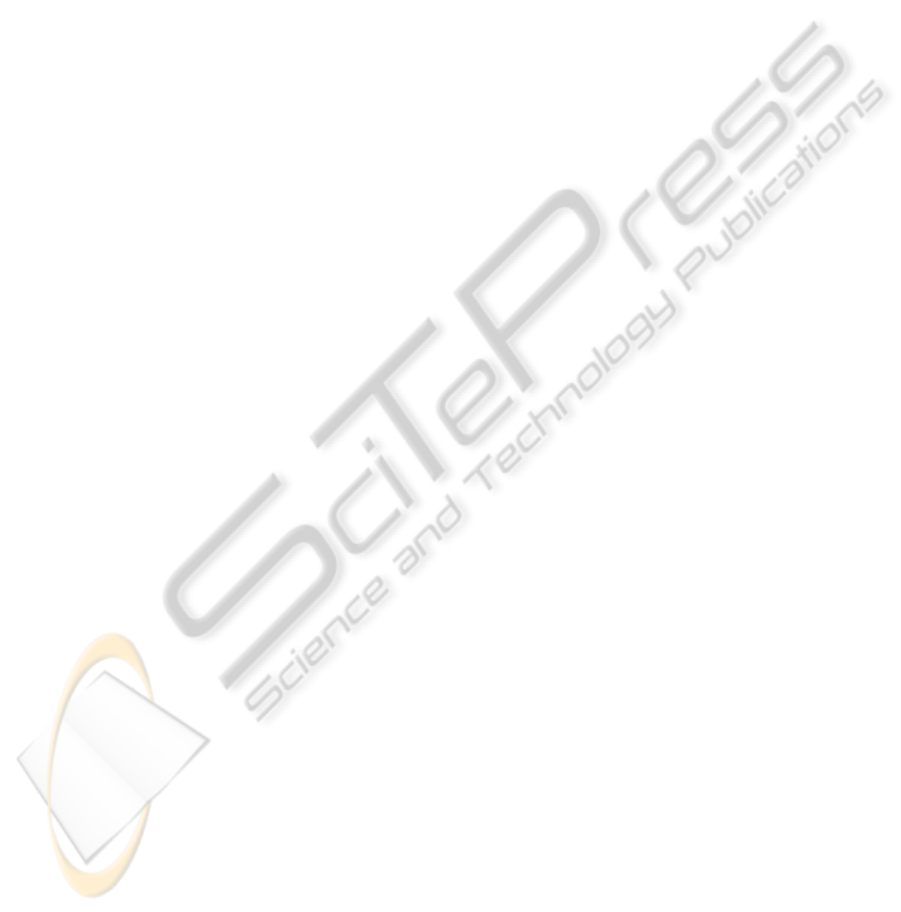
with different types of data. Under a supervised
learning evaluationtask with different classifiers, both
techniques have shown improvement as compared to
unsupervised and supervised FD approaches. The
first technique has obtained similar or better results,
when compared to its unsupervised counterparts. For
the supervised FD tests, the second technique has
proved to be more effective regarding the number of
discretization intervals and the generalization error.
For both techniques, the classifiers learned on dis-
crete features usually attain better accuracy than those
learned on the original ones. Both techniques scale
well for high-dimensional and multi-class problems.
As future work, we will explore the embedding of
feature selection in the discretization process.
REFERENCES
Battiti, R. (1994). Using mutual information for select-
ing features in supervised neural net learning. IEEE
Transactions on Neural Networks, 5:537–550.
Brown, G., Pocock, A., Zhao, M., and Luj´an, M. (2012).
Conditional likelihood maximisation: A unifying
framework for information theoretic feature selection.
J. Machine Learning Research, 13:27–66.
Chiu, D., Wong, A., and Cheung, B. (1991). Information
discovery through hierarchical maximum entropy dis-
cretization and synthesis. In Proc. of the Knowledge
Discovery in Databases, pages 125–140.
Cover, T. and Thomas, J. (1991). Elements of Information
Theory. John Wiley & Sons.
Demsar, J. (2006). Statistical comparisons of classifiers
over multiple data sets. Journal of Machine Learning
Research, 7:1–30.
Dougherty, J., Kohavi, R., and Sahami, M. (1995). Su-
pervised and unsupervised discretization of continu-
ous features. In Int. Conf. Mac. Learn. (ICML), pages
194–202.
Duin, R., Juszczak, P., Paclik, P., Pekalska, E., Ridder, D.,
Tax, D., and Verzakov, S. (2007). PRTools4.1: A Mat-
lab Toolbox for Pattern Recognition. Technical report,
Delft Univ. Technology.
Fayyad, U. and Irani, K. (1993). Multi-interval discretiza-
tion of continuous-valued attributes for classification
learning. In Proc. Int. Joint Conf. on Art. Intell. (IJ-
CAI), pages 1022–1027.
Ferreira, A. and Figueiredo, M. (2012). An unsupervised
approach to feature discretization and selection. Pat-
tern Recognition, 45:3048–3060.
Frank, A. and Asuncion, A. (2010). UCI machine learning
repository, available at http://archive.ics.uci.edu/ml.
Friedman, M. (1940). A comparison of alternative tests of
significance for the problem of m rankings. The An-
nals of Mathematical Statistics, 11(1):86–92.
Garcia, S. and Herrera, F. (2008). An extension on ”sta-
tistical comparisons of classifiers over multiple data
sets” for all pairwise comparisons. Journal of Ma-
chine Learning Research, 9:2677–2694.
Hellman, M. (1970). Probability of error, equivocation, and
the Chernoff bound. IEEE Transactions on Informa-
tion Theory, 16(4):368–372.
Jin, R., Breitbart, Y., and Muoh, C. (2009). Data discretiza-
tion unification. Know. Inf. Systems, 19(1):1–29.
Kononenko, I. (1995). On biases in estimating multi-valued
attributes. In Proc. Int. Joint Conf. on Art. Intell. (IJ-
CAI), pages 1034–1040.
Kotsiantis, S. and Kanellopoulos, D. (2006). Discretization
techniques: A recent survey. GESTS Int. Trans. on
Computer Science and Engineering, 32(1).
Kurgan, L. and Cios, K. (2004). CAIM discretization algo-
rithm. IEEE Trans. on Know. and Data Engineering,
16(2):145–153.
Linde, Y., Buzo, A., and Gray, R. (1980). An algorithm for
vector quantizer design. IEEE Trans. on Communica-
tions, 28:84–94.
Liu, H., Hussain, F., Tan, C., and Dash, M. (2002). Dis-
cretization: An Enabling Technique. Data Mining and
Knowledge Discovery, 6(4):393–423.
Santhi, N. and Vardy, A. (2006). On an improvement over
R´enyi’s equivocation bound. In 44-th Annual Allerton
Conference on Communication, Control, and Com-
puting.
Statnikov, A., Tsamardinos, I., Dosbayev, Y., and Aliferis,
C. F. (2005). GEMS: a system for automated cancer
diagnosis and biomarker discovery from microarray
gene expression data. Int J Med Inf., 74(7-8):491–503.
Tsai, C.-J., Lee, C.-I., and Yang, W.-P. (2008). A dis-
cretization algorithm based on class-attribute contin-
gency coefficient. Inf. Sci., 178:714–731.
Witten, I. and Frank, E. (2005). Data Mining: Practical
Machine Learning Tools and Techniques. Elsevier,
Morgan Kauffmann.
Yang, Y. and Webb, G. (2001). Proportional k-interval dis-
cretization for na¨ıve-Bayes classifiers. In 12th Eur.
Conf. on Machine Learning, (ECML), pages 564–575.
Zhao, Z., Morstatter, F., Sharma, S., Alelyani, S., Anand,
A., and Liu, H. (2010). Advancing feature selection
research - ASU feature selection repository. Technical
report, Arizona State University.
RelevanceandMutualInformation-basedFeatureDiscretization
77