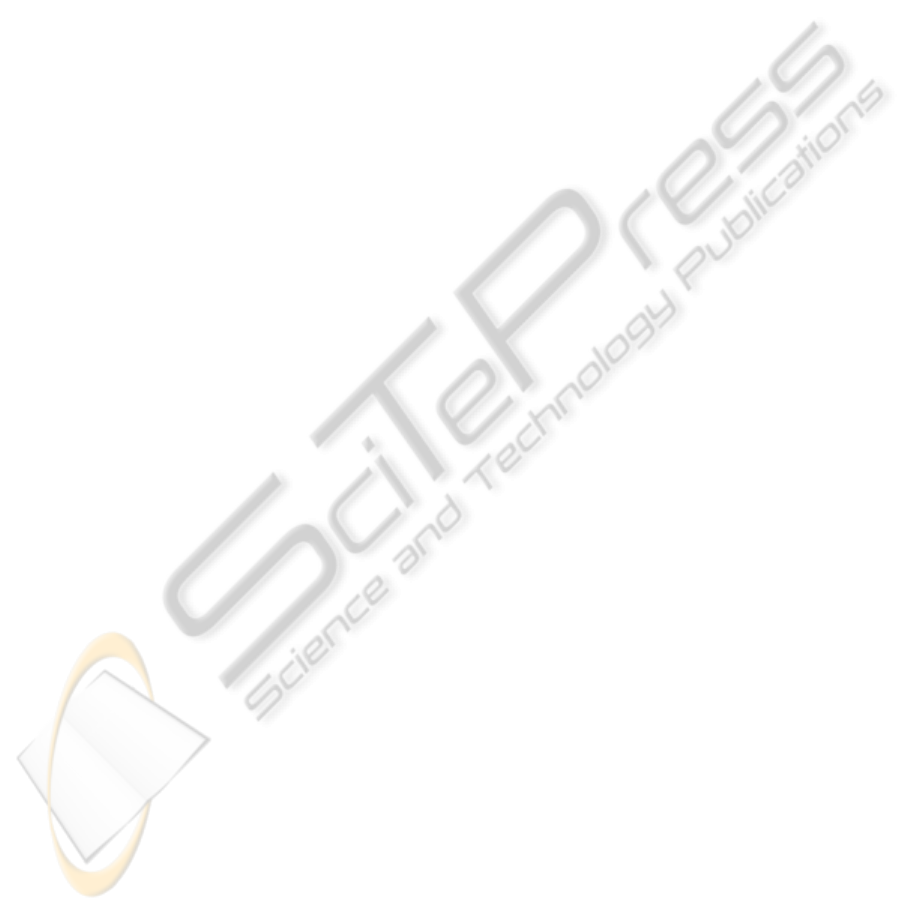
REFERENCES
Ali, S. and Smith, K. A. (2006). On learning algorithm
selection for classification. Applied Soft Computing,
6:119–138.
Asuncion, A. and Newman, D. (2007).
UCI machine learning repository.
http://www.ics.uci.edu/∼mlearn/MLRepository.html
University of California, Irvine, School of Informa-
tion and Computer Sciences.
Bennett, P. N., Dumais, S. T., and Horvitz, E. (2005). The
combination of text classifiers using reliability indica-
tors. Information Retrieval, 8(1):67–100.
Bensusan, H. and Giraud-Carrier, C. (2000a). Casa batl is
in passeig de grcia or how landmark performances can
describe tasks. In Proc. of the ECML-00 Workshop on
Meta-Learning: Building Automatic Advice Strategies
for Model Selection and Method Combination, pages
29–46.
Bensusan, H. and Giraud-Carrier, C. (2000b). Discovering
task neighbourhoods through landmark learning per-
formances. In Proc. of the 4th Europ. Conf. on Princi-
ples of Data Mining and Knowledge Discovery, pages
325–330.
Bensusan, H., Giraud-Carrier, C., and Kennedy, C. (2000).
A higher-order approach to meta-learning. In Proc. of
the ECML’2000 workshop on Meta-Learning: Build-
ing Automatic Advice Strategies for Model Selection
and Method Combination, pages 109–117.
Brazdil, P., Gama, J., and Henery, B. (1994). Characteriz-
ing the applicability of classification algorithms using
meta-level learning. In Machine Learning: ECML-94,
volume 784 of Lecture Notes in Computer Science,
pages 83–102. Springer Berlin / Heidelberg.
Brazdil, P. B., Soares, C., and da Costa, J. P. (2003). Rank-
ing learning algorithms: Using IBL and meta-learning
on accuracy and time results. Machine Learning,
50(3):251–277.
Castiello, C., Castellano, G., and Fanelli, A. M. (2005).
Meta-data: Characterization of input features for
meta-learning. In Modeling Decisions for Artificial
Intelligence, volume 3558, pages 295–304. Springer
Berlin / Heidelberg.
Cornelson, M., Grossmann, R. L., Karidi, G. R., and Shnid-
man, D. (2002). Survey of Text Mining: Cluster-
ing, Classification, and Retrieval, chapter Combining
Families of Information Retrieval Algorithms using
Meta-Learning, pages 159–169. Springer.
Dietterich, T. G. (2000). Ensemble methods in machine
learning. In Proc. of the First Int. Workshop on Multi-
ple Classifier Systems, pages 1–15.
Engels, R. and Theusinger, C. (1998). Using a data metric
for preprocessing advice for data mining applications.
In Proc. of the Europ. Conf. on Artificial Intelligence,
pages 430–434.
Gama, J. and Brazdil, P. (1995). Characterization of clas-
sification algorithms. In Progress in Artificial Intelli-
gence, volume 990 of Lecture Notes in Computer Sci-
ence, pages 189–200. Springer Berlin / Heidelberg.
Kitoogo, F. E. and Baryamureeba, V. (2007). Meta-
knowledge as an engine in classifier combination. In-
ternational Journal of Computing and ICT Research,
1(2):74–86.
Kohavi, R. and John, G. H. (1997). Wrappers for feature
subset selection. Artificial Intelligence – Special issue
on relevance, 97:273–324.
Kuncheva, L. I. and Whitaker, C. J. (2003). Measures
of diversity in classifier ensembles and their relation-
ship with the ensemble accuracy. Machine Learning,
51(2):181–207.
Michie, D., Spiegelhalter, D. J., and Taylor, C. C. (1994).
Machine Learning, Neural and Statistical Classifica-
tion. Ellis Horwood.
Peng, Y., Flach, P., Soares, C., and Brazdil, P. (2002). Im-
proved dataset characterisation for meta-learning. In
Discovery Science, volume 2534 of Lecture Notes in
Computer Science, pages 193–208. Springer Berlin /
Heidelberg.
Pfahringer, B., Bensusan, H., and Giraud-Carrier, C. (2000).
Meta-learning by landmarking various learning algo-
rithms. In Proc. of the 17th Int. Conf. on Machine
Learning, pages 743–750.
Reif, M. (2012). A comprehensive dataset for evaluating
approaches of various meta-learning tasks. In First
International Conference on Pattern Recognition and
Methods.
Reif, M., Shafait, F., Goldstein, M., Breuel, T., and
Dengel, A. (2012). Automatic classifier selection
for non-experts. Pattern Analysis and Applications.
10.1007/s10044-012-0280-z.
Segrera, S., Pinho, J., and Moreno, M. (2008). Information-
theoretic measures for meta-learning. In Hybrid Ar-
tificial Intelligence Systems, volume 5271 of Lecture
Notes in Computer Science, pages 458–465. Springer
Berlin / Heidelberg.
Simonoff, J. S. (2003). Analyzing Categor-
ical Data. Springer Texts in Statis-
tics. Springer Berlin / Heidelberg.
http://people.stern.nyu.edu/jsimonof/AnalCatData/.
Sohn, S. Y. (1999). Meta analysis of classification al-
gorithms for pattern recognition. IEEE Transac-
tions on Pattern Analysis and Machine Intelligence,
21(11):1137 –1144.
Todorovski, L. and D
ˇ
zeroski, S. (2003). Combining clas-
sifiers with meta decision trees. Machine Learning,
50:223–249.
Vilalta, R., Giraud-Carrier, C., Brazdil, P., and Soares, C.
(2004). Using meta-learning to support data mining.
International Journal of Computer Science and Appli-
cations, 1(1):31–45.
Vlachos, P. (1998). StatLib datasets archive.
http://lib.stat.cmu.edu Department of Statistics,
Carnegie Mellon University.
Wolpert, D. H. (1996). The lack of a priori distinctions
between learning algorithms. Neural Computing,
8(7):1341–1390.
PredictingClassifierCombinations
297