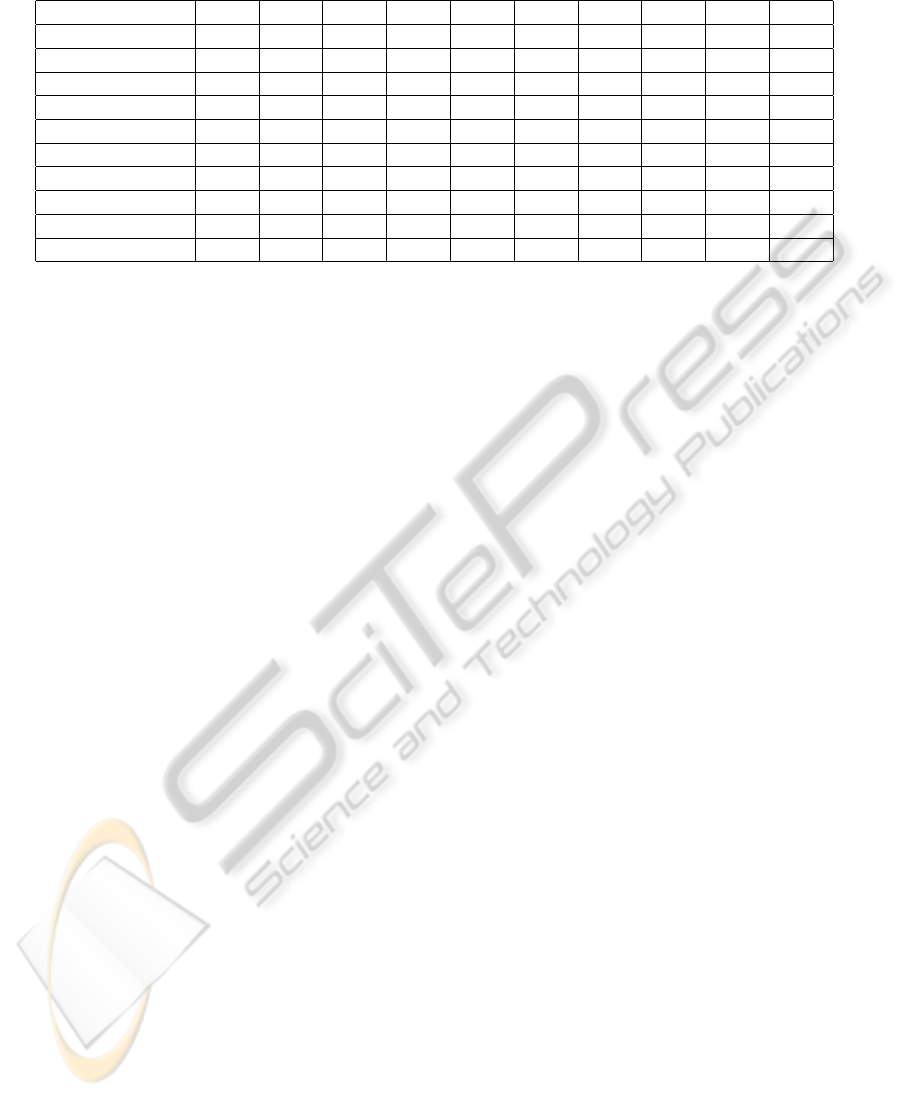
Table 1: Confusion Matrix for the MNIST Data Segmentation.
Obtained / True 0 1 2 3 4 5 6 7 8 9
0 6712 3 39 10 6 36 57 10 61 28
1 1 7738 7 15 9 1 9 23 36 12
2 24 50 6632 95 65 17 16 63 65 30
3 13 16 84 6585 8 218 5 42 153 84
4 5 6 27 8 6279 32 13 59 43 305
5 21 6 13 128 27 5736 57 3 262 34
6 91 26 50 11 35 91 6693 0 45 1
7 6 6 31 97 26 15 0 6689 24 331
8 27 15 86 156 21 110 25 16 6065 66
9 3 11 21 36 348 57 1 388 71 6067
0569 and by ONR grant N0001411AF00002.
REFERENCES
Allwein, E. L., Schapire, R. E., and Singer, Y. (2000). Re-
ducing multiclass to binary: A unifying approach for
margin classifiers. Journal of Machine Learning Re-
search, 1:113–141.
Bertozzi, A., Esedo
¯
glu, S., and Gillette, A. (2007). Inpaint-
ing of binary images using the Cahn-Hilliard equation.
IEEE Transactions on Image Processing, 16(1):285–
291.
Bertozzi, A. L. and Flenner, A. (2012). Diffuse inter-
face models on graphs for classification of high di-
mensional data. Multiscale Modeling and Simulation,
10(3):1090–1118.
B
¨
uhler, T. and Hein, M. (2009). Spectral clustering based on
the graph p-Laplacian. In Bottou, L. and Littman, M.,
editors, Proceedings of the 26th International Confer-
ence on Machine Learning, pages 81–88. Omnipress,
Montreal, Canada.
Chung, F. R. K. (1997). Spectral graph theory. In Regional
Conference Series in Mathematics, volume 92. Con-
ference Board of the Mathematical Sciences (CBMS),
Washington, DC.
Coifman, R. R., Lafon, S., Lee, A. B., Maggioni, M.,
Nadler, B., Warner, F., and Zucker, S. W. (2005).
Geometric diffusions as a tool for harmonic analy-
sis and structure definition of data: Diffusion maps.
Proceedings of the National Academy of Sciences,
102(21):7426–7431.
Dietterich, T. G. and Bakiri, G. (1995). Solving multiclass
learning problems via error-correcting output codes.
Journal of Artificial Intelligence Research, 2(1):263–
286.
Dobrosotskaya, J. A. and Bertozzi, A. L. (2008). A wavelet-
laplace variational technique for image deconvolu-
tion and inpainting. IEEE Trans. Image Process.,
17(5):657–663.
Dobrosotskaya, J. A. and Bertozzi, A. L. (2010). Wavelet
analogue of the Ginzburg-Landau energy and its
gamma-convergence. Interfaces and Free Boundaries,
12(2):497–525.
Gilboa, G. and Osher, S. (2008). Nonlocal operators with
applications to image processing. Multiscale Model-
ing and Simulation, 7(3):1005–1028.
Har-Peled, S., Roth, D., and Zimak, D. (2003). Constraint
classification for multiclass classification and ranking.
In S. Becker, S. T. and Obermayer, K., editors, Ad-
vances in Neural Information Processing Systems 15,
pages 785–792. MIT Press, Cambridge, MA.
Hastie, T. and Tibshirani, R. (1998). Classification by pair-
wise coupling. In Advances in Neural Information
Processing Systems 10. MIT Press, Cambridge, MA.
Hein, M. and Setzer, S. (2011). Beyond spectral clus-
tering - tight relaxations of balanced graph cuts. In
Shawe-Taylor, J., Zemel, R., Bartlett, P., Pereira, F.,
and Weinberger, K., editors, Advances in Neural In-
formation Processing Systems 24, pages 2366–2374.
Jung, Y. M., Kang, S. H., and Shen, J. (2007). Multiphase
image segmentation via Modica-Mortola phase transi-
tion. SIAM J. Appl. Math, 67(5):1213–1232.
Kohn, R. V. and Sternberg, P. (1989). Local minimizers
and singular perturbations. Proc. Roy. Soc. Edinburgh
Sect. A, 111(1-2):69–84.
Li, Y. and Kim, J. (2011). Multiphase image segmentation
using a phase-field model. Computers and Mathemat-
ics with Applications, 62:737–745.
Liu, W., He, J., and Chang, S.-F. (2010). Large graph con-
struction for scalable semi-supervised learning. Pro-
ceedings of the 27th International Conference on Ma-
chine Learning.
Subramanya, A. and Bilmes, J. (2011). Semi-supervised
learning with measure propagation. Journal of Ma-
chine Learning Research, 12:3311–3370.
Szlam, A. and Bresson, X. (2010). Total variation and
cheeger cuts. In F
¨
urnkranz, J. and Joachims, T., edi-
tors, Proceedings of the 27th International Conference
on Machine Learning, pages 1039–1046. Omnipress,
Haifa, Israel.
Szlam, A. D., Maggioni, M., and Coifman, R. R. (2008).
Regularization on graphs with function-adapted dif-
fusion processes. Journal of Machine Learning Re-
search, 9:1711–1739.
von Luxburg, U. (2006). A tutorial on spectral clustering.
Technical Report TR-149, Max Planck Institute for
Biological Cybernetics.
MulticlassDiffuseInterfaceModelsforSemi-supervisedLearningonGraphs
85