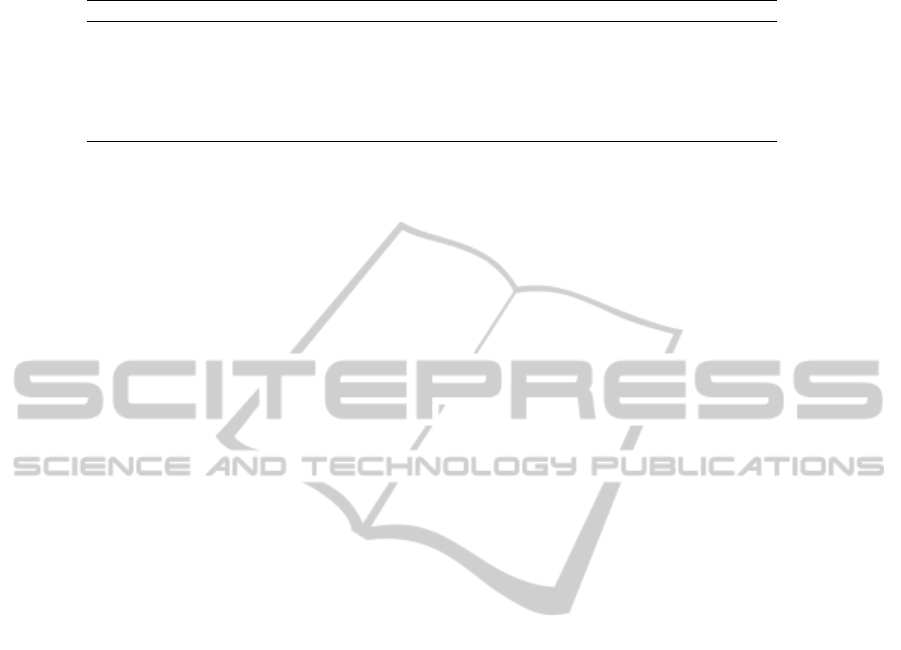
Table 1: 2D annotations of a narrative dialogue between a parent and his/her child.
Line Speaker Utterance Annotations
31 P Who could have stolen the crown? Q - F - -
32 C The crown, it’s in! A - F - -
33 P Do you believe it? Q H K - -
34 C Yes A - F - -
35 P But Babar doesn’t know that it’s in A P N O J
36 P So he says that the crown is a bomb A P N C J
annotations.
The annotation grid corresponds to the follow-
ing coding scheme: Column 1: an (A)ffirmation, a
(Q)uery, a request for paying attention to the story
(D), or a demand for general attention (G). Column 2:
reference of the utterance. It can refer to the character
(P), the interlocutor (H) or the speaker (R). Column
3: an (E)motion, a (V)olition, an observable or a non-
observable cognition (B or N), an epistemic statement
(K), an assumption (Y) or a (S)urprise. The surprise is
distinguished from other emotions because of its link
with the incidental belief. Columns 4 and 5: expla-
nations with cause / (C)onsequence, (O)pposition or
empathy (M), which can be applied either to explain
the story (J), or to precise a situation with a personal
context (F).
3 KNOWLEDGE EXTRACTION
Dialogue Pattern Extraction
With our matrix representation, a dialogue pattern is
defined as a set of annotations which occurs in several
dialogues. The method designed to extract significant
dialogue patterns consists in a regularity extraction
step based on matrix alignment using dynamic pro-
gramming and a clustering step using machine learn-
ing heuristics to group and select the recurrent dia-
logue patterns. The clustering process is applied on
a similarity graph computed during the matrix align-
ment.
The method for extracting two-dimensional pat-
terns is a generalisation of the local string edition dis-
tance. The edit distance between two string S
1
and
S
2
corresponds to the minimal cost of the three ele-
mentary edit operations (insertion, deletion and sub-
stitution of characters) for converting S
1
to S
2
. Two-
dimensional pattern extraction corresponds to matrix
alignment. A local alignment of two matrices M
1
and
M
2
, of size m
1
× n
1
and m
2
× n
2
respectively, consists
in finding the portion of M
1
and M
2
which are the
most similar. To this end, a four-dimensional matrix
T of size (m
1
+ 1) × (n
1
+ 1) × (m
2
+ 1) × (n
2
+ 1)
is computed, such that T [i][ j][k][l] is equal to the lo-
cal edition distance between S
1
[0..i − 1][0.. j − 1] and
S
2
[0..k −1][0..l −1] for all i ∈ J1, m
1
−1K, j ∈ J1, n
1
−
1K, k ∈ J1, m
2
− 1K and l ∈ J1,n
2
− 1K. In our heuris-
tic, the calculation of T is obtained by the minimisa-
tion of a recurrence formula. Once T is computed, the
best local alignment is found from the position of the
maximal value in T , through a trace-back algorithm
to infer the characters which are part of the align-
ment. Figure 1, commented in Section 4, presents
an example of alignment extracted from the corpus.
Details about the two dimensional pattern extraction
algorithm can be found in (Lecroq et al., 2012).
The matrix alignment algorithm extracts the pat-
terns in pairs. To determine the importance of each
pattern, we group them using various standard cluster-
ing heuristics The idea is that large clusters of patterns
represent behaviours which are commonly adopted
by humans, whereas small clusters tend to contain
marginal patterns. A matrix of similarities between
patterns is computed through a global edition distance
applied on all pairs of selected patterns. This similar-
ity matrix is used as input for the clustering heuristics.
The method has been tested on the corpus of nar-
rative dialogues. During the extraction phase, 1740
dialogue patterns have been collected.
Predicting the Interaction of the Child
As our goal is to build a dialogue model dedicated to
narrative ECAs that stimulates child interaction, we
have to model the arising of the child’s interaction,
focusing on event prediction. In other words, we look
for sequences of dialogue events leading to child’s in-
teraction. We split the data over each turn of utter-
ance, in other words over each sequence of parents as-
sertion or question and child’s interaction. The prob-
lem consists therefore in predicting the end of each
turn.
The matrices that encode dialogues are considered
as sequences of features, each sequence ending with
the child’s interaction. For instance, the sequences
corresponding to Table 1, are: < (QF) >, < (QHK) >
, < (APNOJ)(APNOJ) >
The algorithm, without candidate enumeration,
mines the episodes with recursive projections, in a
greedy manner. The combinatorial explosion is lim-
ited with two anti-monotone constraints: the support
of the currently computed episode (the number of se-
ICAART2013-InternationalConferenceonAgentsandArtificialIntelligence
528