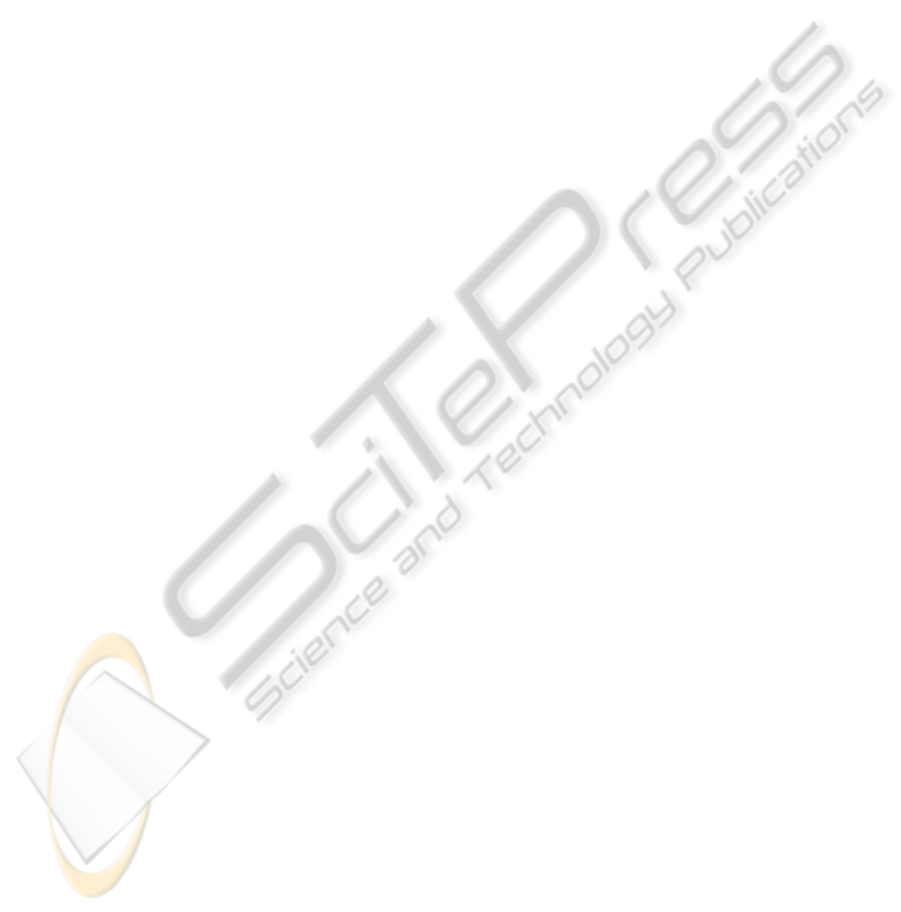
tration level: it is intuitive that lines which took longer
to finish did so because the subject was maintaining a
lower concentration level. A third possibility is to use
lines of different difficulty (with harder lines having
more pairs of numbers to be indicated), and assume
that harder lines will induce more stress. Acquisition
of data with this type of external information, and its
subsequent analysis using the methodology proposed
here, will be the subject of future work.
5 CONCLUSIONS
We presented a methodology for exploratory data
analysis of EEG data acquired while subjects per-
formed a task which demands high concentration lev-
els. We preprocessed the data using bandpass filter-
ing, independent component analysis (ICA) and em-
pirical mode decomposition (EMD); we then used
two different measures: band power features (BPF)
and phase-locking factor (PLF), which measure en-
ergy in typical EEG bands and phase synchrony
across pairs of channels, respectively. Finally, we
used the clustering ensembles framework to extract
relevant information from those features.
The main conclusion is the finding of few clus-
ters per subject and per test line (usually 2 or 3; 4 or
more clusters are rare), and the fact that these clus-
ters are almost always composed of a single time in-
terval. These findings suggest that this methodology
may be detecting a transition in the brain activity of
the subject, which could be caused by a change in the
emotional state due to tiredness or stress.
ACKNOWLEDGEMENTS
This work was supported by the Portuguese Founda-
tion for Science and Technology grants PTDC/EIA-
CCO/103230/2008 and SFRH/BD/65248/2009.
REFERENCES
Almeida, M., Schleimer, J.-H., Vig
´
ario, R., and Bioucas-
Dias, J. (2011). Source separation and clustering of
phase-locked subspaces. IEEE Transactions on Neu-
ral Networks, 22:1419–1434.
Ayad, H. G. and Kamel, M. S. (2005). Cluster-based cumu-
lative ensembles. In Proc. Int. Workshop on Multiple
Classifier Systems.
Carreiras, C., de Almeida, L. B., and Sanches, J. M.
(2012). Phase-locking factor in a motor imagery
brain-computer interface. In Eng. in Medicine and
Biology Society, 2012. 34th Annual Int. Conf. of the
IEEE.
Fred, A. (2001). Finding consistent clusters in data parti-
tions. In Proc. Int. Workshop on Multiple Classifier
Systems, pages 309–318.
Fred, A. and Jain, A. (2005). Combining multiple clus-
terings using evidence accumulation. IEEE Transac-
tions on Pattern Analysis and Machine Intelligence,
27(6):835–850.
Fred, A. and Jain, A.K. (2002). Evidence Accumulation
Clustering based on the K-Means Algorithm. In Proc.
Joint IAPR Int. Workshop on Structural, Syntactic and
Statistical Pattern Recognition, pages 442–451.
Fulton, J. (2000). The Mensa Book of Total Genius. Carlton
Books.
Gamboa, H., Silva, H., and Fred, A. (2007). HiMotion
project. Technical report, Instituto Superior T
´
ecnico,
Lisbon, Portugal.
Huang, N., Shen, Z., Long, S., Wu, M., Shih, H., Zheng,
Q., Yen, N., Tung, C., and Liu, H. (1998). The em-
pirical mode decomposition and the hilbert spectrum
for nonlinear and non-stationary time series analysis.
Proc. Royal Society of London. Series A: Mathemati-
cal, Physical and Eng. Sciences, 454(1998):903–995.
Hyv
¨
arinen, A., Karhunen, J., and Oja, E. (2001). In-
dependent component analysis, volume 26. Wiley-
interscience.
Jain, A. K., Murty, M. N., and Flynn, P. J. (1999). Data
clustering: a review. ACM Computing Surveys,
31(3):264–323.
Jung, T., Makeig, S., Westerfield, M., Townsend, J.,
Courchesne, E., and Sejnowski, T. (2000). Removal
of eye activity artifacts from visual event-related po-
tentials in normal and clinical subjects. Clinical Neu-
rophysiology, 111(10):1745–1758.
Kuncheva, L. I. and Hadjitodorov, S. T. (2004). Using di-
versity in cluster ensembles. In Proc. Int. Conf. on
Systems, Man and Cybernetics, pages 1214–1219.
Pfurtscheller, G. and Lopes da Silva, F. H. (1999). Event-
related EEG/MEG synchronization and desynchro-
nization: basic principles. Clinical Neurophysiology,
110:1842 – 1857.
Strehl, A. and Ghosh, J. (2002). Cluster ensembles - a
knowledge reuse framework for combining multiple
partitions. Journal of Machine Learning Research,
3:583–617.
Theodoridis, S. and Koutroumbas, K. (2009). Pattern
Recognition. Elsevier Academic Press, 4th edition.
Vega-Pons, S. and Ruiz-Shulcloper, J. (2011). A survey of
clustering ensemble algorithms. Int. Journal of Patt.
Recog. and Artificial Intelligence, 25(3):337–372.
ICPRAM2013-InternationalConferenceonPatternRecognitionApplicationsandMethods
484