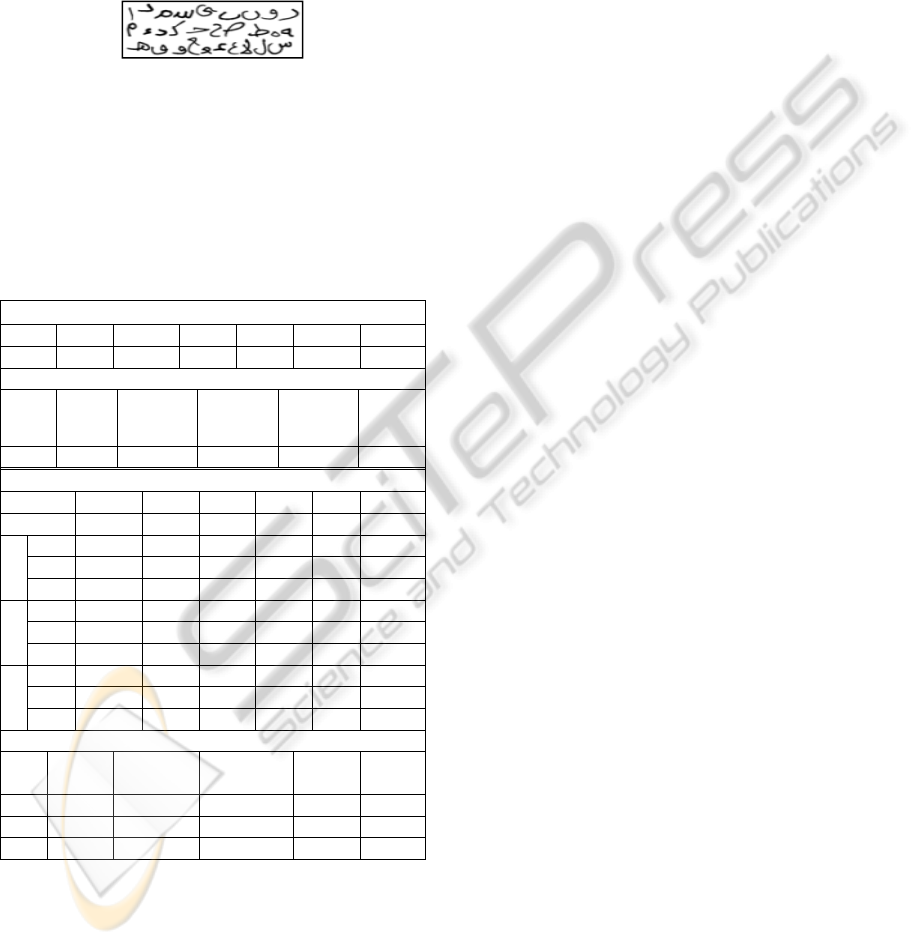
3.3 Handwritten Dataset
The handwritten dataset contained 2900 samples (29
classes X 100 samples). The samples were chosen
randomly from the multi-writers IFN/ENIT dataset
(400 different writers). The segmentation and the
diacritics elimination were done manually. Figure 3
presents examples from the handwritten dataset.
Figure 3: Examples from IFN/ENIT dataset.
Table 4 presents top results using handwritten
dataset. The poor performance rates are explained by
the dataset nature (handwriting) and the number of
writers (400 writers). Despite their weakness,
features combination can improve the results by
using k-NN (66.68%) as well as SVM (66.62%).
Table 4: Performance rates using handwritten dataset.
k-NN Classifier
(Canberra distance and k=1)
ZM TMM IM FMT FD AMI
Rate
45.65
34.13 32.75 26.34 22.55 22.20
Features Combination
All
ZM+TMM
+IM+AMI+
FMT
ZM+TMM
+IM+FMT
ZM+TMM
+IM+AMI
ZM+TM
M+IM+F
D
Rate
66.68
66.13 65.10 62.06 61.10
SVM Classifier
ZM FMT FD AMI IM TMM
L
55.24 33.44 17.03 14.27 13.1 3.44
P
(a)
60.34 26.62 20.55 11.17 9.10 3.44
(b)
60.62 30.96 17.79 11.86 6.41 3.44
(c)
57.72 37.37 3.44 14.82 3.44 3.44
S
(d)
50.89 3.86 2.96 7.37 3.51 3.65
(e)
54.20 3.31 3.44 8.27 3.70 3.65
(f)
51.93 3.44 3.79 7.58 3.03 3.51
R
B
F
(g)
60.68 37.17 18.62 16.20 7.86 4.68
(h)
60.89
38.13 23.03 14.13 7.72 4.68
(i)
60.34 30.48 22.89 12.68 8.06 4.75
Features Combination
ZM+FM
T
ZM+FMT+
FD
ZM+FMT+
FD+AMI
ZM+FD All
L 61.86 61.17 62.13 54.96 3.44
(h)
66.62
37.44 35.51 32.62 5.03
(b) 26.82 39.44 40.62 38.20 3.44
4 CONCLUSIONS AND FUTURE
WORK
The present paper proposed a comparative study
over Arabic optical character recognition, following
the statistic approach. We tried to highlight the
obtained results using different datasets, different
feature extraction techniques and different
classifiers. For printed and for handwritten datasets,
Zernike moments give the best recognition rate. This
conclusion can be explained by Zernike polar
coordinates which are more robust than other
coordinates types. For multi-oriented dataset, affine
moment invariants are in first position. This
conclusion can be explained by their robust
invariance to the rotation. The choice of k-NN or
SVM depends on the system needs. In future
experiments, we aim to extend our study to larger
datasets and to incorporate and to study other
different feature extraction techniques (wavelets,
fractal dimension ...) and different classifiers (neural
networks...). In future work, we will develop the
system towards Arabic words and texts recognition.
REFERENCES
Abandah, G. and Anssari, N., 2009. Novel moment
features extraction for recognizing handwritten arabic
letters. J. Comput. Sci., 5: 226-232. DOI: 10.3844/
jcssp. 2009. 226.232.
Abdul Sattar, S., Shah, S., 2012. Character Recognition of
Arabic Script Languages. ICCIT, pp. 502-506.
Aboaisha, Hosain, Xu, Zhijie and El-Feghi, Idris (2012)
An investigation on efficient feature extraction
approaches for Arabic letter recognition. In:
PQDJCEAR’ Conference 2012: CEARC’12, pp. 80-
85. ISBN 978-1-86218-106-9.
Arif, M., Brouard, T., Vincent, N., 2006. A new fuzzy k-
Nearest Neighbors rule. Pattern recognition.
Imran, K. P., Abdulbari A. A., Ramteke R. J., 2012.
Recognition of Offline Handwritten Isolated Urdu
Character. ACR ISSN: 0975-3273 & E-ISSN: 0975-
9085, Volume 4, Issue 1, pp.-117-121.
Jenabzadeh, M. R., Azmi, R., Pishgoo, B., Shirazi, S.S.,
2011. Two Methods for Recognition of Hand Written
Farsi Characters. IJIP, Vol. 5(4).
Mozaffari, S., Faez, K., Kanan, H., R., 2004. Feature
Comparison between Fractal Codes and Wavelet
Transform in Handwritten Alphanumeric Recognition
Using SVM Classifier. ICPR (2): 331-334.
Sabri A. Mahmoud, Ashraf S. Mahmoud, 2009. Arabic
Character Recognition Using Modified Fourier
Spectrum (MFS) vs. Fourier Descriptors. Cybernetics
and Systems 40(3): 189-210.
Slimane, F., Ingold, R., Kanoun, S., Alimi, M. A., and
Hennebert, J., 2009. A New Arabic Printed Text
Image Database and Evaluation Protocols. In proc. of
10th IEEE ICDAR 2009, Barcelona (Spain), July 26 -
29 2009 , pp. 946-950.
Zaghloul, R. I., AlRawashdeh, E., F., Bader, D. M. K.,
2011. Multilevel Classifier in Recognition of
Handwritten Arabic Characters. Journal of Computer
Science 7 (4): 512-518.
ICPRAM2013-InternationalConferenceonPatternRecognitionApplicationsandMethods
284