autonomous Agents and Multiagent
Systems (Honolulu, Hawaii, May 14 - 18, 2007)..
Komis, V., Avouris, N. & Fidas, C., 2002. Computer-
Supported Collaborative Concept Mapping: Study of
Synchronous Peer Interaction. Education and
Information Technologies, 7(2), pp. 169-188.
Siebers, P. O. & Aickelin, U., 2008. Introduction to Multi-
Agent Simulation. In: F. Adam & P. Humphreys, eds.
Encyclopedia of Decision Making and Decision
Support Technologies. Pennsylvania: Idea Group
Publishing, pp. 554-564.
Swarm Development Group, 2012. SWARM. [Online]
Available at: http://www.swarm.org
[Accessed 10. 5. 2012].
Tang, Y., Parsons, S. & Sklar, E., 2006. Agent-based
modeling of human education data. Proceedings of the
fifth international joint conference on Autonomous
agents and multiagent systems, pp. 129-131.
Wilensky, U., 1999. NetLogo. [Online]
Available at: http://ccl.northwestern.edu/netlogo/
[Accessed 22. 8. 2012].
Xiang-Min, G. & Ming-Yong, P., 2010. Modeling and
simulating dynamic evolvement of collective learning
behaviors by Voronoi diagram. Proceedings
LSMS/ICSEE'10, pp. 548-554.
ICAART2013-InternationalConferenceonAgentsandArtificialIntelligence
406