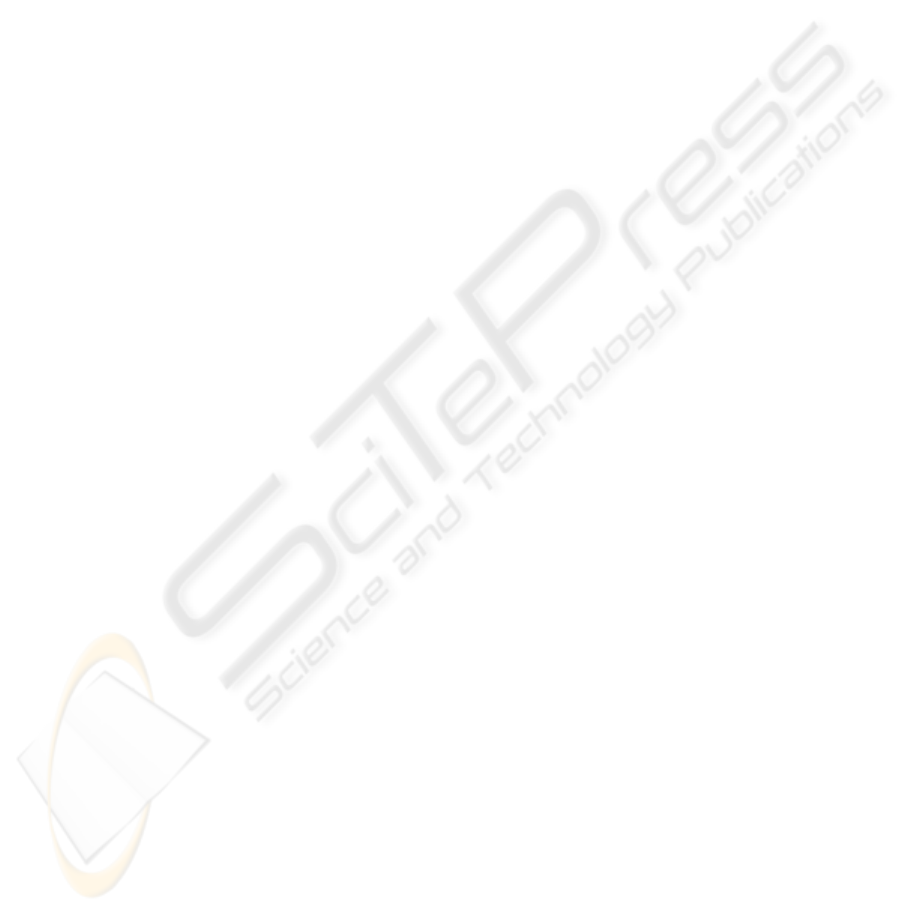
Prediction of Organ Geometry from Demographic and
Anthropometric Data based on Supervised Learning Approach
using Statistical Shape Atlas
Yoshito Otake
1,2
, Catherine Carneal
3
, Blake Lucas
1
, Gaurav Thawait
4
, John Carrino
4
, Brian Corner
5
,
Marina Carboni
5
, Barry DeCristofano
5
, Michale Maffeo
5
, Andrew Merkle
3
and Mehran Armand
2,3
1
Department of Computer Science, The Johns Hopkins University, Baltimore, MD, U.S.A.
2
Department of Mechanical Engineering, The Johns Hopkins University, Baltimore, MD, U.S.A.
3
Applied Physics Laboratory, The Johns Hopkins University, Laurel, MD, U.S.A.
4
Department of Radiology, The Johns Hopkins Hospital, Baltimore, MD, U.S.A.
5
US Army Natick Soldier Research Development and Engineering Center, Natick, MA, U.S.A.
Keywords: Statistical Shape Atlas, Demographic and Anthropometric Data, Principal Component Analysis, Regression
Analysis, Supervised Learning, Allometry.
Abstract: We propose a method relating internal human organ geometries and non-invasively acquired information
such as demographic and anthropometric data. We first apply a dimensionality reduction technique to a
training dataset to represent the organ geometry with low dimensional feature coordinates. Regression
analysis is then used to determine a regression function between feature coordinates and the external
measurements of the subjects. Feature coordinates for the organ of an unknown subject are then predicted
from external measurements using the regression function, subsequently the organ geometry is estimated
from the feature coordinates. As an example case, lung shapes represented as a point distribution model was
analyzed based on demographic (age, gender, race), and several anthropometric measurements (height,
weight, and chest dimensions). The training dataset consisted of 124 topologically consistent lung shapes
created from thoracic CT scans. The prediction error of lung shape of an unknown subject based on 11
demographic and anthropometric information was 10.71 ± 5.48 mm. This proposed approach is applicable
to scenarios where the prediction of internal geometries from external parameters is of interest. Examples
include the use of external measurements as a prior information for image quality improvement in low dose
CT, and optimization of CT scanning protocol.
1 INTRODUCTION
Analysis of organ geometries using statistical shape
atlases is a prevalent trend in various target
application fields, such as cardiac modelling (Frangi
et al., 2002), pelvis shape analysis for dose reduction
(Chintalapani et al., 2010), 4-dimensional lung
motion modelling (Ehrhardt et al., 2011), and a
small animal research using Micro-CT (Hongkai
Wang et al., 2012).
Most existing statistical shape atlases of human
organ are created from an unnonimized CT dataset,
thus the analyses were often confined to organ shape
among a select disease group or subject population.
To our knowledge, the relationship between
anthropometric and demographic data with a
statistical atlas of generalized population is not
investigated as yet.
In order to address this gap, we collected a
thoracic computed tomography (CT) dataset together
with non-invasively acquired “external
measeurements” including demographic information
and several anthropometric metrics. We propose a
method to analyze correlation between a subject’s
external measurements and their internal organ
geometry based on a supervised learning approach.
As an initial feasibility study, we used lung as a
target organ and considered its geometric features as
a cloud of connected points (Point Distribution
Model, PDM).
Prediction of information about internal structure
which are typically measured in invasive way from
these readily measureable external measurements
365
Otake Y., Catherine C., Lucas B., Thawait G., Carrino J., Corner B., Carboni M., DeCristofano B., Maffeo M., Merkle A. and Armand M. (2013).
Prediction of Organ Geometry from Demographic and Anthropometric Data based on Supervised Learning Approach using Statistical Shape Atlas.
In Proceedings of the 2nd International Conference on Pattern Recognition Applications and Methods, pages 365-374
DOI: 10.5220/0004263803650374
Copyright
c
SciTePress