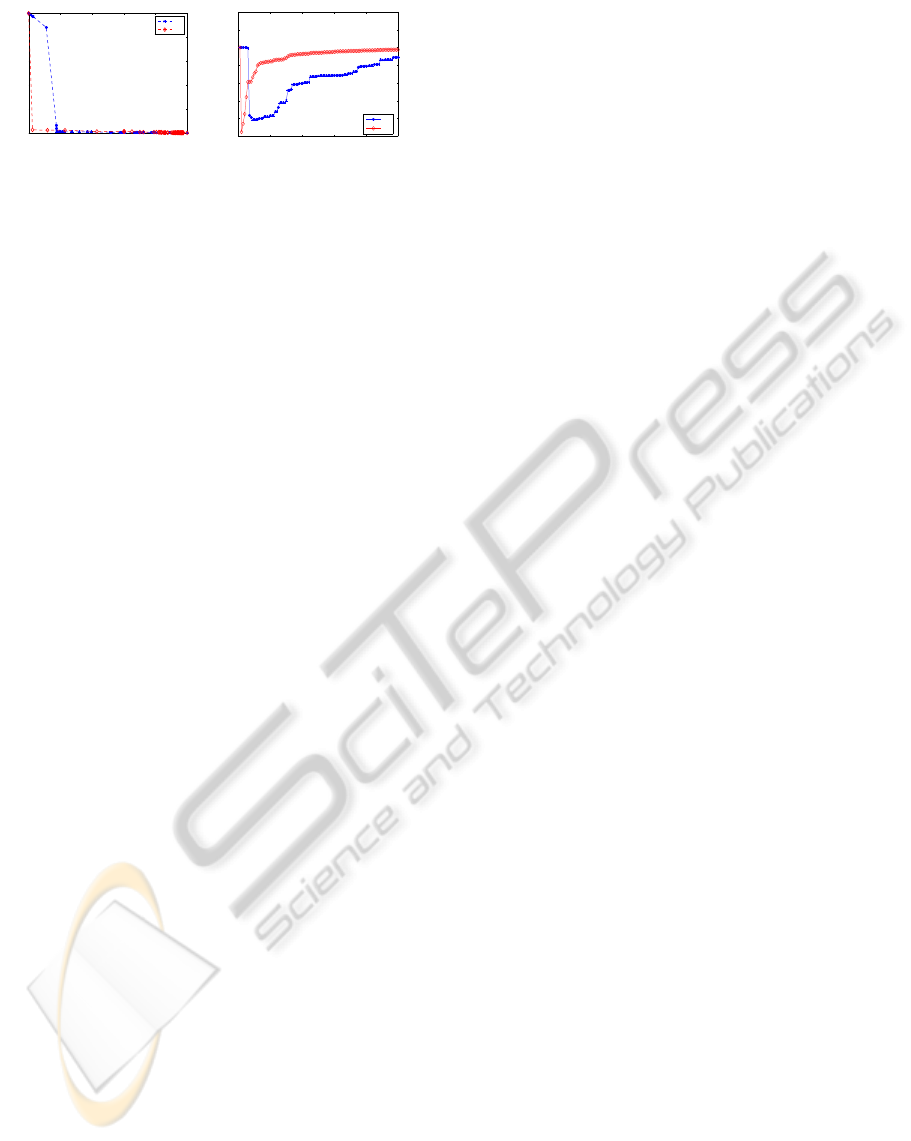
0 0.2 0.4 0.6 0.8 1
0
0.2
0.4
0.6
0.8
1
0 20 40 60 80 100
0
0.2
0.4
0.6
0.8
1
1.2
1.4
Figure 1: Synthetic dataset with two clusters. Top: ROC
curve for single-link (SL) and average-link (AL). Bottom:
Error rate for SL and AL, which corresponds to the sum of
the two type of errors, ε
1
and ε
2
.
2.1 ROC Curve
In this paper, a ROC curve shows the fraction of false
positives out of the positives versus the fraction of
false negatives out of the negatives. Consider two
given points x
a
, x
b
; a type I error occurs if those
two points are clustered separated when they should
be in the same cluster, i.e., for any pair of objects
(x
a
, x
b
), type I error is given by ε
1
≡ P(x
a
∈ C
i
, x
b
∈
C
j
|x
a
, x
b
∈ P
l
), i 6= j and type II error is given by
ε
2
≡ P(x
a
, x
b
∈ C
i
|x
a
∈ P
j
, x
b
∈ P
l
), j 6= l. In terms of
the ROC curve, for a clustering algorithm with vary-
ing k, for each k we compute the pair (ε
k
1
, ε
k
2
), and we
join those pairs to get the curve (see figure 1 top).
We define that a clustering partition C is concor-
dant with the true labeling, P , of the data if
ε
1
= 0 if k ≤ m
ε
2
= 0 if k ≥ m
ε
1
= ε
2
= 0 if k = m.
(1)
We call a ROC curve proper if, when varying k,
ε
1
increases whenever ε
2
decreases and vice-versa.
These increases and decreases are not strict. Intu-
itively, small values of k should yield low values of ε
1
(at the cost of higher ε
2
) if the clustering algorithm is
working correctly. Similarly, large values of k should
lead to low values of ε
2
(at the cost of higher ε
1
).
2.2 Evaluate Robustness
At some point, a clustering algorithm can make bad
choices: e.g., an agglomerative method might merge
two clusters that in reality should not be together.
Looking at the curve can help in predicting what is
the optimal number of clusters for that algorithm,
k
0
, which minimizes the error rate; it is given by
k
0
= argmin(ε
1
+ ε
2
). In figure 1, right, we plot the
sum of the two types of errors as a function of the
number of clusters, k; this is equivalent to the error
rate. In figure 1 left, we see a knee in the curves which
corresponds to the lowest error rate found in the bot-
tom plot. We see that average-link (AL) merges clus-
ters correctly to obtain the lowest error rate when the
true number of clusters is reached (k = 2). On the
other hand, for single-link (SL), the minimum error
rate is only achieved when k = 9. Since that number
is incorrect, the minimum of the AL curve is lower
(better) than the minimum of the SL curve.
In the previous example, visually inspecting the
ROC curve shows that AL performs better than SL:
the former’s curve is closer to the axes than the lat-
ter’s. However, visual inspection is not possible if
we want to compare several clustering algorithms; we
need a quantitative criterion. The criterion we choose
is the AUC. A lower AUC value corresponds to a bet-
ter clustering algorithm, which will be close to the
true labeling for some k. In the example, we have
AUC = 0.0247 for AL and AUC = 0.1385 for SL.
Also, if AUC = 0 then the clustering partition C is
concordant with the true labeling, P . This definition
is consistent with (1).
The ROC curve can also be useful to study the
robustness of clustering algorithms to the choice of k.
We say that a clustering algorithm is more robust to
the choice of k than another algorithm if the former’s
AUC is smaller than the latter’s. In the example, AL
is more robust to the choice of k than SL.
2.3 ROC and Parameter Selection
Some hierarchical clustering algorithms need to set a
parameter in order to find a good partition of the data
(Fred and Leit
˜
ao, 2003; Aidos and Fred, 2011). Also,
most of the partitional clustering algorithms have pa-
rameters which need to be defined, or are dependent
of some initialization. For example, k-means is a par-
titional algorithm that needs to be initialized.
Typically, k-means is run with several initializa-
tions and the mean of some measure (e.g. error rate)
is computed, or the intrinsic criterion (sum of the dis-
tance of all points to their respective centroid) is used,
to choose the best run. We could also consider a fixed
initialization for k-means like the one proposed by (Su
and Dy, 2007). In this paper we compute the mean
(over all runs) of type I error and type II error to plot
the ROC curve for this algorithm.
2.4 Fully Supervised Case
In the fully supervised case, we assume that we have
access to the labels of all samples and we apply clus-
tering algorithms to that data. The main goal is to
study the robustness of each clustering algorithm as
described in section 2.2.
TheAreaundertheROCCurveasaCriterionforClusteringEvaluation
277